Human-oriented Representation Learning for Robotic Manipulation
arXiv (Cornell University)(2023)
摘要
Humans inherently possess generalizable visual representations that empower them to efficiently explore and interact with the environments in manipulation tasks. We advocate that such a representation automatically arises from simultaneously learning about multiple simple perceptual skills that are critical for everyday scenarios (e.g., hand detection, state estimate, etc.) and is better suited for learning robot manipulation policies compared to current state-of-the-art visual representations purely based on self-supervised objectives. We formalize this idea through the lens of human-oriented multi-task fine-tuning on top of pre-trained visual encoders, where each task is a perceptual skill tied to human-environment interactions. We introduce Task Fusion Decoder as a plug-and-play embedding translator that utilizes the underlying relationships among these perceptual skills to guide the representation learning towards encoding meaningful structure for what's important for all perceptual skills, ultimately empowering learning of downstream robotic manipulation tasks. Extensive experiments across a range of robotic tasks and embodiments, in both simulations and real-world environments, show that our Task Fusion Decoder consistently improves the representation of three state-of-the-art visual encoders including R3M, MVP, and EgoVLP, for downstream manipulation policy-learning. Project page: https://sites.google.com/view/human-oriented-robot-learning
更多查看译文
关键词
representation learning,manipulation,human-oriented
AI 理解论文
溯源树
样例
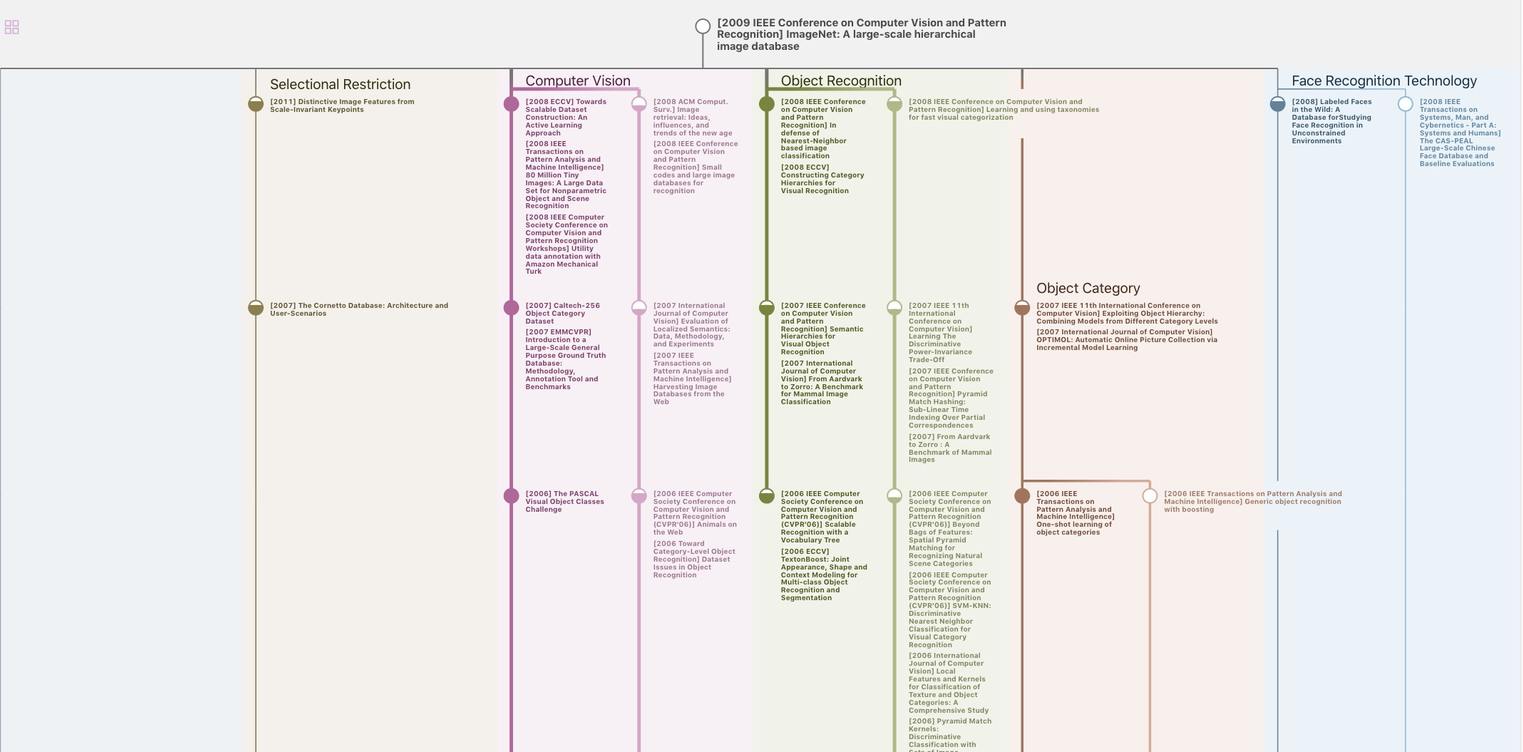
生成溯源树,研究论文发展脉络
Chat Paper
正在生成论文摘要