NeuSort: An Automatic Adaptive Spike Sorting Approach with Neuromorphic Models
arXiv (Cornell University)(2023)
摘要
Objective. Spike sorting, a critical step in neural data processing, aims to classify spiking events from single electrode recordings based on different waveforms. This study aims to develop a novel online spike sorter, NeuSort, using neuromorphic models, with the ability to adaptively adjust to changes in neural signals, including waveform deformations and the appearance of new neurons. Approach. NeuSort leverages a neuromorphic model to emulate template-matching processes. This model incorporates plasticity learning mechanisms inspired by biological neural systems, facilitating real-time adjustments to online parameters. Results. Experimental findings demonstrate NeuSort's ability to track neuron activities amidst waveform deformations and identify new neurons in real-time. NeuSort excels in handling non-stationary neural signals, significantly enhancing its applicability for long-term spike sorting tasks. Moreover, its implementation on neuromorphic chips guarantees ultra-low energy consumption during computation. Significance. NeuSort caters to the demand for real-time spike sorting in brain-machine interfaces through a neuromorphic approach. Its unsupervised, automated spike sorting process makes it a plug-and-play solution for online spike sorting.
更多查看译文
关键词
adaptive
AI 理解论文
溯源树
样例
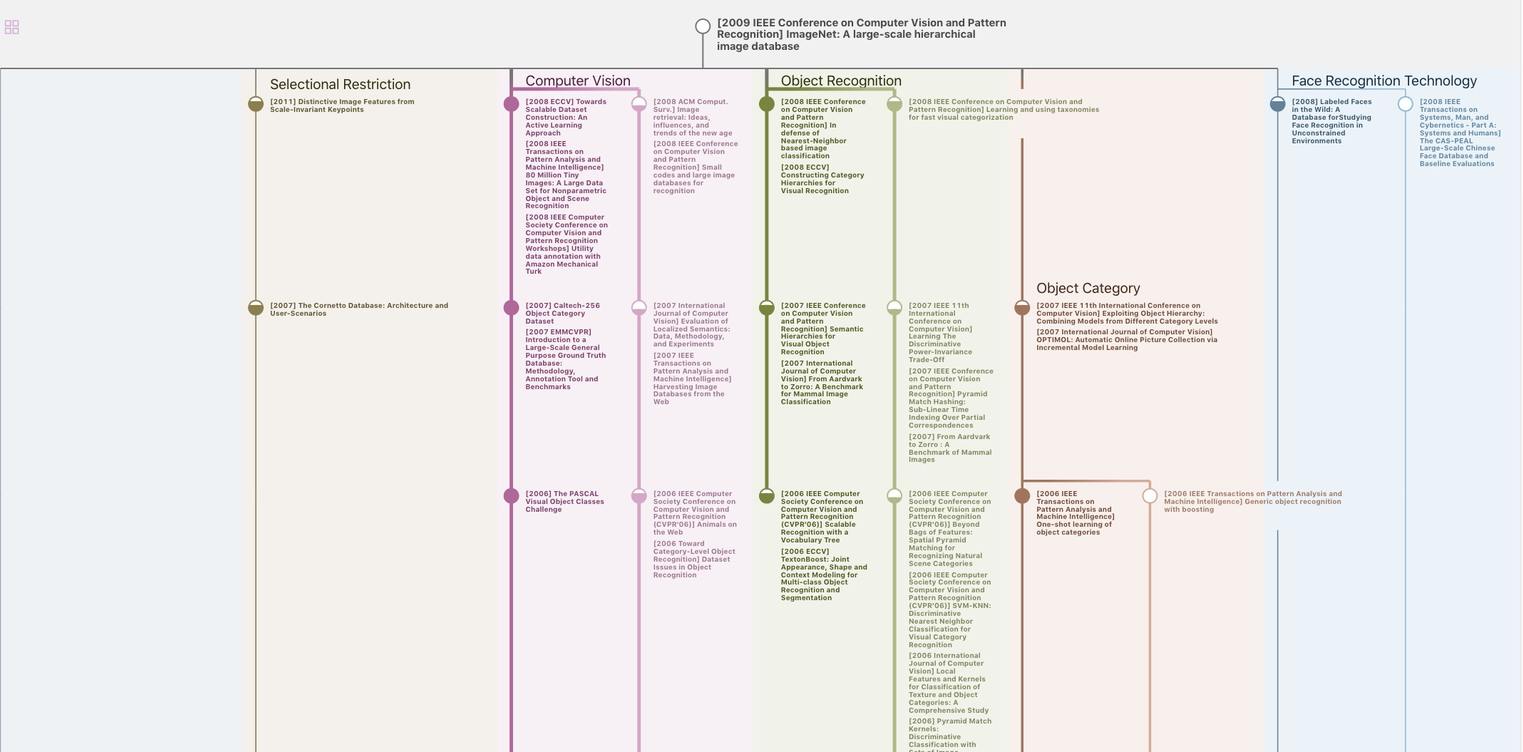
生成溯源树,研究论文发展脉络
Chat Paper
正在生成论文摘要