SecureSL: A Privacy-preserving Vertical Cooperative Learning Scheme for Web 3.0
IEEE Transactions on Network Science and Engineering(2023)
摘要
Web 3.0 is the highly anticipated new evolution of the World Wide Web that promises greater decentralization, intelligence, and security than its predecessors. Neural networks are a powerful tool that can be used to build more intelligent and personalized web. However, traditional centralized learning of neural networks is limited, and the emerging cooperative learning methods such as federated learning are often restricted to horizontally partitioned data. To enable vertical cooperative learning and tackle the associated privacy preservation issues, we propose SecureSL, a vertical cooperative learning scheme based on split learning. We present an edge-end cooperative learning framework that fits the decentralized feature of Web 3.0. We adopt multi-key homomorphic encryption (MKHE) to ensure the confidentiality of the data, the labels and the trained model, making SecureSL resistant to collusion attacks. Furthermore, we employ the single instruction, multiple data (SIMD) technique and optimize the training process to improve the computational efficiency of MKHE. Finally, we evaluate the privacy preservation performance and the model accuracy of SecureSL through extensive experiments. The results show that our proposed scheme provides a robust solution for vertical cooperative learning in Web 3.0 applications which can address the critical privacy concerns while maintaining the model accuracy.
更多查看译文
关键词
Web 3.0,Vertical cooperative learning,Split learning,Privacy-preserving
AI 理解论文
溯源树
样例
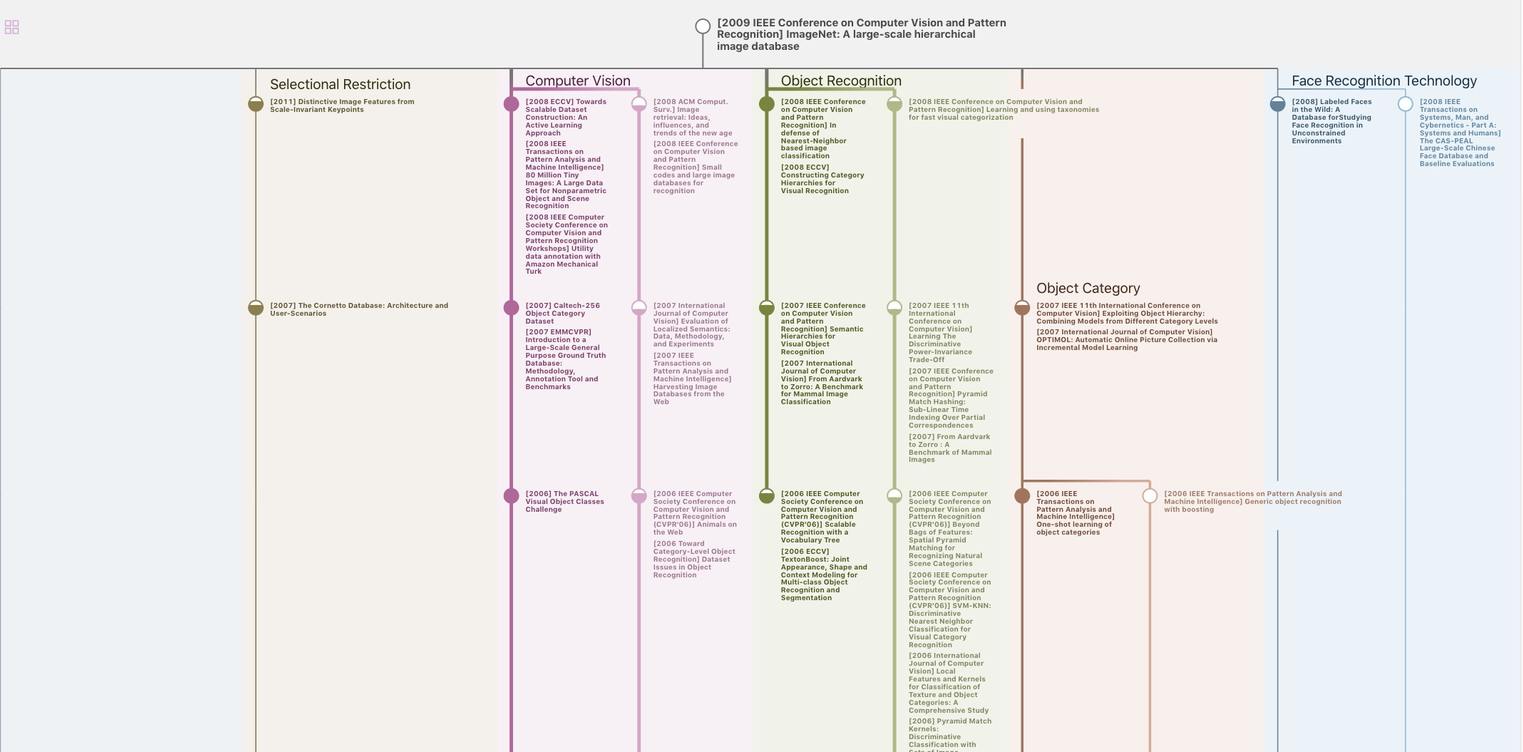
生成溯源树,研究论文发展脉络
Chat Paper
正在生成论文摘要