Schema-Aware Hyper-Relational Knowledge Graph Embeddings for Link Prediction
IEEE Transactions on Knowledge and Data Engineering(2023)
摘要
Knowledge Graph (KG) embeddings have become a powerful paradigm to resolve link prediction tasks for KG completion. The widely adopted triple-based representation, where each triplet
$(h,r,t)$
links two entities
$h$
and
$t$
through a relation
$r$
, oversimplifies the complex nature of the data stored in a KG, in particular for hyper-relational facts, where each fact contains not only a base triplet
$(h,r,t)$
, but also the associated key-value pairs
$(k,v)$
. Even though a few recent techniques tried to learn from such data by transforming a hyper-relational fact into an n-ary representation (i.e., a set of key-value pairs only without triplets), they result in suboptimal models as they are unaware of the triplet structure, which serves as the fundamental data structure in modern KGs and preserves the essential information for link prediction. Moreover, as the KG schema information has been shown to be useful for resolving link prediction tasks, it is thus essential to incorporate the corresponding hyper-relational schema in KG embeddings. Against this background, we propose sHINGE, a schema-aware hyper-relational KG embedding model, which learns from hyper-relational facts directly (without the transformation to the n-ary representation) and their corresponding hyper-relational schema in a KG. Our extensive evaluation shows the superiority of sHINGE on various link prediction tasks over KGs. In particular, compared to a sizeable collection of 21 baselines, sHINGE consistently outperforms the best-performing triple-based KG embedding method, hyper-relational KG embedding method, and schema-aware KG embedding method by 19.1%, 1.8%, and 12.9%, respectively.
更多查看译文
关键词
Hyper-relation,knowledge graph embedding,link prediction,schema
AI 理解论文
溯源树
样例
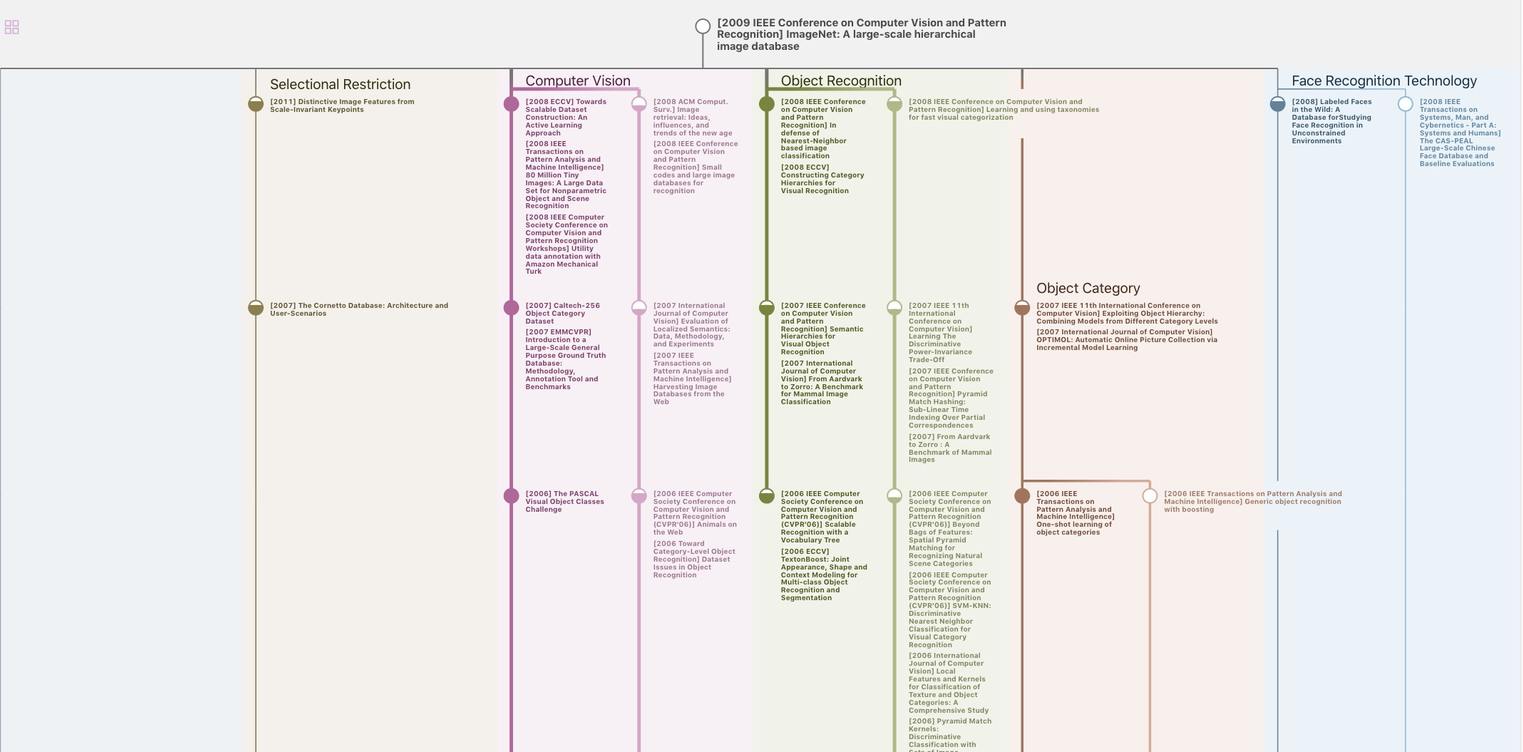
生成溯源树,研究论文发展脉络
Chat Paper
正在生成论文摘要