A statistical approach to detect disparity prone features in a group fairness setting
AI and Ethics(2023)
摘要
The use of machine learning models in decision support systems with high societal impact raised concerns about unfair (disparate) results for different groups of people. When evaluating such unfair decisions, one generally relies on predefined groups that are determined by a set of features that are considered sensitive. However, such an approach is subjective and does not guarantee that these features are the only ones to be considered as sensitive nor that they entail unfair (disparate) outcomes. In this paper, we propose a preprocessing step to address the task of automatically recognizing disparity-prone features that does not require a trained model to verify unfair results. Our proposal is based on the Hilbert-Schmidt independence criterion, which measures the statistical dependence of variable distributions. We hypothesize that if the dependence between the label vector and a candidate is high for a sensitive feature, then the information provided by this feature will entail disparate performance measures between groups. Our empirical results at test our hypothesis and show that several features considered as sensitive in the literature do not necessarily entail disparate (unfair) results.
更多查看译文
关键词
group fairness,disparity,features
AI 理解论文
溯源树
样例
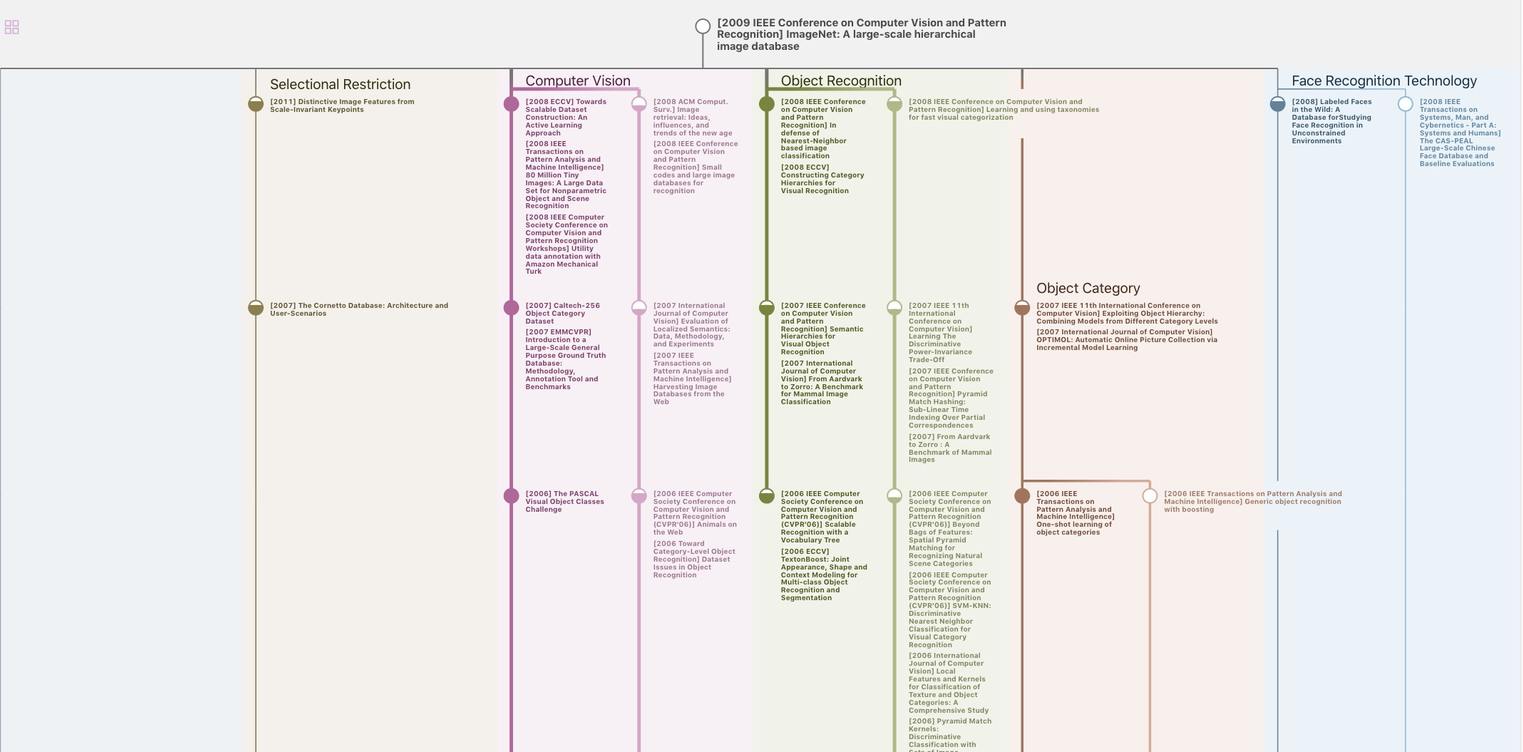
生成溯源树,研究论文发展脉络
Chat Paper
正在生成论文摘要