Distributed Reinforcement Learning for Networked Dynamical Systems
IEEE Transactions on Control of Network Systems(2023)
摘要
We propose a scalable algorithm for learning distributed optimal controllers for networked dynamical systems. Assuming that the network is comprised of nearly homogeneous subsystems, each sub-controller is trained by the local state and input information from its corresponding subsystem and filtered information from its neighbors. Thus, the costs of both learning and control become independent of the number of subsystems. We show the optimality and convergence of the algorithm for the case when the individual subsystems are identical, based on an algebraic property of such networks. Thereafter, we show the robustness of the algorithm when applied to general heterogeneous networks. The effectiveness of the design is investigated through numerical simulations.
更多查看译文
关键词
Distributed control,distributed reinforcement learning,networked dynamical systems,scalability
AI 理解论文
溯源树
样例
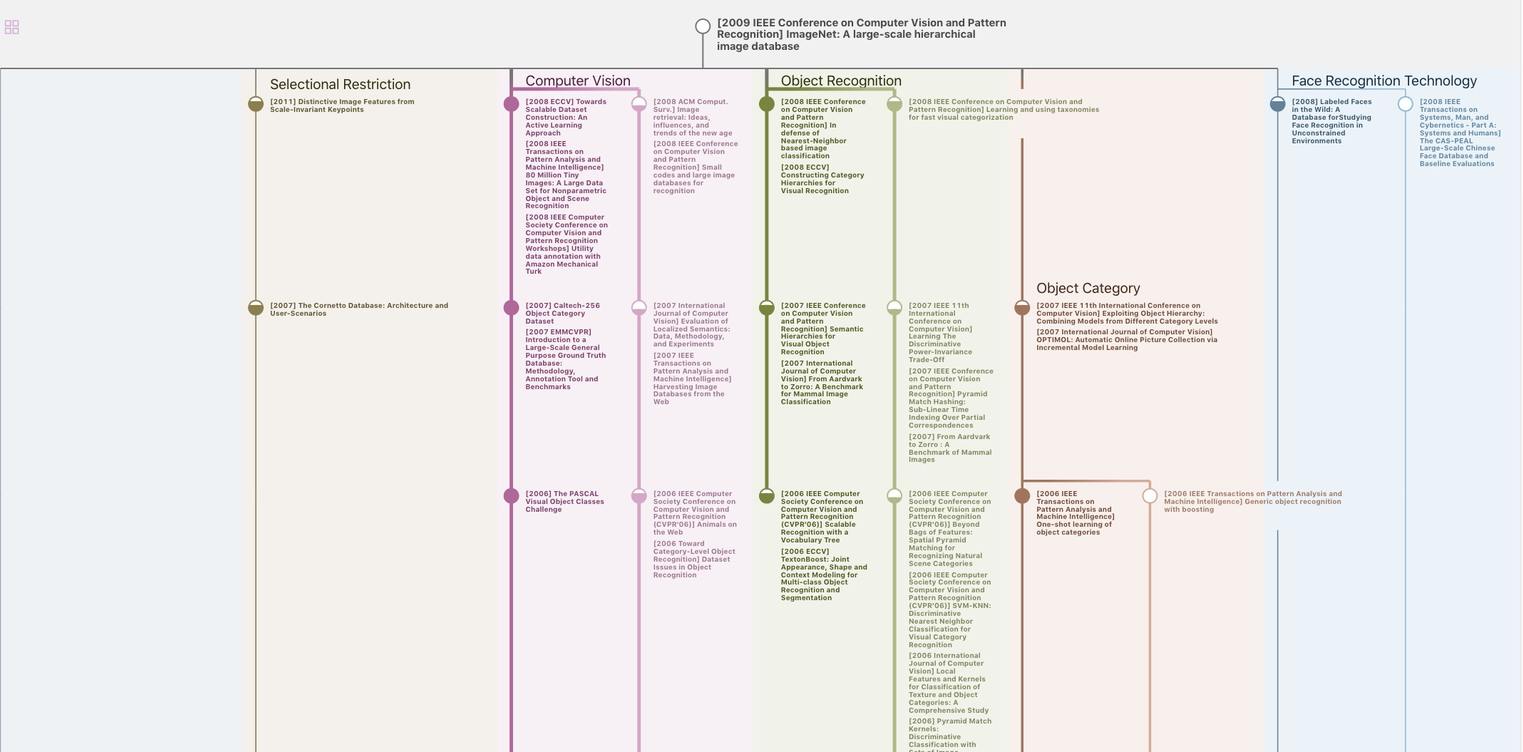
生成溯源树,研究论文发展脉络
Chat Paper
正在生成论文摘要