Joints-Centered Spatial-Temporal Features Fused Skeleton Convolution Network for Action Recognition
IEEE TRANSACTIONS ON MULTIMEDIA(2024)
摘要
Skeleton-based action recognition is crucial for natural human-computer interaction, dynamic behavior analysis, and behavior surveillance. The key challenge is to effectively capture the intrinsic local-global clues of the activity. However, it remains challenging to efficiently leverage multidimensional information related to joints' local visual appearances, global spatial relationships, and coherent temporal cues. To address this challenge, we propose a joints-centered spatial-temporal feature-fused framework for action recognition, which exploits skeleton-based graph diffusion and convolution. Specifically, we employ Partial Differential Equation (PDE) based skeleton graph diffusion to automatically activate and diffuse the salient appearance features of joints. This approach simultaneously integrates the joints' appearance clues and their hierarchical relationships at both the super-pixel level and structure level. The diffused appearance-related features of the joints are further fused with skeleton-related spatial-temporal features, and the resulting fused features are fed into a skeleton convolution network for action recognition. Our method was extensively evaluated on two public datasets (NTU-RGBD and UWA3D), and the results demonstrate the improved accuracy and effectiveness of our approach. Our code will be public.
更多查看译文
关键词
Skeleton,Feature extraction,Convolution,Visualization,Task analysis,Joints,Data mining,Skeleton-based action recognition,spatial-temporal feature fusion,PDE diffusion
AI 理解论文
溯源树
样例
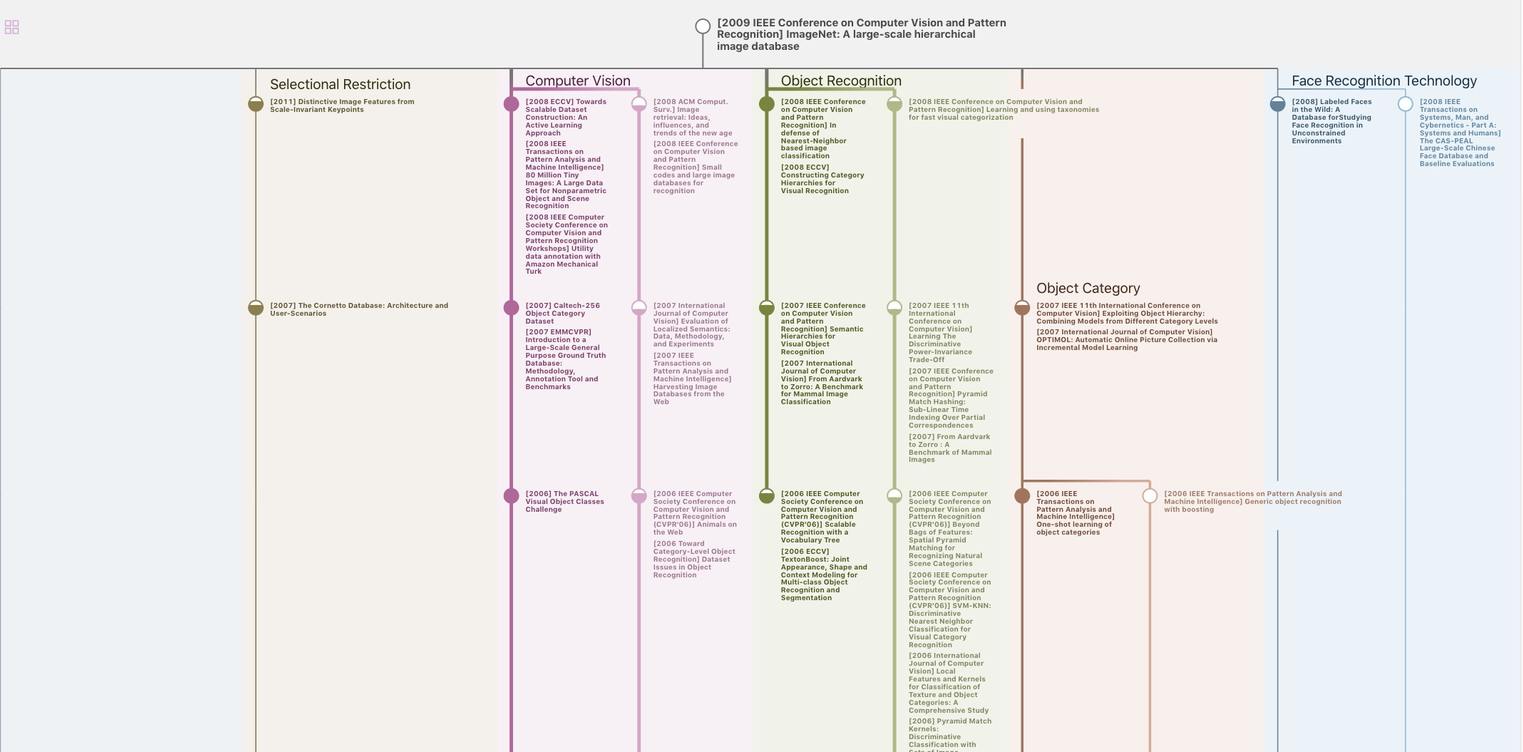
生成溯源树,研究论文发展脉络
Chat Paper
正在生成论文摘要