Viewpoint Disentangling and Generation for Unsupervised Object Re-ID
ACM TRANSACTIONS ON MULTIMEDIA COMPUTING COMMUNICATIONS AND APPLICATIONS(2024)
摘要
Unsupervised object Re-ID aims to learn discriminative identity features from a fully unlabeled dataset to solve the open-class re-identification problem. Satisfying results have been achieved in existing unsupervised Re-ID methods, primarily trained with pseudo-labels created by feature clustering. However, the viewpoint variation of objects is the key challenge, introducing noisy labels in the clustering process. To address this problem, a novel viewpoint disentangling and generation framework (VDG) is proposed to learn viewpoint-invariant ID features, including a disentangling and generation module, as well as a contrastive learning module. First, we design an ID encoder to map the viewpoint and identity features into the latent space. Second, a generator is used to disentangle view features and synthesize images with different orientations. Especially, the well-trained encoder serves as a pre-trained feature extractor in the contrastive learning module. Third, a viewpoint-aware loss and a class-level loss are integrated to facilitate contrastive learning between original and novel views. The generation of novel view images and the application of viewpoint-aware contrastive loss mutually assist model learning viewpoint-invariant ID features. Extensive experiments on Market-1501, DukeMTMC, MSMT17, and VeRi-776 demonstrate the effectiveness of the proposed VDG framework, as well as its superiority over the existing state-of-the-art approaches. The VDG model also demonstrates high quality in the image generation tasks.
更多查看译文
关键词
Person re-identification,unsupervised,generation,disentanglement,viewpoint
AI 理解论文
溯源树
样例
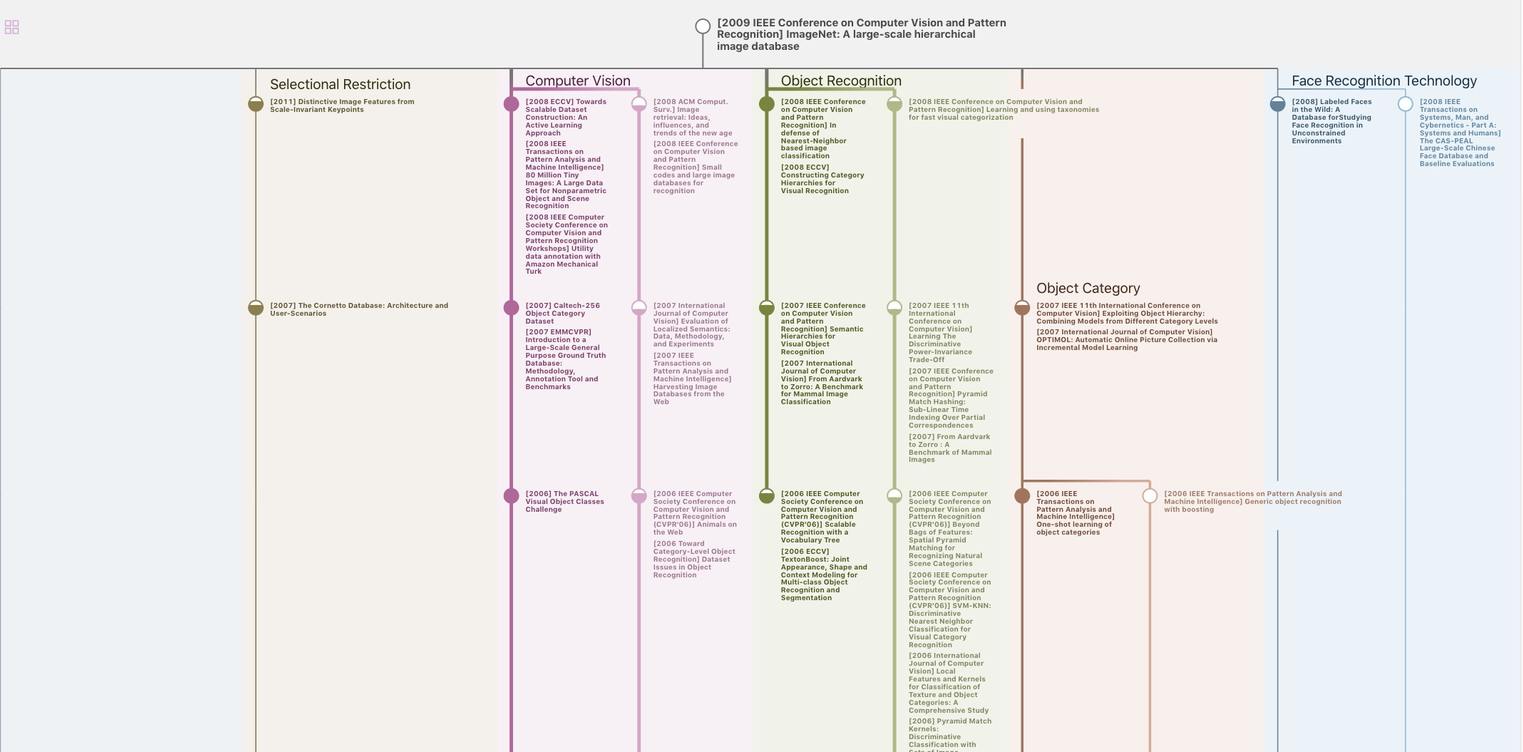
生成溯源树,研究论文发展脉络
Chat Paper
正在生成论文摘要