Reality-enhanced synthetic image training dataset for computer vision construction monitoring
Proceedings of International Structural Engineering and Construction(2023)
摘要
Recent growth in the availability of aerial and ground-level images from construction sites has created a surge in computer vision-based techniques for construction monitoring applications. To address the need for large volumes of visual data with ground truth for training the underlying machine learning models, the application of synthetic data, particularly from 3D/4D BIM, has gained traction in the research community. However, the existing domain gap between real and synthetic images, such as rendering noise from repeated material texture patterns, randomized positional camera parameters that collect the ground truth of BIM elements, and the poorly visibility of BIM elements to the camera viewpoints within these environments, has negatively impacted the potential use of these synthetic datasets as scale. To address these limitations, this paper presents a new synthetic image generation pipeline that optimizes and integrates camera extrinsic parameters with a novel synthetic image appearance enhancement technique to generate high volume of quality synthetic data with respective ground-truth. The use of synthetic datasets for training progress monitoring models is validated through several real-data segmentation cases that incorporate: 1) the automatic collection of synthetic images and ground-truth annotations from high-LoD BIM model disciplines, 2) optimization of positional camera parameters using element visibility metrics, and 3) the enhancement of realism of synthetic images using a patch-based generative approach. The benefits and the current limitations to automated progress monitoring, as well as robotic path automation and optimization for progress motoring real data collection are discussed.
更多查看译文
关键词
vision,construction,training,image,reality-enhanced
AI 理解论文
溯源树
样例
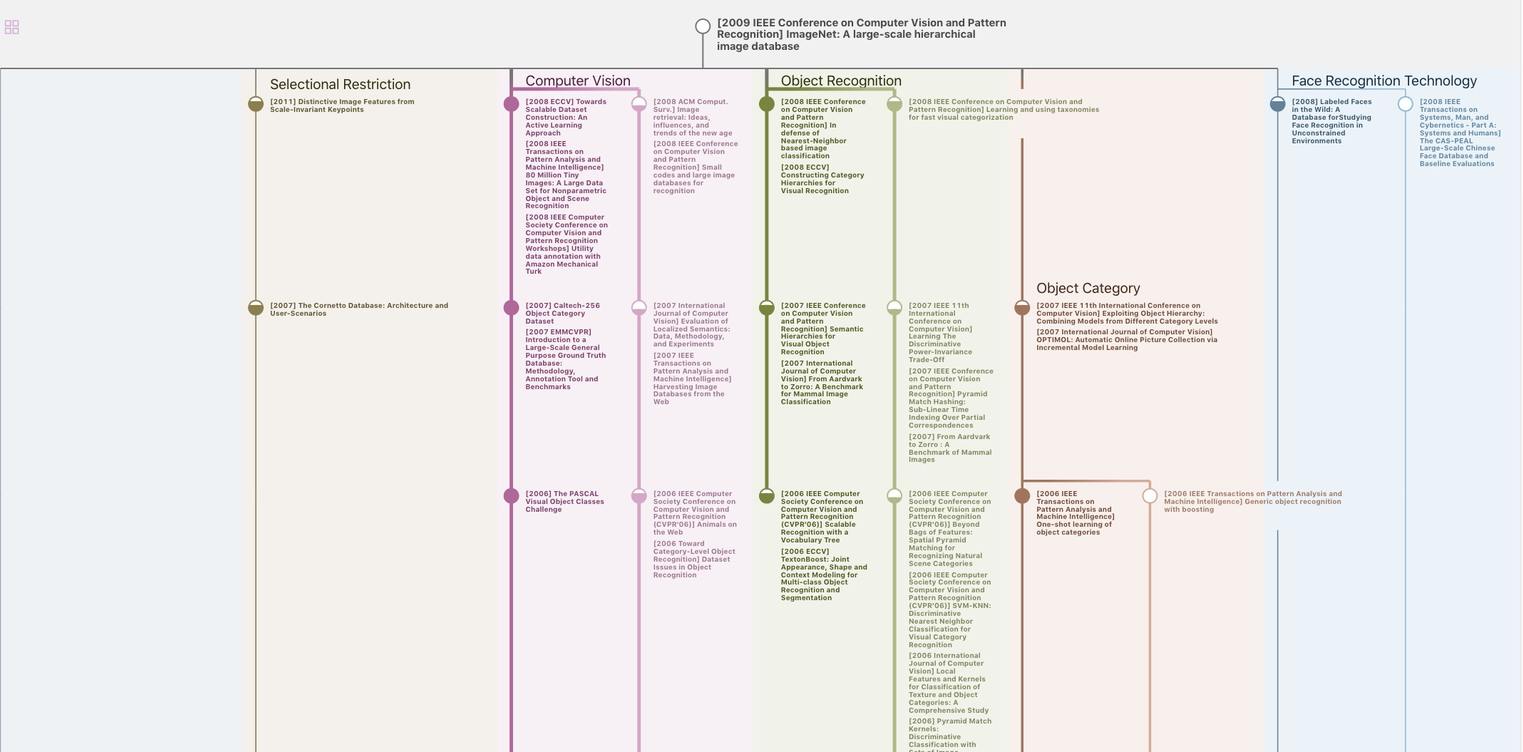
生成溯源树,研究论文发展脉络
Chat Paper
正在生成论文摘要