A Low-cost and Scalable Framework to Build Large-Scale Localization Benchmark for Augmented Reality
IEEE Transactions on Circuits and Systems for Video Technology(2023)
摘要
Nowadays the application of AR is expanding from small or medium environments to large-scale environments, where the visual-based localization in the large-scale environments becomes a critical demand. Current visual-based localization techniques face robustness challenges in complex large-scale environments, requiring tremendous number of data with groundtruth localization for algorithm benchmarking or model training. The previous groundtruth solutions can only be used outdoors, or require high equipment/labor costs, so they cannot be scalable to large environments for both indoors and outdoors, nor can they produce large amounts of data at a feasible cost. In this work, we propose LSFB, a novel low-cost and scalable framework to build localization benchmark in large-scale indoor and outdoor environments. The key is to reconstruct an accurate HD map of the environment. For each visual-inertial sequence captured in the environment, the groundtruth poses are obtained by joint optimization taking both the HD map and visual-inertial constraints. The experiments demonstrate the obtained groundtruth poses have cm-level accuracy. We use the proposed method to collect a localization dataset by mobile phones and AR glasses in various environments with various motions, and release the dataset as the first large-scale localization benchmark for AR.
更多查看译文
关键词
Augmented Reality (AR),Benchmark,SLAM,Visual Localization,Indoor Localization
AI 理解论文
溯源树
样例
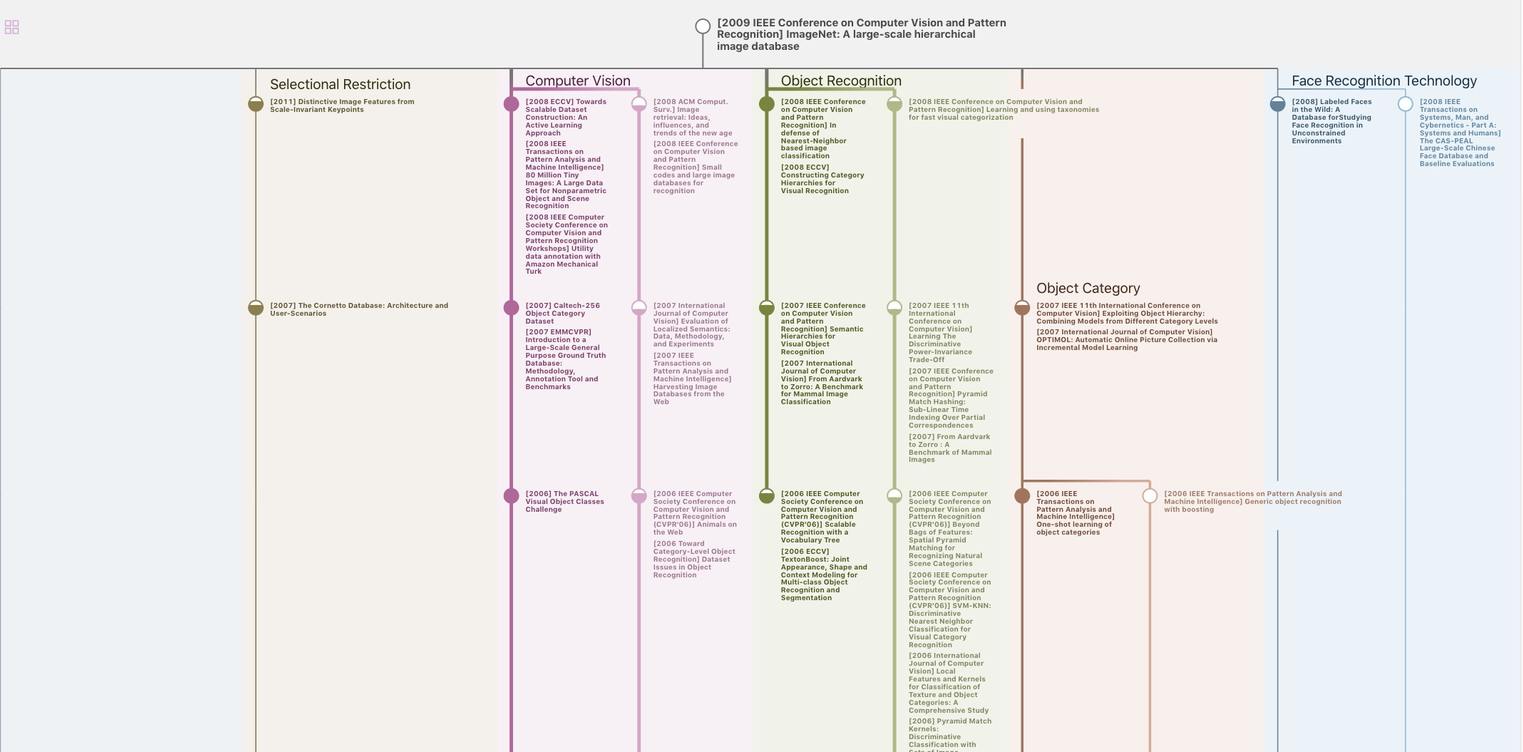
生成溯源树,研究论文发展脉络
Chat Paper
正在生成论文摘要