Robot Unknown Objects Instance Segmentation Based on Collaborative Weight Assignment RGB-Depth Fusion Strategy
IEEE-ASME TRANSACTIONS ON MECHATRONICS(2023)
摘要
Unknown objects instance-aware segmentation (UOIS) is crucial for the operation of autonomous robots, especially in unstructured scenes with unknown objects. As the primary data source types for robots, RGB and Depth are not fully exploited by existing studies due to the inherent information differences between RGB (2-D appearance) and Depth (3-D geometry). Therefore, it is challenging to fully exploit the features of both modalities to achieve segmentation for instances of unknown objects. This article proposes a collaborative weight assignment (CWA) fusion strategy for fusing RGB and Depth (RGB-D). It contains three carefully designed modules, motivational pixel weight assignment(MPWA) module, dual-direction spatial weight assignment (DSWA) module, and stepwise global feature aggregation (SGFA) module. Our method aims to adaptively assign fusion weights between two modalities to exploit RGB-D features from multiple dimensions better. On the popular Graspnet-1Bilion and WISDOM RGB-D robot operation datasets, the proposed method achieves competitive performance with state-of-the-art techniques, proving our approach can make good use of the information between the two modalities. Furthermore, we have deployed the fusion model on the AUBO i5 robotic manipulation platform to test its segmentation and grasping optimization effects oriented to unknown objects. The proposed method achieves robust performance through qualitative and quantitative analysis experiments.
更多查看译文
关键词
Instance-aware segmentation,RGB-D fusion,robotic operation,unknown objects,weight shared strategy
AI 理解论文
溯源树
样例
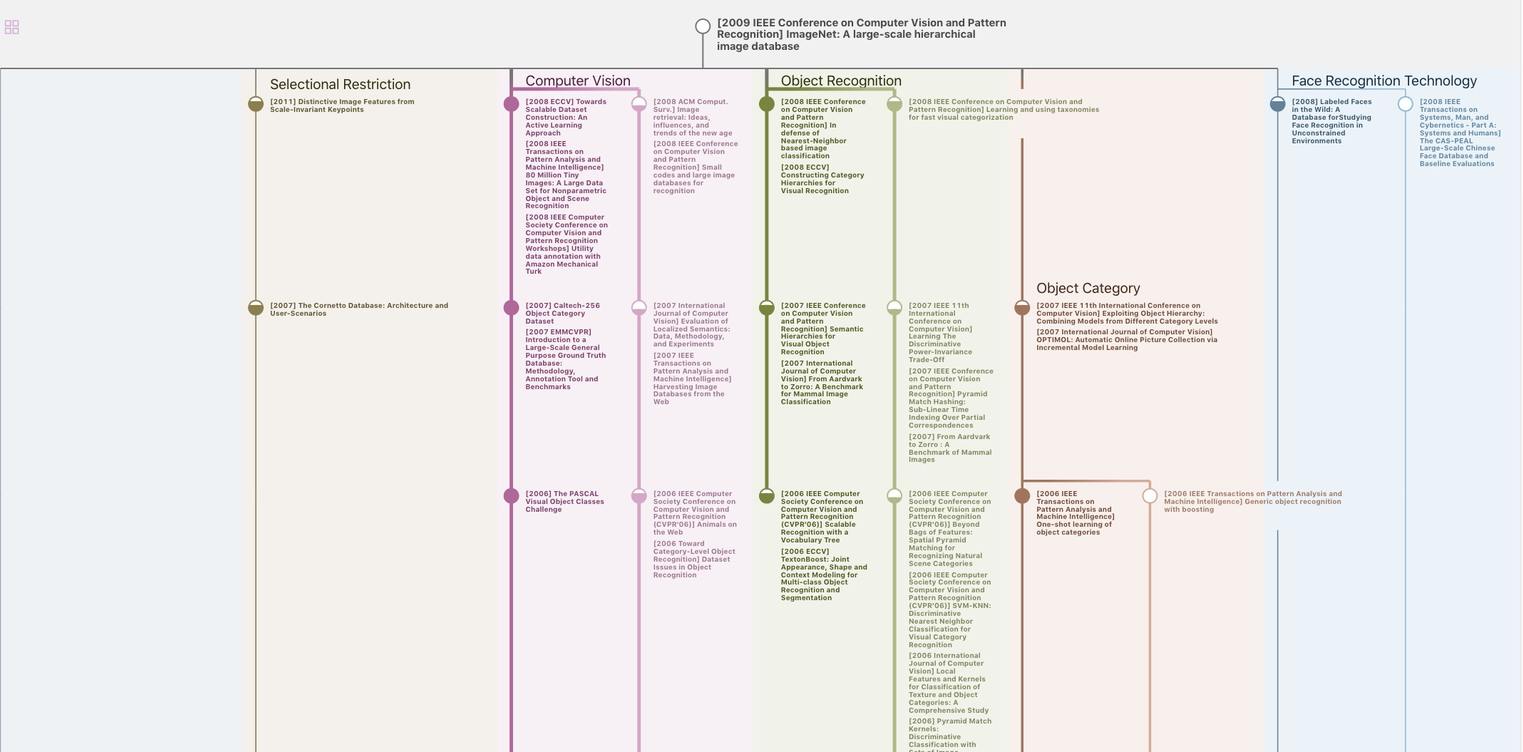
生成溯源树,研究论文发展脉络
Chat Paper
正在生成论文摘要