Proteomic insights into the pathophysiology of hypertension-associated albuminuria: Pilot study in a South African cohort
Clinical Proteomics(2024)
摘要
Background Hypertension is an important public health priority with a high prevalence in Africa. It is also an independent risk factor for kidney outcomes. We aimed to identify potential proteins and pathways involved in hypertension-associated albuminuria by assessing urinary proteomic profiles in black South African participants with combined hypertension and albuminuria compared to those who have neither condition. Methods The study included 24 South African cases with both hypertension and albuminuria and 49 control participants who had neither condition. Protein was extracted from urine samples and analysed using ultra-high-performance liquid chromatography coupled with mass spectrometry. Data were generated using data-independent acquisition (DIA) and processed using Spectronaut™ 15. Statistical and functional data annotation were performed on Perseus and Cytoscape to identify and annotate differentially abundant proteins. Machine learning was applied to the dataset using the OmicLearn platform. Results Overall, a mean of 1,225 and 915 proteins were quantified in the control and case groups, respectively. Three hundred and thirty-two differentially abundant proteins were constructed into a network. Pathways associated with these differentially abundant proteins included the immune system (q-value [false discovery rate] = 1.4 × 10 − 45 ), innate immune system (q = 1.1 × 10 − 32 ), extracellular matrix (ECM) organisation (q = 0.03) and activation of matrix metalloproteinases (q = 0.04). Proteins with high disease scores (76–100% confidence) for both hypertension and chronic kidney disease included angiotensinogen (AGT), albumin (ALB), apolipoprotein L1 (APOL1), and uromodulin (UMOD). A machine learning approach was able to identify a set of 20 proteins, differentiating between cases and controls. Conclusions The urinary proteomic data combined with the machine learning approach was able to classify disease status and identify proteins and pathways associated with hypertension-associated albuminuria.
更多查看译文
关键词
Proteomics,Albuminuria,Hypertension,Ultra-high-performance liquid chromatography,Machine learning
AI 理解论文
溯源树
样例
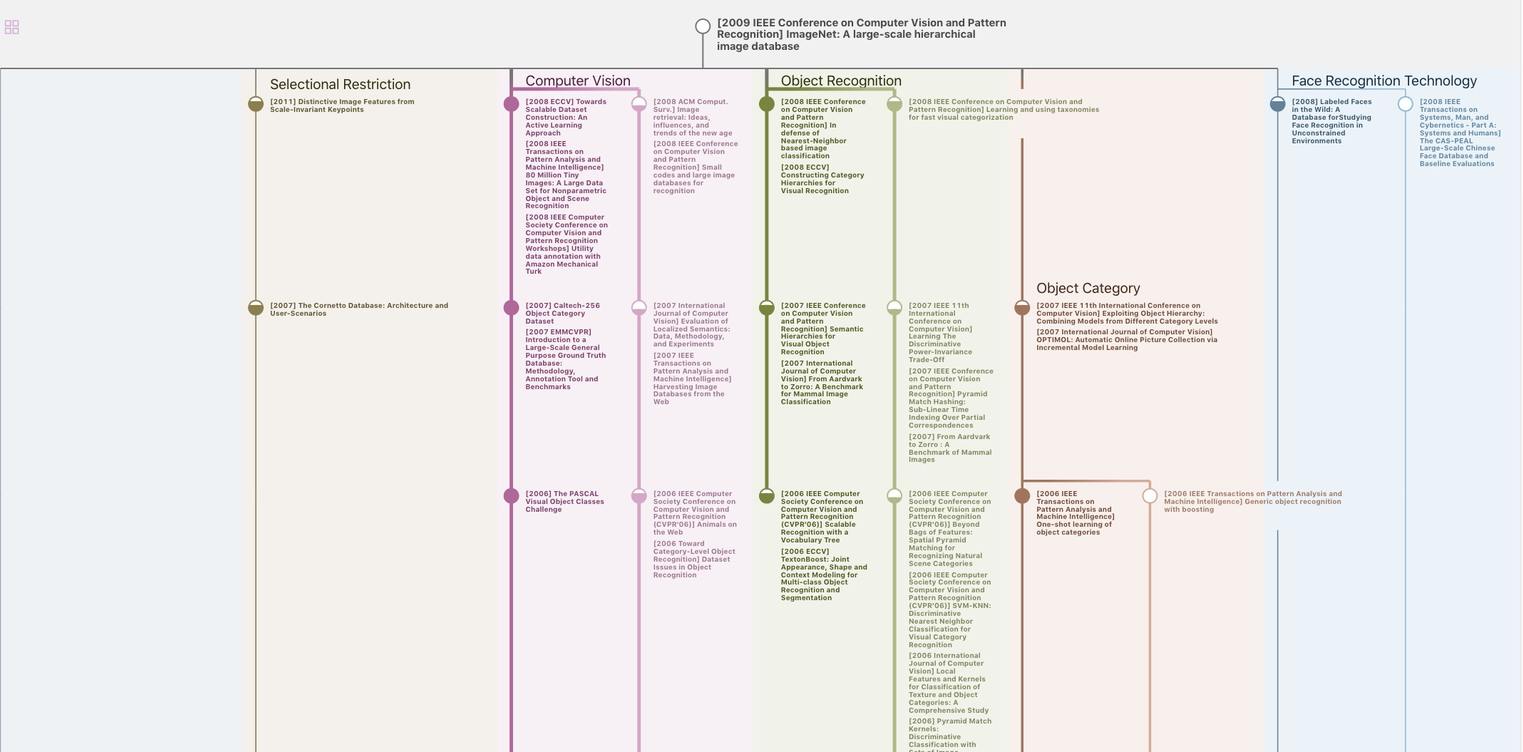
生成溯源树,研究论文发展脉络
Chat Paper
正在生成论文摘要