Label Enhanced Graph Attention Network for Truth Inference
ARTIFICIAL NEURAL NETWORKS AND MACHINE LEARNING, ICANN 2023, PT IV(2023)
摘要
Crowdsourcing platforms tend to provide redundant noise labels when annotating data for machine learning, which promotes truth inference to be proposed for crowdsourced labels denoising. Existing truth inference methods have effectively improved the model's inference quality by utilizing Graph Neural Networks (GNNs) to mine potential crowdsourcing relationships. However, the GNN-based methods fail to reflect crowdsourcing behaviors comprehensively when mining crowdsourcing relationships in a task-worker graph, which limits their performance. To cope with this challenge, we propose a Label Enhanced Graph Attention Network for truth inference, which realizes GNN-based truth inference on complete crowdsourcing behaviors to explore latent crowdsourcing relationships between tasks and workers more effectively. Specifically, we construct an attentive label semantic propagation module based on a graph attention network, which propagates crowdsourced label semantics into the process of information aggregation to represent complete crowdsourcing behaviors. In addition, we design a label-aware graph encoder, which captures the semantics of crowdsourced labels more efficiently by reducing the difference between the predicted and the actual distribution of crowdsourced labels. Extensive experiments on eight real-world datasets demonstrate that LEGAT outperforms eight state-of-theart methods.
更多查看译文
关键词
Truth inference,Crowdsourcing,Graph attention network,Label-aware propagation
AI 理解论文
溯源树
样例
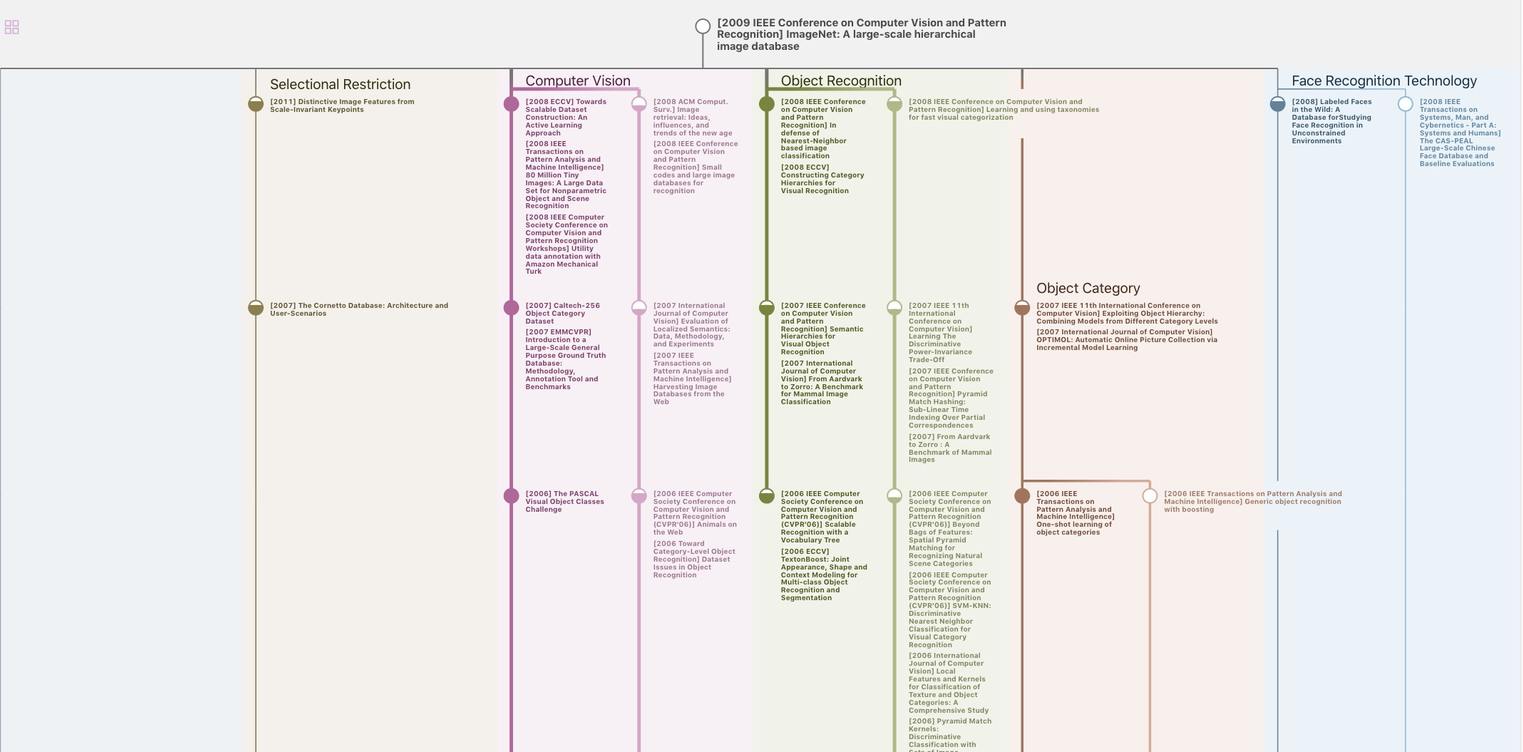
生成溯源树,研究论文发展脉络
Chat Paper
正在生成论文摘要