Transferring experiences in k-nearest neighbors based multiagent reinforcement learning: an application to traffic signal control
Ai Communications(2023)
摘要
The increasing demand for mobility in our society poses various challenges to traffic engineering, computer science in general, and artificial intelligence in particular. Increasing the capacity of road networks is not always possible, thus a more efficient use of the available transportation infrastructure is required. Another issue is that many problems in traffic management and control are inherently decentralized and/or require adaptation to the traffic situation. Hence, there is a close relationship to multiagent reinforcement learning. However, using reinforcement learning poses the challenge that the state space is normally large and continuous, thus it is necessary to find appropriate schemes to deal with discretization of the state space. To address these issues, a multiagent system with agents learning independently via a learning algorithm was proposed, which is based on estimating Q-values from k-nearest neighbors. In the present paper, we extend this approach and include transfer of experiences among the agents, especially when an agent does not have a good set of k experiences. We deal with traffic signal control, running experiments on a traffic network in which we vary the traffic situation along time, and compare our approach to two baselines (one involving reinforcement learning and one based on fixed times). Our results show that the extended method pays off when an agent returns to an already experienced traffic situation.
更多查看译文
关键词
multiagent reinforcement learning,reinforcement learning,k-nearest
AI 理解论文
溯源树
样例
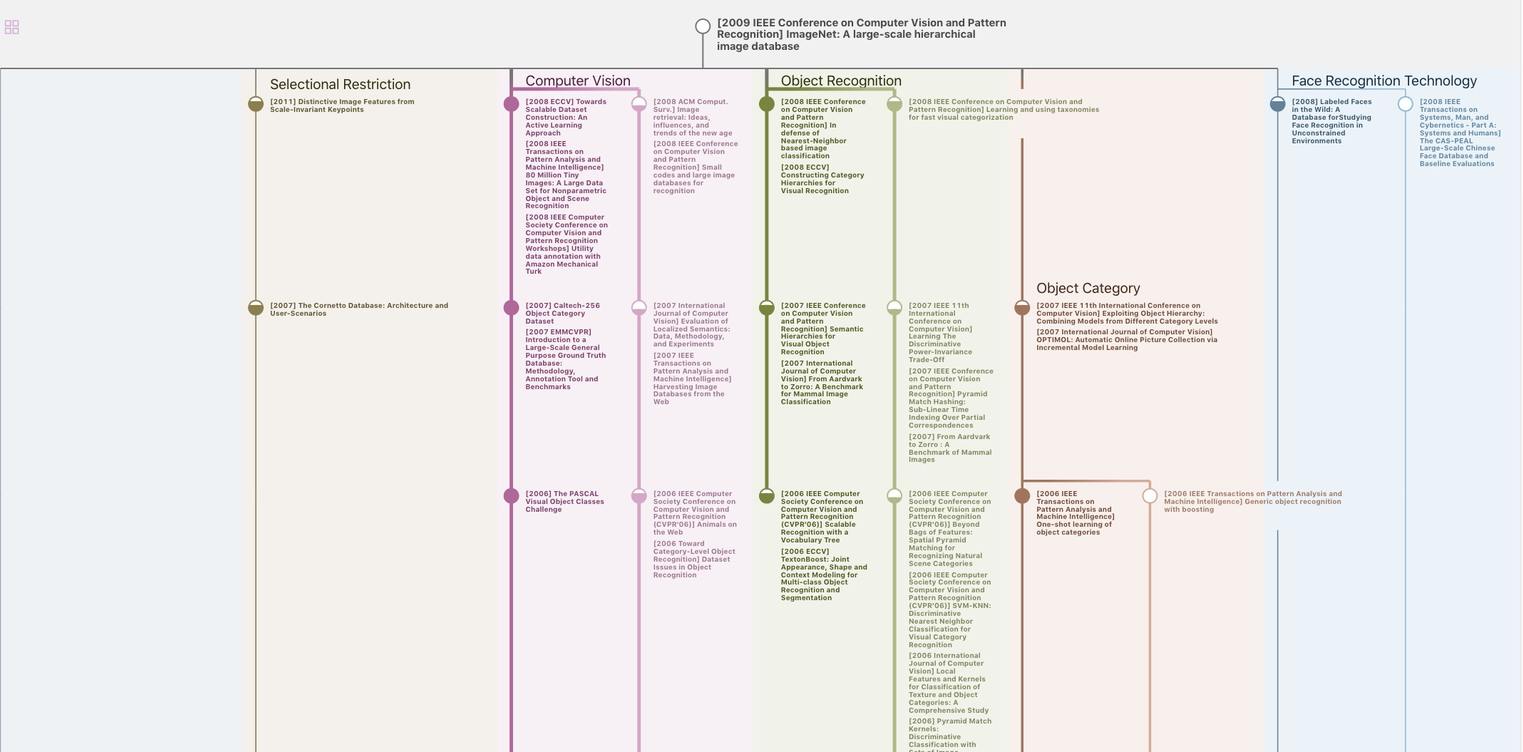
生成溯源树,研究论文发展脉络
Chat Paper
正在生成论文摘要