Proffler: Toward Collaborative and Scalable Edge-Assisted Crowdsourced Livecast
IEEE INTERNET OF THINGS JOURNAL(2024)
摘要
In recent years, crowdsourced livecast has seen remarkable progress due to the interactivity and real-time nature, playing an essential role in multimedia applications in the post-epidemic era. Given the delay sensitivity, large viewing volumes, and heterogeneous viewing patterns, the traditional video streaming methods fail to provide the optimized Quality of Experience (QoE) for viewers using the minimum system cost over an edge-assisted service architecture. The emerging technology of mobile edge computing (MEC) offers a new perspective of reducing user latency and enhancing the quality of dispatched videos in a promising way. In this article, we propose Proffler, an integrated framework that addresses this problem through effective stream caching at the network edge server. We first examine the underlying correlations in viewing patterns across different regions and propose a novel transformer-based algorithm, Chili-TF, that achieves accurate viewer request prediction, even for regions with insufficient data. We then design a scalable algorithm, U2VR, that achieves near-optimal video stream allocation as well as viewer scheduling. Extensive real-data-driven experiments further confirm that Proffler can achieve improvements of 20%-55% in average QoE compared to state-of-the-art solutions.
更多查看译文
关键词
Request scheduling,video stream allocation,viewer request prediction
AI 理解论文
溯源树
样例
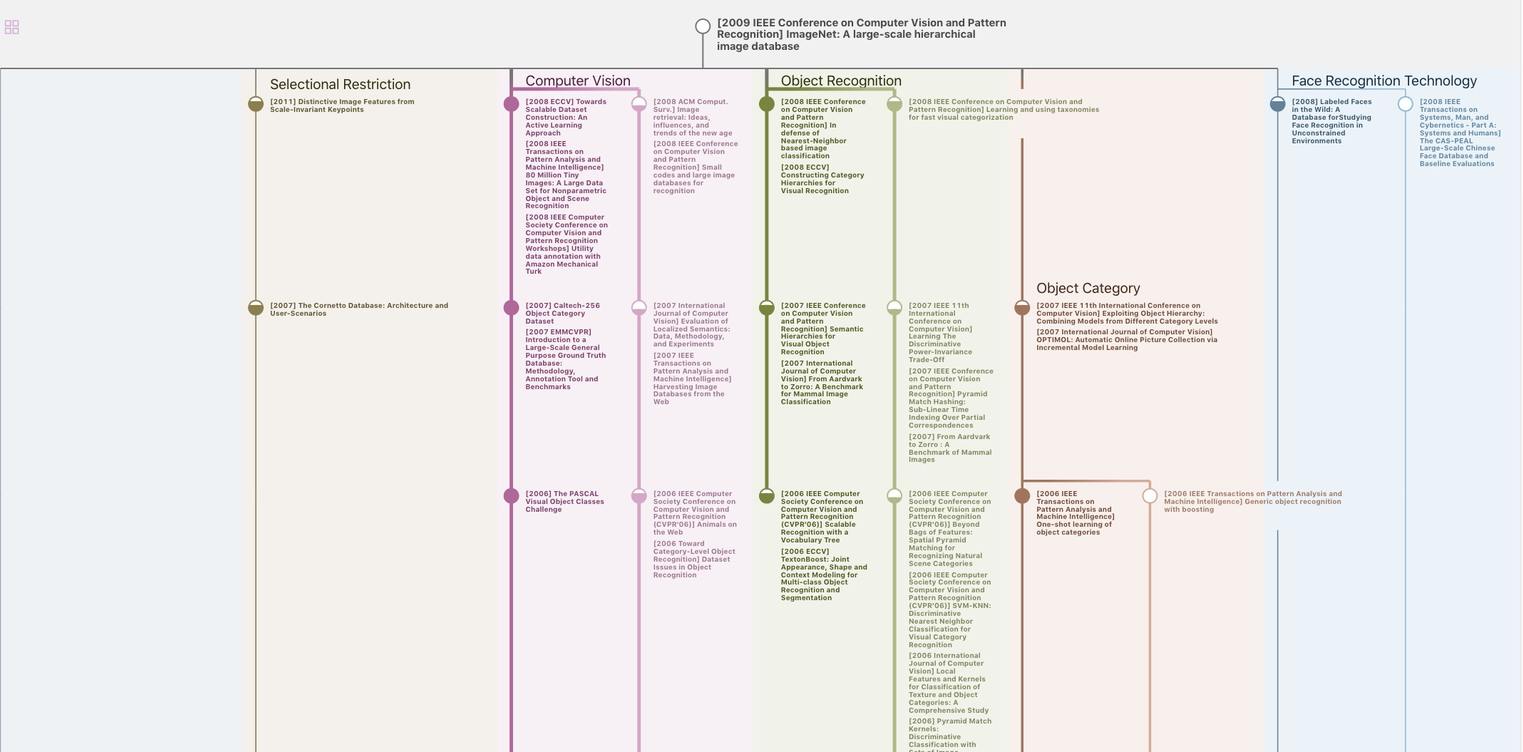
生成溯源树,研究论文发展脉络
Chat Paper
正在生成论文摘要