Entropy-stable Deep Learning for Navier–Stokes Predictions of Transitional-regime Flows
AIAA SCITECH 2023 Forum(2023)
摘要
The simulation of rarefied and/or nonequilibrium flows generally requires the computation of expensive and approximate solutions to the Boltzmann equations. Since these regimes are at the limit of the continuum assumption, the accuracy of the (computationally tractable) Navier–Stokes equations is unreliable. To be able to reliably use the Navier–Stokes equations in these regimes, a deep learning augmentation framework was recently presented [1] with optimization targets obtained from Boltzmann equation solutions. This framework is different from standard applications of deep learning methods to physical systems. It leverages the a-priori-known physics and ensures consistency with the governing equations. The training of the deep neural network is done via solving adjoint equations which calculate the the loss sensitivities with respect to the dependent variables. The adjoint PDEs are constructed using automatic differentiation (AD). To enable more powerful models, we propose entropy constraints on the closure model outputs to ensure consistency with the second law of thermodynamics. An example prediction of density profiles for low-pressure argon is presented, for which the augmented models have comparable accuracy to reference direct Boltzmann solutions. Comparisons of different augmentation methods are also presented.
更多查看译文
关键词
navier–stokes predictions,learning,entropy-stable,transitional-regime
AI 理解论文
溯源树
样例
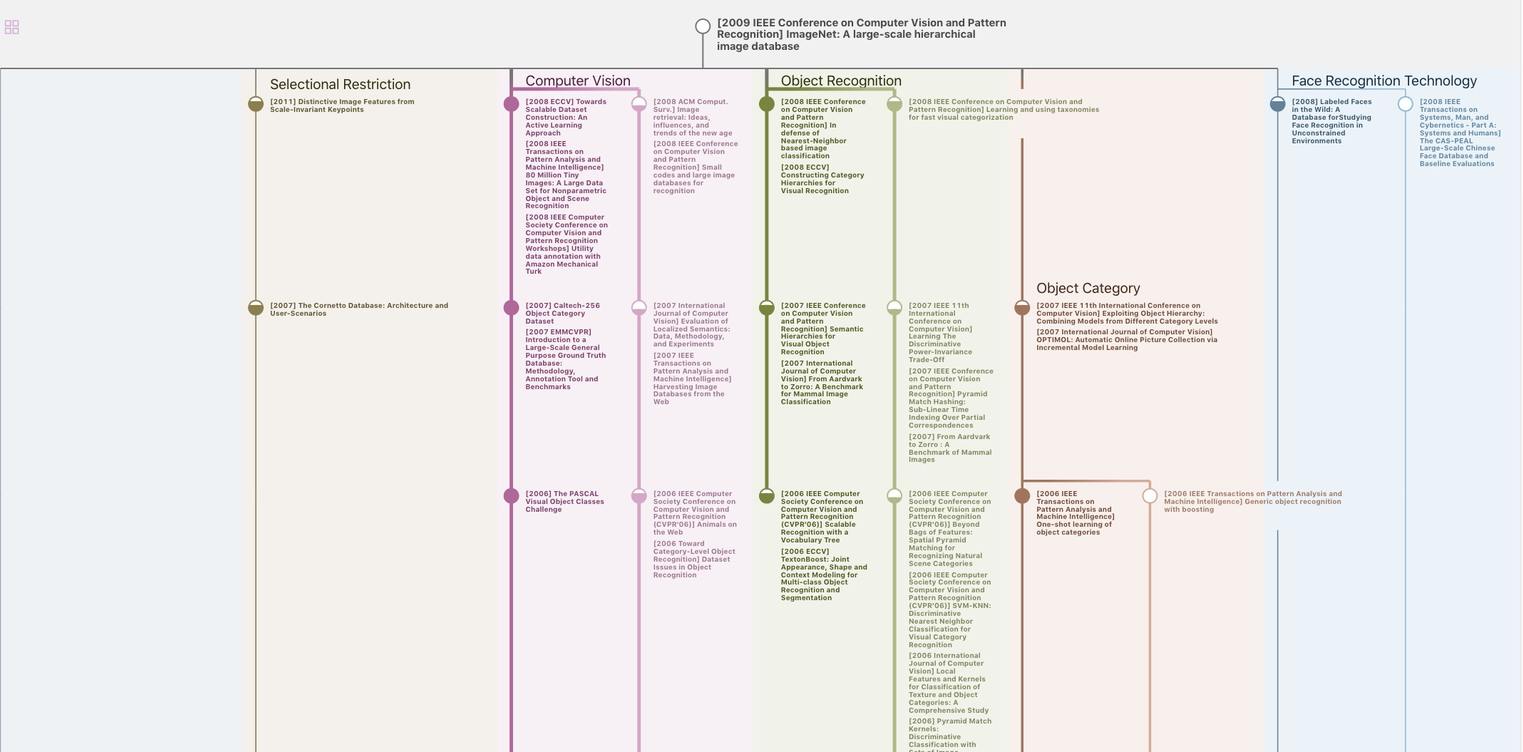
生成溯源树,研究论文发展脉络
Chat Paper
正在生成论文摘要