The use of machine learning models to predict PFS and OS outcomes from waterfall plots in randomized clinical trials (MAP-OUTCOMES).
JCO global oncology(2023)
摘要
107 Background: Depth of tumor response (DepOR) of individual patients, as visualized by waterfall plots, is an emerging short-term endpoint that may represent a surrogate for survival-based outcomes such as PFS and OS. We hypothesize that the configuration of waterfall plots in randomized trials may predict PFS/OS outcomes. Methods: A literature-based search was performed for all phase II/III randomized clinical trials published in MEDLINE from 2010 to 2022 testing molecularly targeted agents (MTA) or immunotherapy (IO). Articles reporting at least 1 waterfall plot for each treatment arm depicting maximum DepOR of target lesions with corresponding PFS/OS Kaplan-Meier plots were included. Studies are defined as positive or negative based on the achievement of a priori stated primary endpoint. Trial data collected included sample size per arm, cancer type, mechanisms of action of drug(s) tested, line of treatment, etc. Images of waterfall plots were manually extracted from publications and then processed through a semi-automatic extraction process using WebPlotDigitizer and Tesseract to produce tabular representations. Logistic regression with L2 regularization was used for modeling; hyperparameter tuning was accomplished with five-fold cross-validation on a training set compromising 80% of the data. Results: A total of 111 studies were identified: 65 (59%) phase III and 46 (41%) phase II, mean sample size per arm 317 (19-1581). Most frequent cancer type was gastrointestinal 24 (22%). MTA, IO and combinations were tested in 113 (51%), 35(16%) and 11 (5%) studies respectively. Chemotherapy and other treatment regimens were used in 63 (28%) trials. PFS was the primary endpoint in 62 (56%); 80 (75%) studies were positive. Of the 111 studies only 83 (75%) were retained for machine learning analysis, the remainder were excluded due to atypical formatting such as superimposed waterfall plots. Performance of the model was assessed on a test set which comprised 20% of the original dataset. Table below shows the classification metrics from modelling. Both PPV and NPV were ≥80%. Conclusions: MAP-OUTCOMES evaluated pan-cancer randomized studies with diverse therapeutic anticancer agents. It is a computational tool with the potential to predict survival-based outcomes from waterfall plots and may help with decisions regarding follow-on randomized studies. Further validation is ongoing. [Table: see text]
更多查看译文
关键词
waterfall plots,clinical trials,machine learning models,machine learning,map-outcomes
AI 理解论文
溯源树
样例
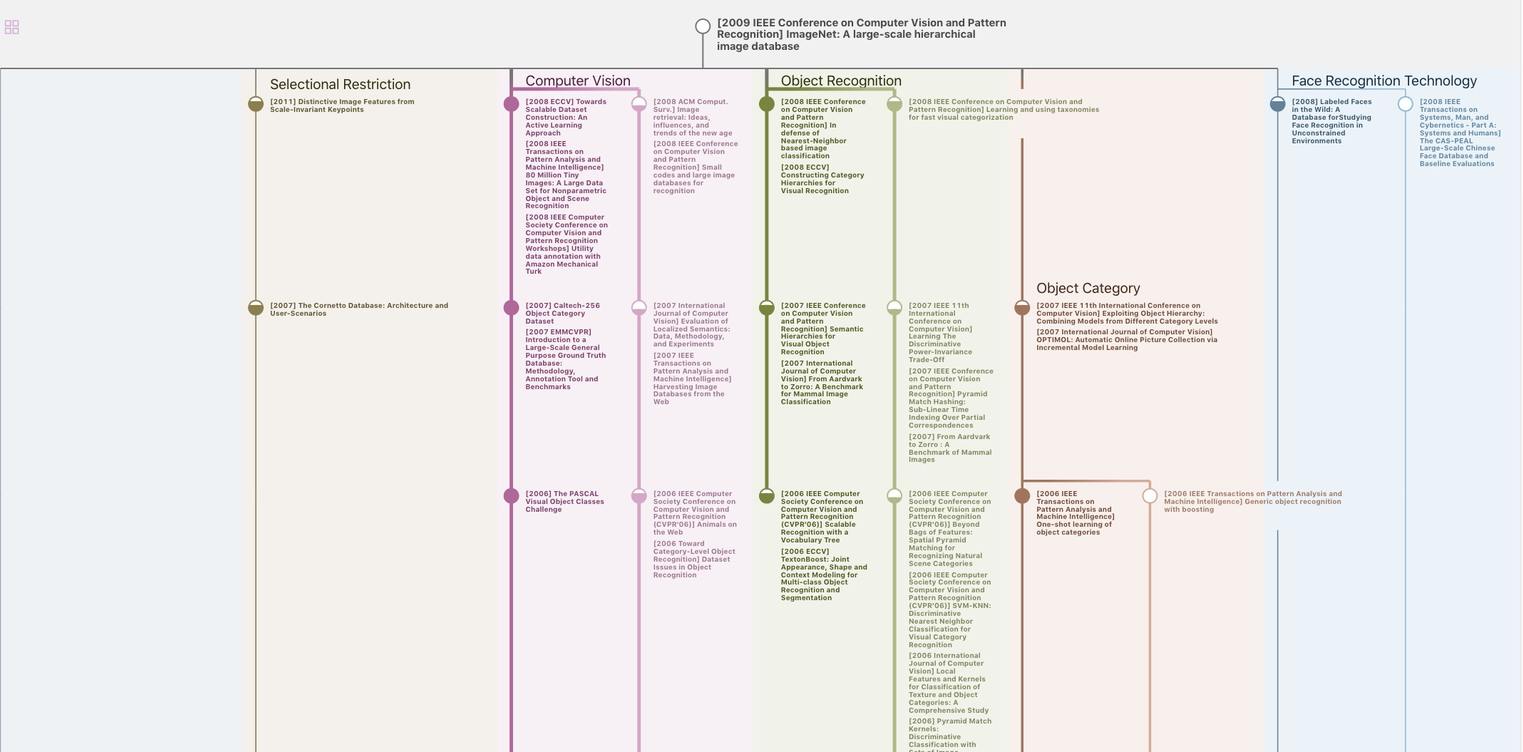
生成溯源树,研究论文发展脉络
Chat Paper
正在生成论文摘要