Data-Driven Predictive Control (DDPC) with Deep Neural Networks for Building Energy Savings
Environmental science and engineering(2023)
摘要
Building model predictive control (MPC) relies on a white- or grey-box model that can require significant time and domain knowledge to develop and calibrate, often on an ad hoc basis. Black-box models can be developed and trained with limited domain knowledge and are easily transferable. In this study, a deep neural network is trained to predict indoor temperature response using a few easily obtained predictors. The trained network is embedded within an MPC framework, replacing a grey-box model, and this data-driven predictive controller (DDPC) is implemented in a facility consisting of two side-by-side identical office spaces. One office is controlled by DDPC; the other remains under automated control. Experimental results show that DDPC reduces energy consumption by up to 30% compared to baseline control while maintaining indoor temperature throughout the day. DDPC presents a scalable solution to the challenges associated with developing and implementing building MPC on a large scale.
更多查看译文
关键词
building energy savings,predictive control,deep neural networks,ddpc,data-driven
AI 理解论文
溯源树
样例
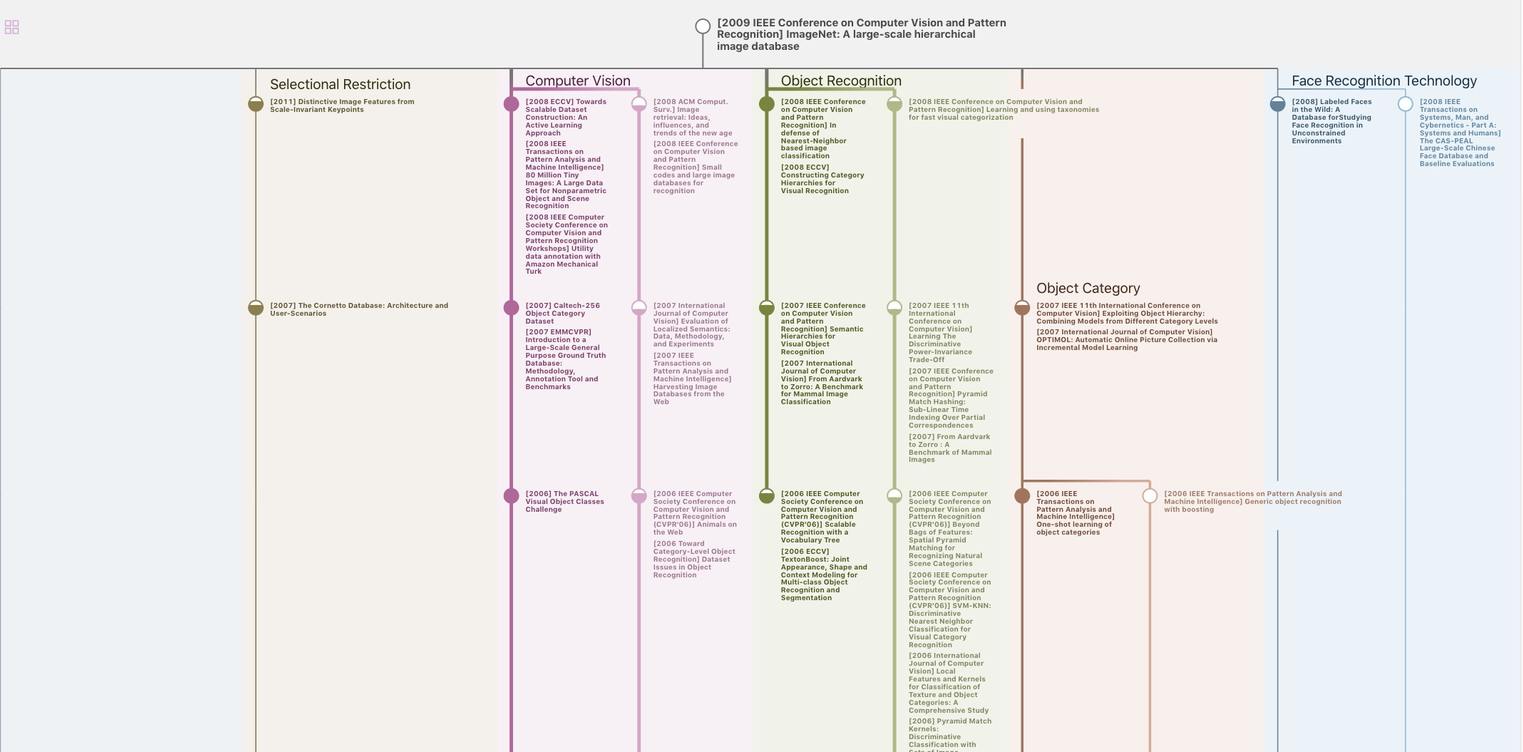
生成溯源树,研究论文发展脉络
Chat Paper
正在生成论文摘要