Performance and Failure Cause Estimation for Machine Learning Systems in the Wild
COMPUTER VISION SYSTEMS, ICVS 2023(2023)
摘要
Machine learning systems at the edge may fail as the real world data can be noisy and have different distribution from the training dataset which the machine learning systems were developed on. However, it is very difficult to detect the system failures and identify root cause of the failures for systems on the edge devices due to many factors such as privacy concerns, regulations, constrained computation resources and expensive error labeling. In this work, we propose a flexible and general framework, PERF, to estimate the performance of a machine learning system deployed at the edge device and identify the root cause of failure if it fails. PERFis similar yet different from the classic teacher-student paradigm. Within PERF, a larger performance estimation model PE is deployed along with the smaller target system T to be evaluated on the same edge device. While the device is idle, PE can be activated and predicts T 's performance and the failure causes from T 's internal and outputs features on the device without human intervention. The privacy risk can be avoided as the evaluation is done on the edge device without sending any user data to the backend cloud. We validated PERFon two exemplar tasks and showed promising results.
更多查看译文
关键词
Machine learning,Performance evaluation
AI 理解论文
溯源树
样例
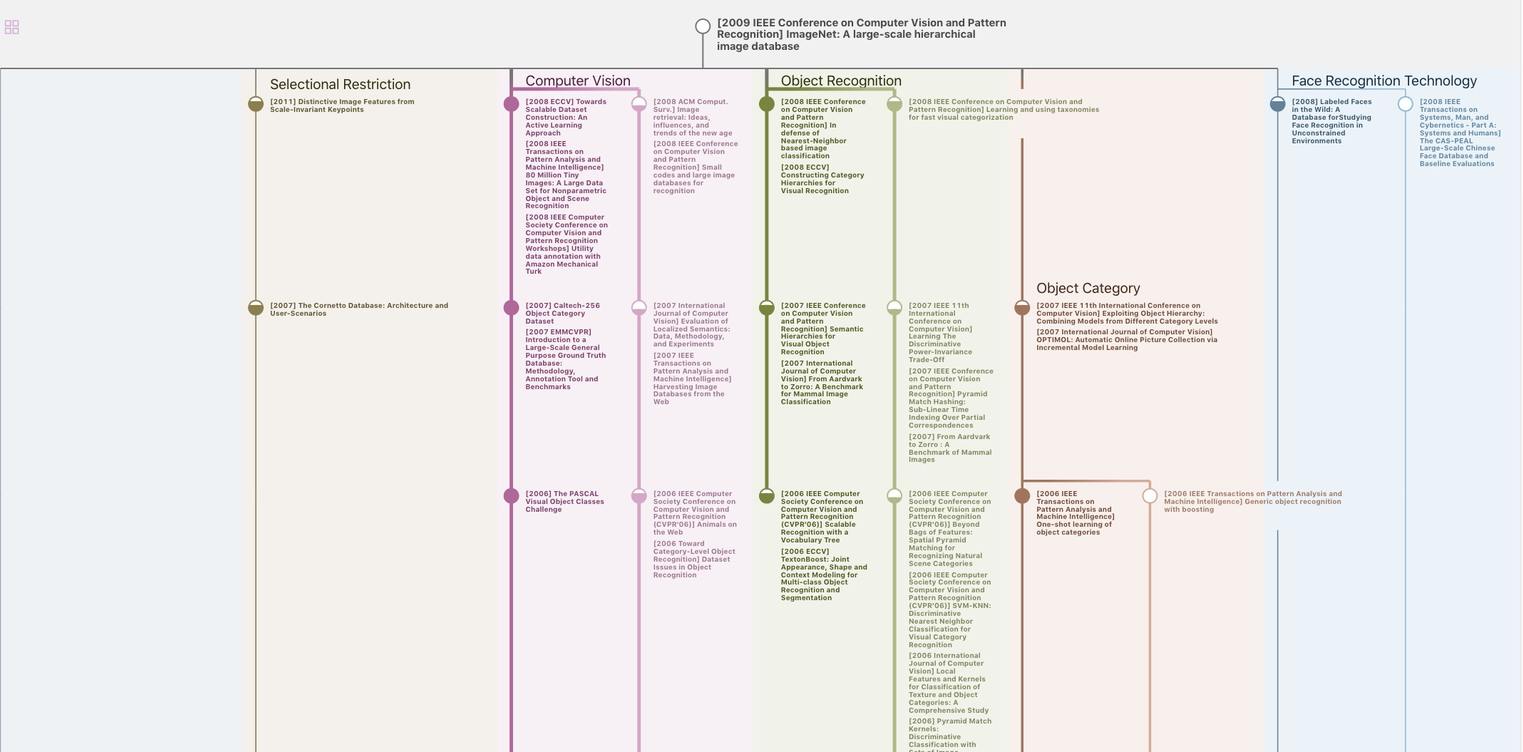
生成溯源树,研究论文发展脉络
Chat Paper
正在生成论文摘要