Siamese Transformer for Group Re-Identification via Multiscale Feature Transform and Joint Learning
IEEE TRANSACTIONS ON INSTRUMENTATION AND MEASUREMENT(2024)
摘要
Group re-identification (GReID) is crucial in intelligent video surveillance for retrieving human groups across cameras. However, existing works mainly focus on group variation challenges, including membership and layout changes, and neglect the occluded groups. To address this, we propose a novel Siamese Transformer for GReID, integrating multiscale feature transform and joint learning. Specifically, the multiscale feature transform includes global feature mapping and local feature encoding. To enhance robustness against occlusions, local feature encoding utilizes a random patch regrouping module (RPRM) and dynamical alignment of local features (DALFs). RPRM rearranges and transforms member patch embeddings, generating local features with diversified coverage for handling occluded groups. DALF dynamically aligns the local features to handle misalignment caused by occlusions. Additionally, we employ joint learning of identification and verification to extract robust and discriminative group representations. Experimental results on three benchmark datasets confirm the effectiveness and superiority of our proposed method.
更多查看译文
关键词
Group re-identification (GReID),joint learning,multiscale feature transform,occlusion,vision transformer (ViT)
AI 理解论文
溯源树
样例
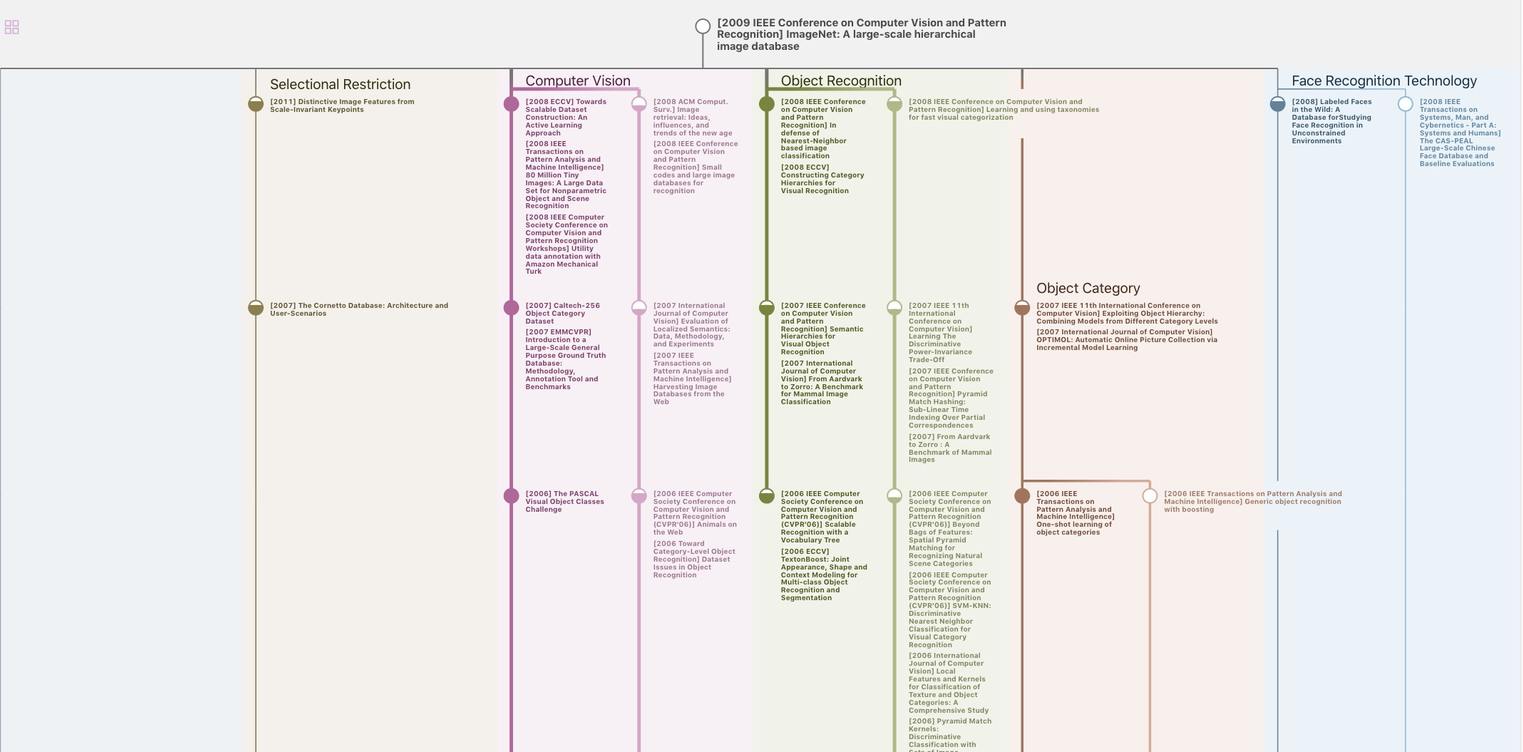
生成溯源树,研究论文发展脉络
Chat Paper
正在生成论文摘要