Advancements in ct lung cancer diagnosis: the role of deep learning for effective classification
Journal of Medical Imaging and Radiation Sciences(2023)
摘要
Objective The study aims to explore the effectiveness of deep learning techniques in classifying CT lung cancer images into three groups: benign, malignant, and normal, with a focus on enhancing diagnostic accuracy and efficiency. MATERIALS & METHODS Lung CT images were collected, comprising normal (N=416), malignant (N=561), and benign (N=120) cases. Convolutional neural network (CNN) models, including EfficientNetB0, InceptionV3, MobileNetV2, ResNet101, and ResNet50, were employed with transfer learning. Hyperparameters included the use of optimizers (SGDM, ADAM, RMSprop), a batch size of 5, 10 epochs, and a learning rate of 1e-3. A 2-fold cross-validation schema was utilized for model validation. The accuracy and Kappa value were used to evaluate the performance of the models. RESULTS The MobileNetV2 model with the SGDM optimizer achieved the highest accuracy of 0.9945 and a Kappa value of 0.9906 among all tested models. CONCLUSION The RESULTS: demonstrate the potential of deep learning techniques, particularly the MobileNetV2 model with the SGDM optimizer, for effective classification of CT lung cancer images into benign, malignant, and normal categories. This study's findings contribute to the advancement of CT lung cancer diagnosis and emphasize the role of deep learning in improving diagnostic accuracy and efficiency. The study aims to explore the effectiveness of deep learning techniques in classifying CT lung cancer images into three groups: benign, malignant, and normal, with a focus on enhancing diagnostic accuracy and efficiency. Lung CT images were collected, comprising normal (N=416), malignant (N=561), and benign (N=120) cases. Convolutional neural network (CNN) models, including EfficientNetB0, InceptionV3, MobileNetV2, ResNet101, and ResNet50, were employed with transfer learning. Hyperparameters included the use of optimizers (SGDM, ADAM, RMSprop), a batch size of 5, 10 epochs, and a learning rate of 1e-3. A 2-fold cross-validation schema was utilized for model validation. The accuracy and Kappa value were used to evaluate the performance of the models. The MobileNetV2 model with the SGDM optimizer achieved the highest accuracy of 0.9945 and a Kappa value of 0.9906 among all tested models. The RESULTS: demonstrate the potential of deep learning techniques, particularly the MobileNetV2 model with the SGDM optimizer, for effective classification of CT lung cancer images into benign, malignant, and normal categories. This study's findings contribute to the advancement of CT lung cancer diagnosis and emphasize the role of deep learning in improving diagnostic accuracy and efficiency.
更多查看译文
关键词
ct lung cancer diagnosis,deep learning,lung cancer,effective classification
AI 理解论文
溯源树
样例
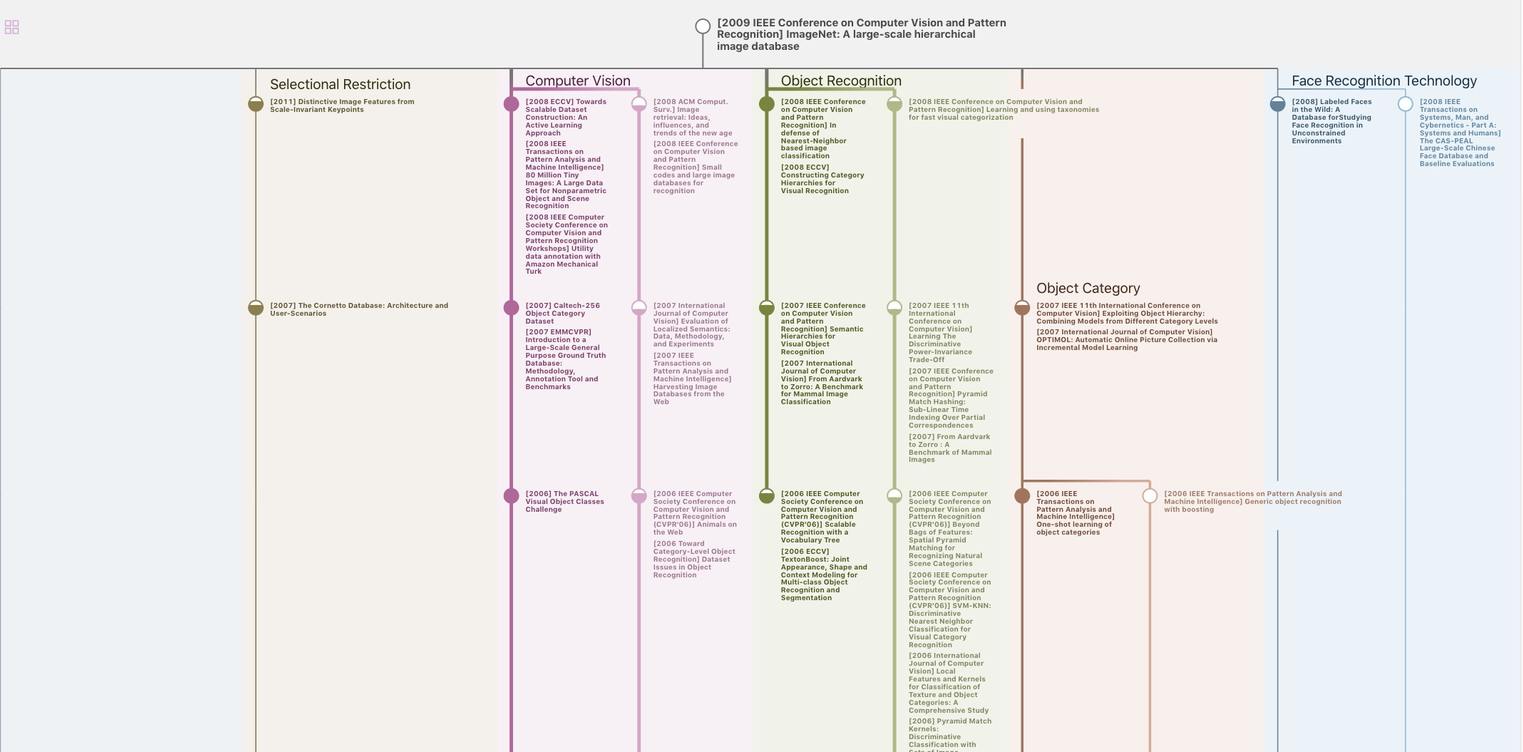
生成溯源树,研究论文发展脉络
Chat Paper
正在生成论文摘要