FedSuper: A Byzantine-Robust Federated Learning Under Supervision
ACM TRANSACTIONS ON SENSOR NETWORKS(2024)
摘要
Federated Learning (FL) is a machine learning setting where multiple worker devices collaboratively train a model under the orchestration of a central server, while keeping the training data local. However, owing to the lack of supervision on worker devices, FL is vulnerable to Byzantine attacks where the worker devices controlled by an adversary arbitrarily generate poisoned local models and send to FL server, ultimately degrading the utility (e.g., model accuracy) of the global model. Most of existing Byzantine-robust algorithms, however, cannot well react to the threatening Byzantine attacks when the ratio of compromised worker devices (i.e., Byzantine ratio) is over 0.5 and worker devices ' local training datasets are not independent and identically distributed (non-IID). We propose a novel Byzantine-robust Federated Learning under Supervision (FedSuper), which can maintain robustness against Byzantine attacks even in the threatening scenario with a very high Byzantine ratio (0.9 in our experiments) and the largest level of non-IID data (1.0 in our experiments) when the state-of-the-art Byzantine attacks are conducted. The main idea of FedSuper is that the FL server supervises worker devices via injecting a shadow dataset into their local training processes. Moreover, according to the local models ' accuracies or losses on the shadow dataset, we design a Local Model Filter to remove poisoned local models and output an optimal global model. Extensive experimental results on three real-world datasets demonstrate the effectiveness and the superior performance of FedSuper, compared to five latest Byzantine-robust FL algorithms and two baselines, in defending
更多查看译文
关键词
Federated learning,Byzantine attack,Byzantine ratio,non-IID
AI 理解论文
溯源树
样例
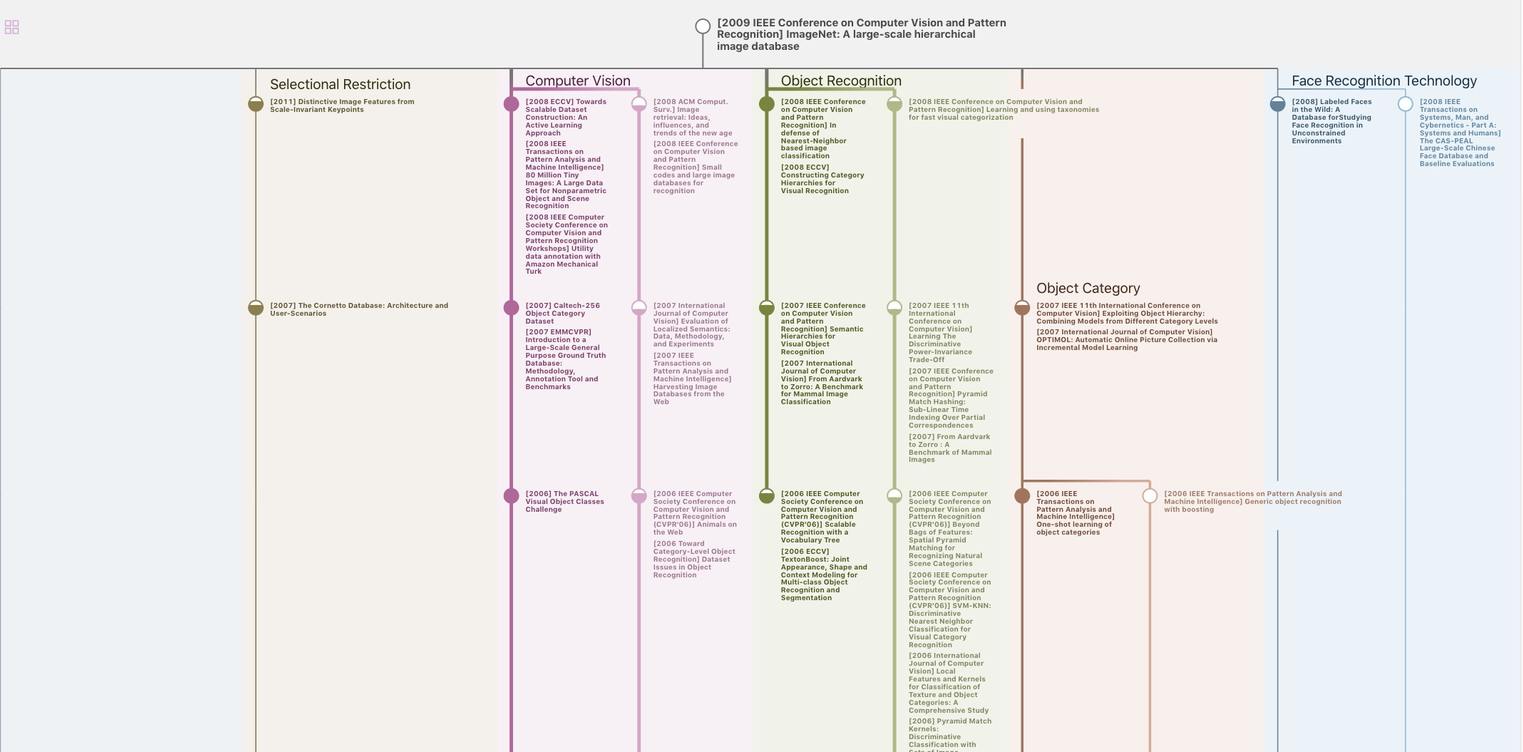
生成溯源树,研究论文发展脉络
Chat Paper
正在生成论文摘要