The importance of good practices and false hits for QSAR-driven virtual screening real application: a SARS-CoV-2 main protease (Mpro) case study
Frontiers in drug discovery(2023)
摘要
Computer-Aided Drug Design (CADD) approaches, such as those employing quantitative structure-activity relationship (QSAR) methods, are known for their ability to uncover novel data from large databases. These approaches can help alleviate the lack of biological and chemical data, but some predictions do not generate sufficient positive information to be useful for biological screenings. QSAR models are often employed to explain biological data of chemicals and to design new chemicals based on their predictions. In this review, we discuss the importance of data set size with a focus on false hits for QSAR approaches. We assess the challenges and reliability of an initial in silico strategy for the virtual screening of bioactive molecules. Lastly, we present a case study reporting a combination approach of hologram-based quantitative structure-activity relationship (HQSAR) models and random forest-based QSAR (RF-QSAR), based on the 3D structures of 25 synthetic SARS-CoV-2 M pro inhibitors, to virtually screen new compounds for potential inhibitors of enzyme activity. In this study, optimal models were selected and employed to predict M pro inhibitors from the database Brazilian Compound Library (BraCoLi). Twenty-four compounds were then assessed against SARS-CoV-2 M pro at 10 µM. At the time of this study (March 2021), the availability of varied and different M pro inhibitors that were reported definitely affected the reliability of our work. Since no hits were obtained, the data set size, parameters employed, external validations, as well as the applicability domain (AD) could be considered regarding false hits data contribution, aiming to enhance the design and discovery of new bioactive molecules.
更多查看译文
关键词
virtual screening,mpro,qsar-driven,sars-cov
AI 理解论文
溯源树
样例
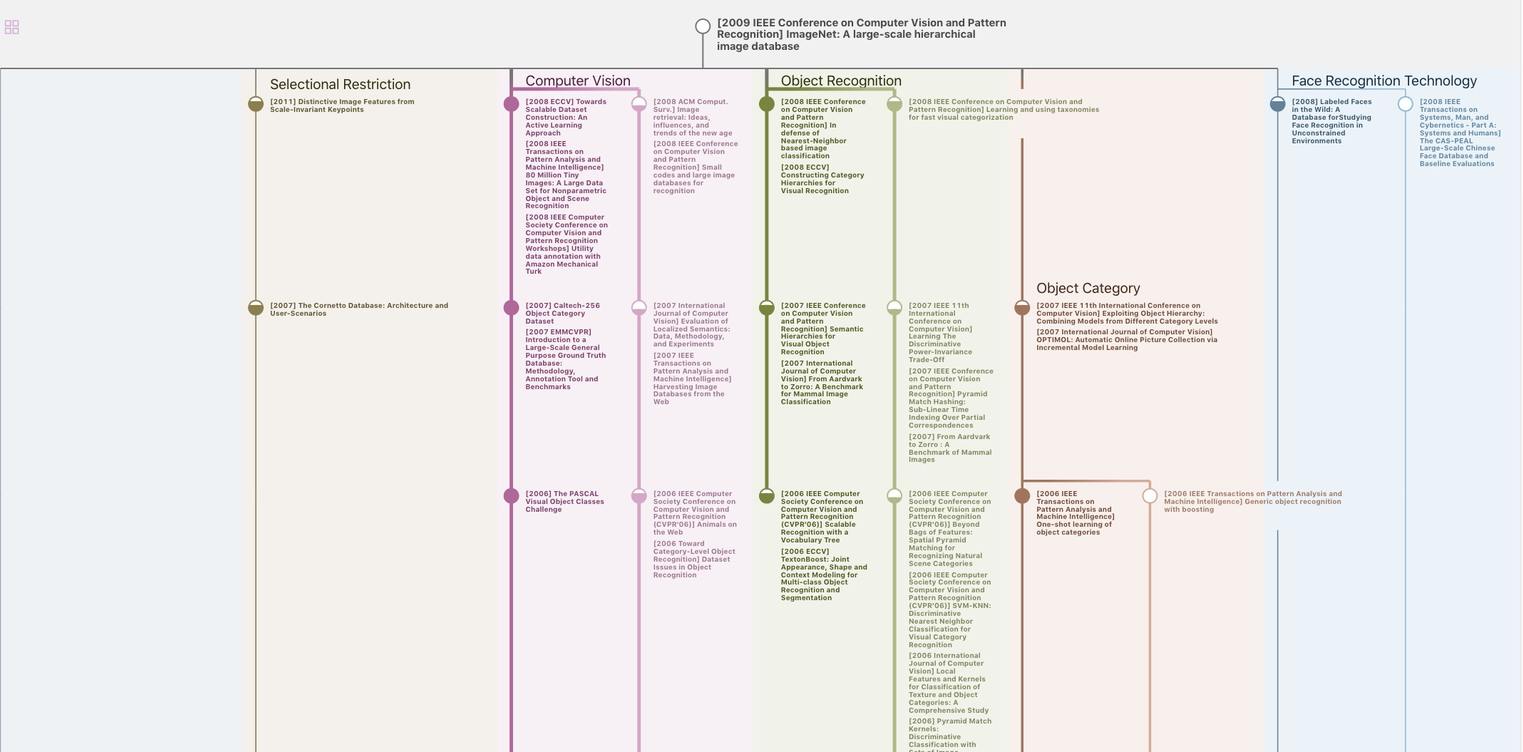
生成溯源树,研究论文发展脉络
Chat Paper
正在生成论文摘要