Leto: Crowdsourced Radio Map Construction With Learned Topology and a Few Landmarks.
IEEE Trans. Mob. Comput.(2024)
摘要
Existing crowdsourced indoor positioning systems (CIPSs) usually require prior knowledge about the site and a tedious calibration process. Moreover, they may require a large number of landmarks while ignoring the topology information that may be contained in the crowdsourced data. In this paper, we present Leto, a system that uses le arned to pology information from combined user traces to construct a radio map. Leto relies on crowdsourced WiFi and accelerometer signals only without requiring any prior knowledge about the site. Our key idea is that learned topology information can reduce the required number of landmarks, while available landmarks can transform the topology into a map. We propose a novel framework that efficiently learns the map topology by a hybrid multidimensional scaling (HMDS) algorithm and accurately rectifies the map using only a few anchors by an adaptive force-directed (AFD) algorithm. We also provide a theoretical convergence analysis of the HMDS algorithm. Experimental results on real-world datasets show that Leto can capture useful topology information and achieve significant improvements in radio map construction compared to existing systems.
更多查看译文
关键词
Map rectification,radio map construction,topology learning
AI 理解论文
溯源树
样例
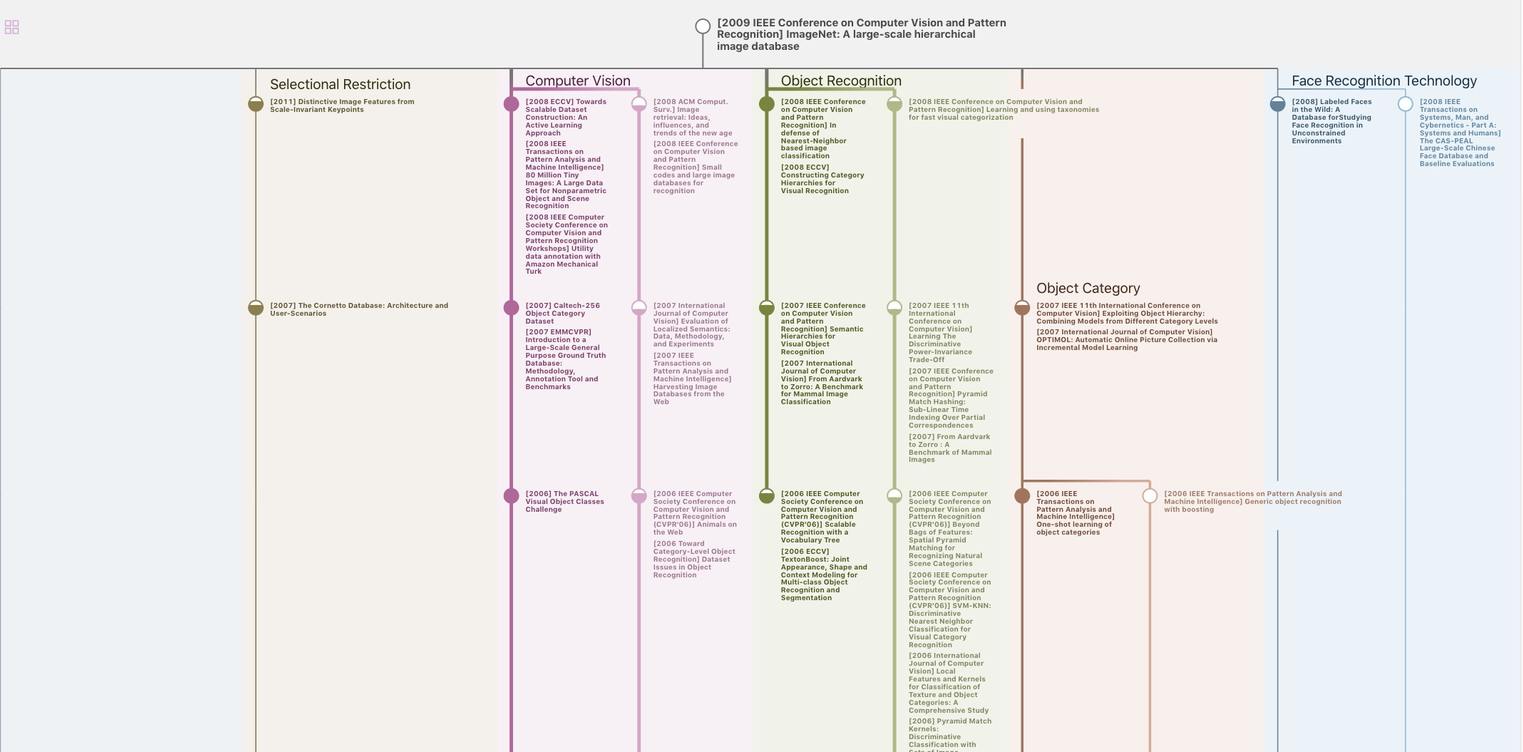
生成溯源树,研究论文发展脉络
Chat Paper
正在生成论文摘要