Modeling airfoil dynamic stall using State-Space Neural Networks
AIAA SCITECH 2023 Forum(2023)
摘要
The present paper investigates the effectiveness of artificial neural networks for the identification of nonlinear state-space models in fluid dynamics. The test case under consideration consists in the modeling of the unsteady lift force of a pitching NACA 0018 airfoil at a Reynolds number Re = 3 x 10^5. Data used for model training and validation are obtained through Computational Fluid Dynamics (CFD) simulations performed in OpenFOAM. The State-Space Neural Network (SS-NN) model is trained using swept sines performed at several angle-of-attack ranges, and it is then validated using different sine sweeps as well as simple harmonic motions. The study demonstrates that the selected SS-NN model represents a powerful tool to accurately predict the unsteady aerodynamic loads of a pitching airfoil, both in pre-stall and post-stall conditions. In particular, the model succeeds in correctly capturing highly nonlinear flow features such as the delay in flow separation, and the formation and shedding of the dynamic stall vortex. The accuracy and evaluation speed of this technique make it particularly valuable for engineering applications involving design optimization and real-time control of systems based on lift. The obtained results represent a stepping-stone towards the integration of flexible, data-driven SS-NN models representing unsteady aerodynamics inside dynamic multi-physics models.
更多查看译文
关键词
airfoil,dynamic stall,neural networks,state-space
AI 理解论文
溯源树
样例
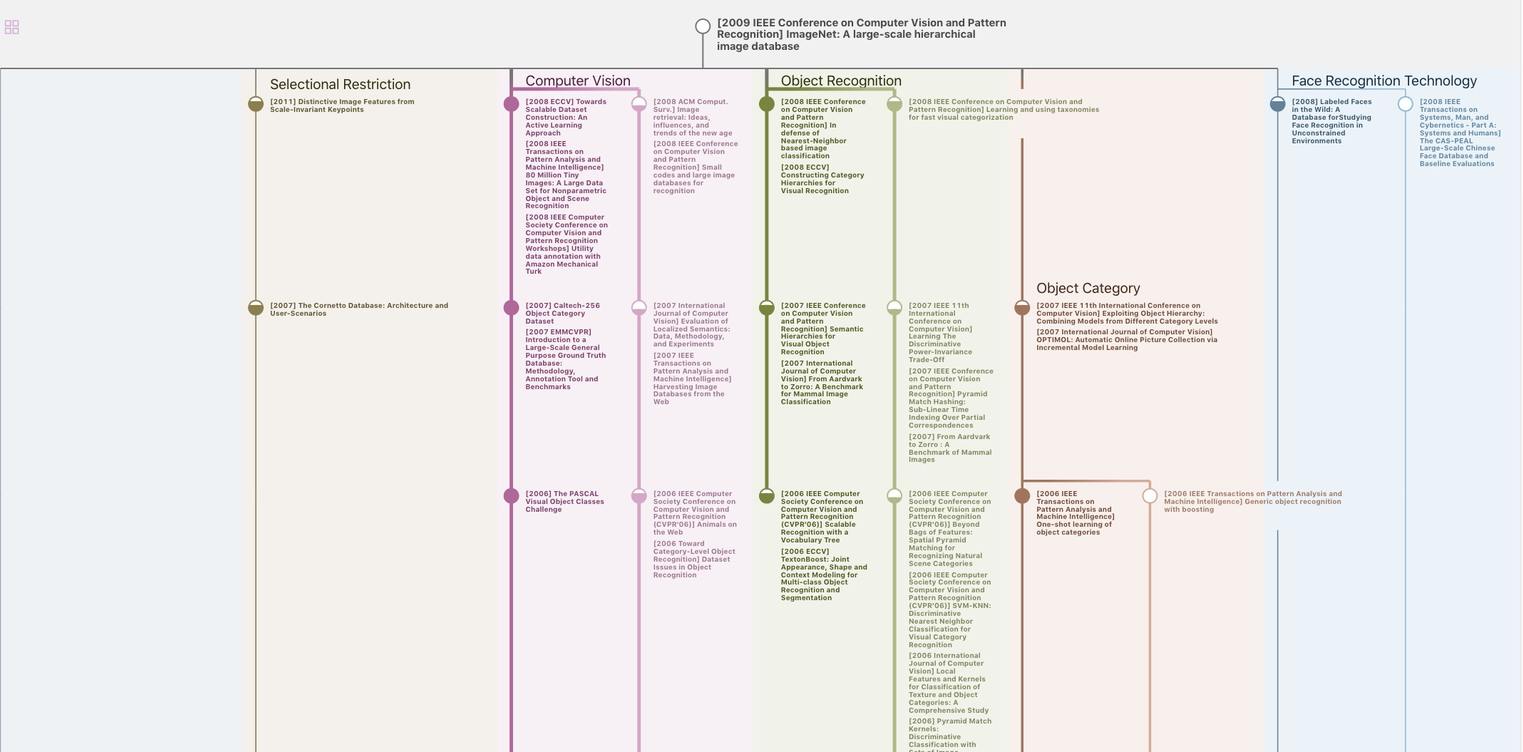
生成溯源树,研究论文发展脉络
Chat Paper
正在生成论文摘要