Progressive Adaptive Chance-Constrained Safeguards for Reinforcement Learning
arXiv (Cornell University)(2023)
摘要
Safety assurance of Reinforcement Learning (RL) is critical for exploration in real-world scenarios. In handling the Constrained Markov Decision Process, current approaches experience intrinsic difficulties in trading-off between optimality and feasibility. Direct optimization methods cannot strictly guarantee state-wise in-training safety while projection-based methods are usually inefficient and correct actions through lengthy iterations. To address these two challenges, this paper proposes an adaptive surrogate chance constraint for the safety cost, and a hierarchical architecture that corrects actions produced by the upper policy layer via a fast Quasi-Newton method. Theoretical analysis indicates that the relaxed probabilistic constraint can sufficiently guarantee forward invariance to the safe set. We validate the proposed method on 4 simulated and real-world safety-critical robotic tasks. Results indicate that the proposed method can efficiently enforce safety (nearly zero-violation), while preserving optimality (+23.8%), robustness and generalizability to stochastic real-world settings.
更多查看译文
关键词
adaptive,reinforcement
AI 理解论文
溯源树
样例
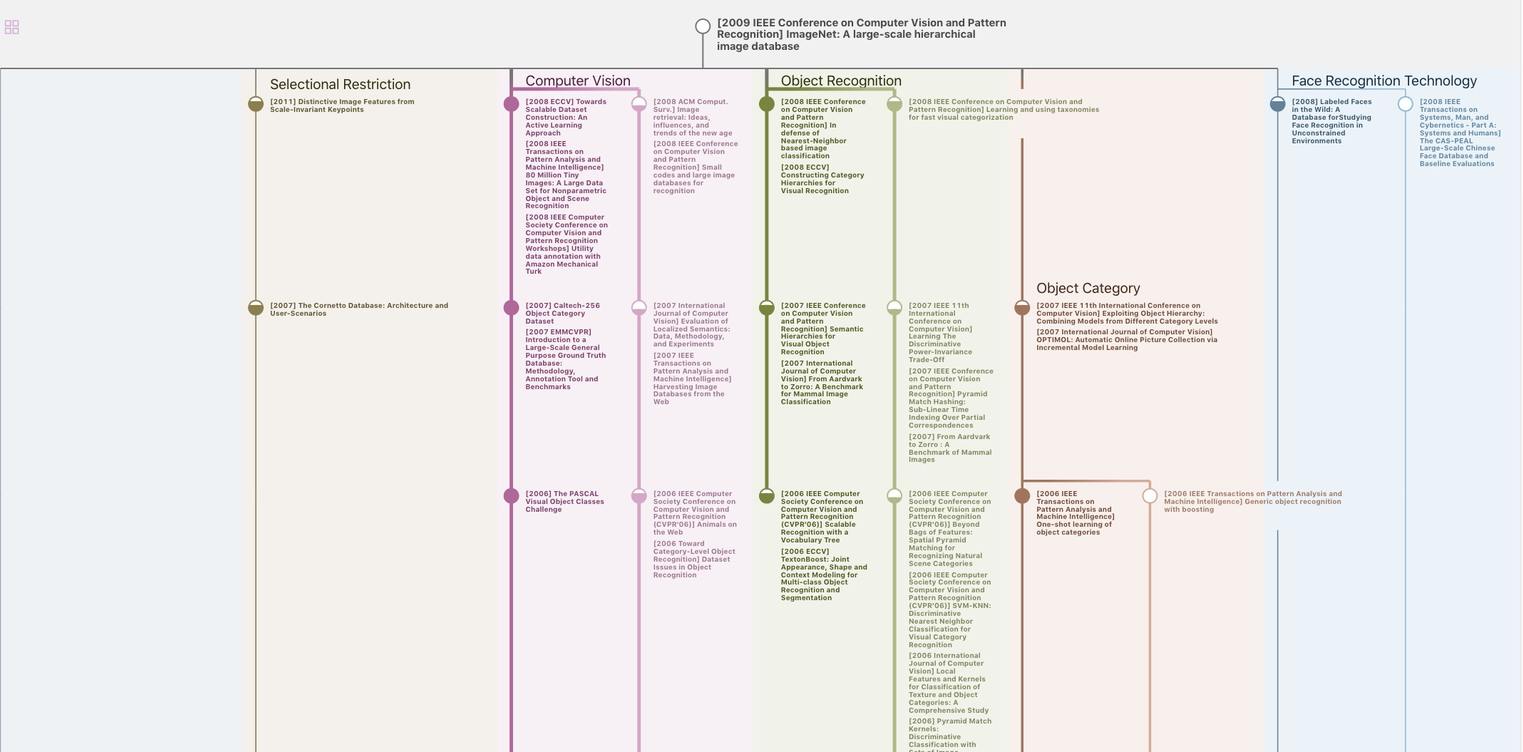
生成溯源树,研究论文发展脉络
Chat Paper
正在生成论文摘要