Application of Machine Learning on Hydrate formation prediction of pure components with water and inhibitors solution
Research Square (Research Square)(2023)
摘要
Abstract Determining gas hydrate formation conditions with pure water and presence of inhibitors are Indispensable for ensuring efficient management of hydrate in various processes such as flow assurance, deepwater drilling, and the advancement of hydrate-based technology. Current work utilizes five machine learning algorithms that are used in the development of prediction models for estimating the temperature of hydrate formation of pure components with pure water and with the addition of inhibitors. The machine learning algorithm tested in this study is the KNN (k-Nearest Neighbor), DTR (Decision Tree Regressor), GBR (Gradient Boosting Regressor), RFR (Random Forest Regressor), and XGBR (Extreme Gradient Boosting Regressor). The model was trained and tested using a comprehensive dataset comprising 3029 experimental data points sourced from various literature sources. The experimental data pertaining to methane, ethane, propane, carbon dioxide, and nitrogen were examined within a temperature range spanning from 148.8 to 323.9 K while maintaining a pressure not exceeding 1000 MPa. k-Nearest Neighbor emerges as the most accurate predictor among the five machine learning methods, boasting an impressive R 2 score of 0.983 and a commendable AARD value of 0.267. Hence, the k-Nearest Neighbor regression method proves to be a reliable technique for forecasting the conditions under which hydrate formation occurs in pure components mixed with water and inhibitors.
更多查看译文
关键词
hydrate formation prediction,machine learning,water
AI 理解论文
溯源树
样例
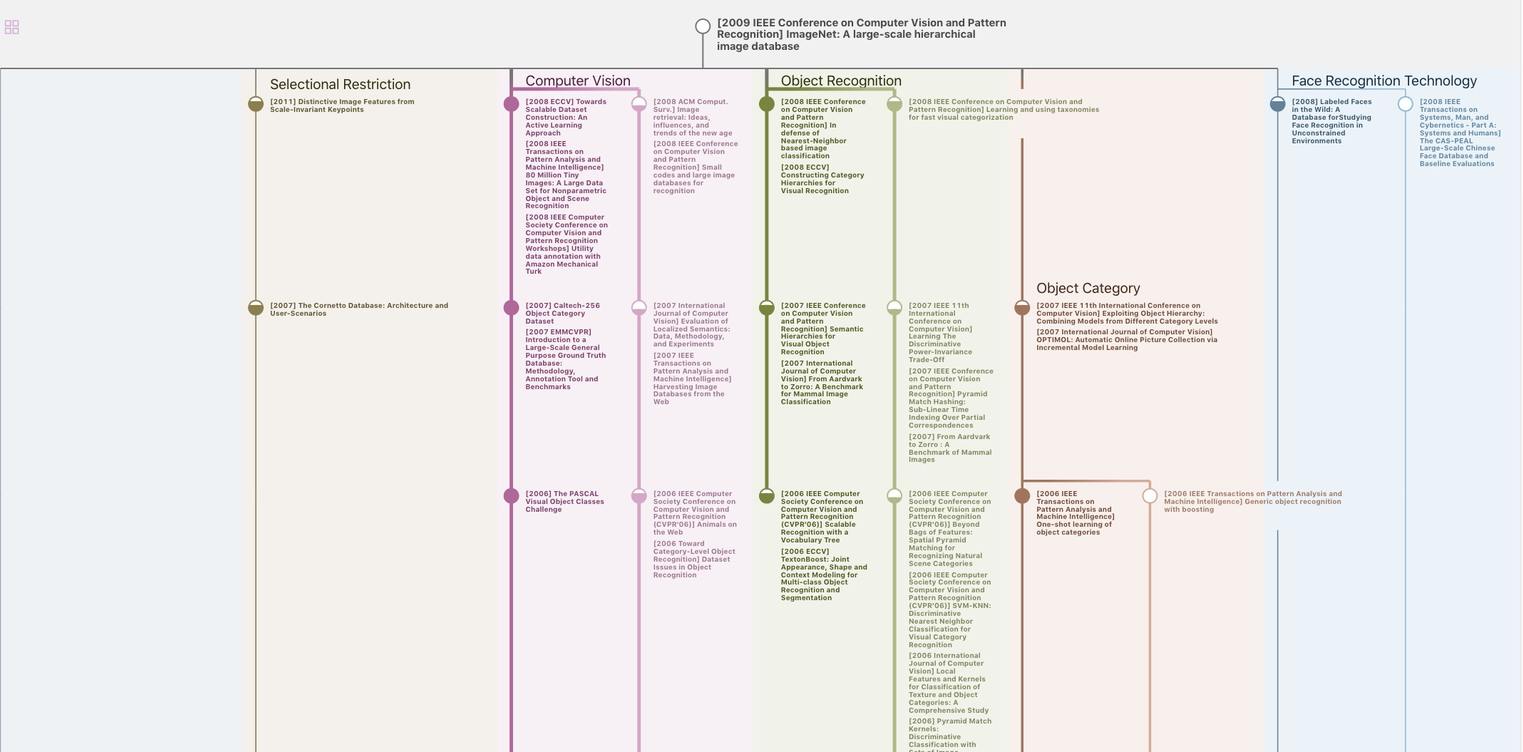
生成溯源树,研究论文发展脉络
Chat Paper
正在生成论文摘要