Exploring Model Depth Adaptation in Image Super-Resolution for Efficient Inference
Communications in computer and information science(2023)
摘要
In the field of single-image super-resolution, different images have varying levels of difficulty in restoration. The restoration for images with a significant proportion of smooth regions is easier than images with complex textures or edge areas. This implies that the restoration for easy images requires fewer computational resources, whereas the restoration for difficult images requires more. Based on this, we have designed Model Depth Adaptation SR (MDASR) network, which can adaptively adjust the capacity of the model based on the difficulty level of the input image restoration. Specifically, we have employed a dynamic structural neural network that incorporates a gating mechanism to adaptively decide whether to execute or skip the current layer based on the current input, thereby achieving adaptive modeling in deep dimension. The experimental results demonstrate that our network significantly reduces FLOPS and achieves the best LPIPS performance on all the test datasets.
更多查看译文
关键词
model depth adaptation,efficient inference,super-resolution
AI 理解论文
溯源树
样例
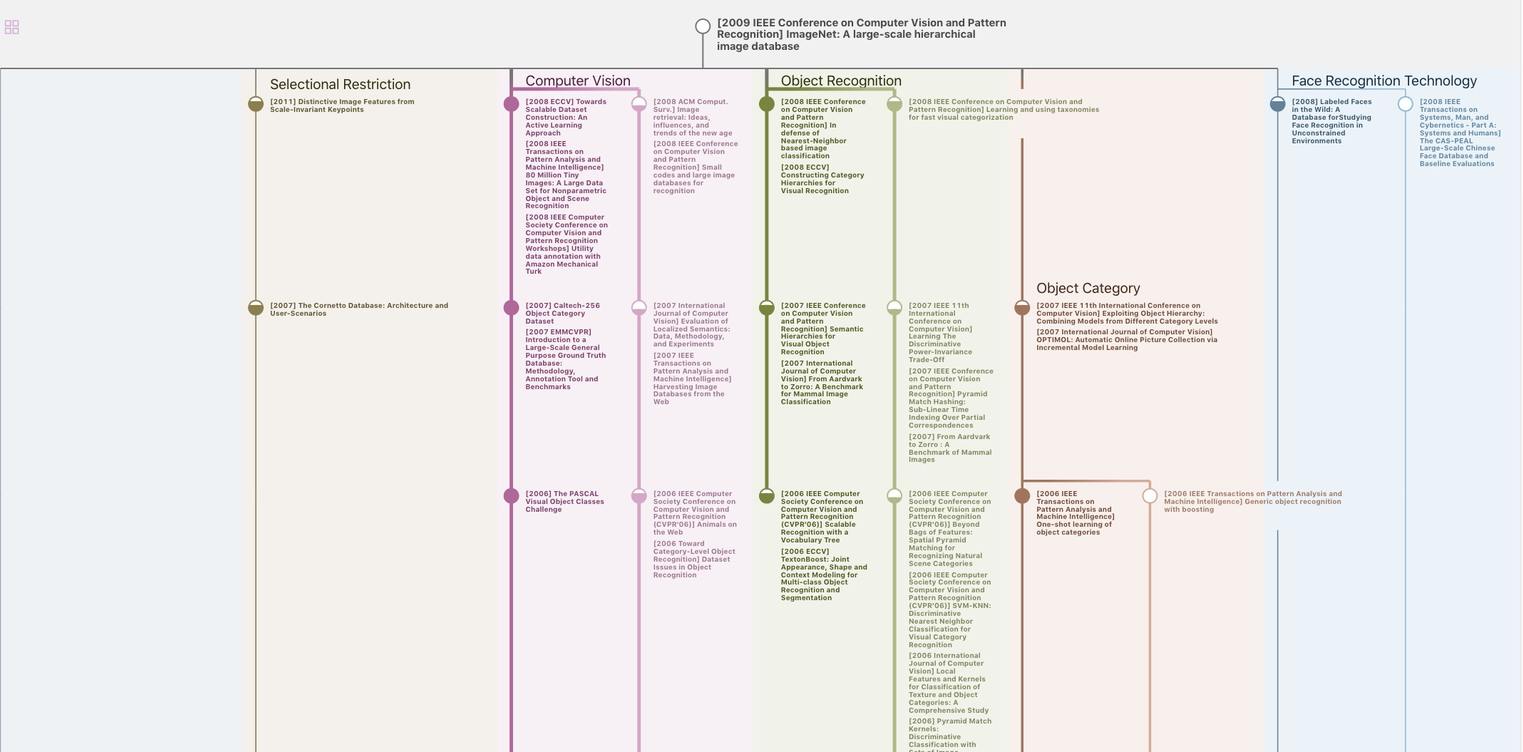
生成溯源树,研究论文发展脉络
Chat Paper
正在生成论文摘要