Improving the Accuracy of Cable-Driven Parallel Robots Through Model Optimization and Machine-Learning
Mechanisms and machine science(2023)
摘要
The accuracy of cable-driven parallel robots (CDPRs) is an important performance criteria in many of their applications. While various modeling and calibration approaches have been proposed to improve the accuracy of CDPRs, only few works in the literature systematically compare the accuracy of different models and approaches in practice. Therefore, this work compares the accuracy improvements achieved by different CDPR and machine-learning (ML) models (linear regression, boosted regression trees, and neural networks) that are optimized or trained based on measurement data from a CDPR. A hyperparameter study is performed to select the most accurate models, which exhibit the least overfitting on a validation dataset. The accuracy of these models is evaluated in practice using an additional test measurement. Optimized CDPR models yield accuracy improvements of up to $$61\%$$ on the training and $$30\%$$ on the validation dataset. The best ML model achieves improvements of $$66\%$$ and $$41\%$$ , respectively. These results show that suitable optimized CDPR and ML models can significantly improve the accuracy of CDPR in practice.
更多查看译文
关键词
parallel robots,model optimization,cable-driven,machine-learning
AI 理解论文
溯源树
样例
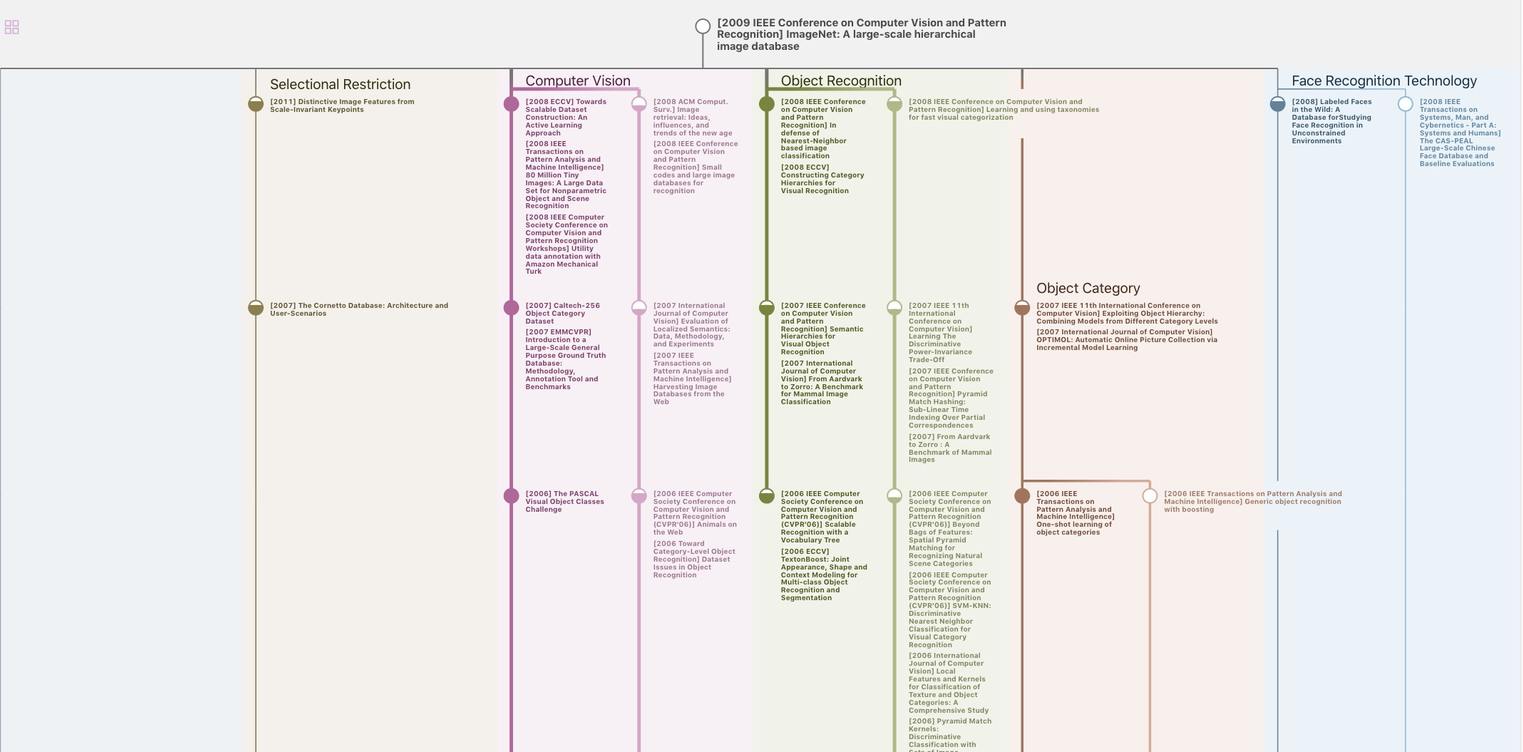
生成溯源树,研究论文发展脉络
Chat Paper
正在生成论文摘要