Deep learning reveals key aspects to help interpret the structure-property relationships of materials
Research Square (Research Square)(2023)
摘要
Abstract Deep learning (DL) models currently used for materials research have limitations in providing meaningful information for interpreting predictions and understanding the relationships between structure and material properties. To address this, we propose a DL architecture that incorporates the attention mechanism to predict material properties and gain insights into their structure-property relationships. The proposed architecture is evaluated using four datasets: the QM9 molecule dataset and three in-house-developed computational materials datasets. Train--test--split validations confirm that the models derived from the proposed DL architecture exhibit strong predictive capabilities comparable to those of current state-of-the-art models. Furthermore, comparative validations, based on first-principles calculations, indicate that the degree of attention of the atoms' local structures to the representation of the material structure is critical when interpreting structure-property relationships with respect to physical properties. The properties include molecular orbital energies or formation energies of crystals. Our proposed architecture shows great potential in accelerating material design by predicting material properties and identifying critical features of corresponding structures.
更多查看译文
关键词
materials,deep learning,structure-property
AI 理解论文
溯源树
样例
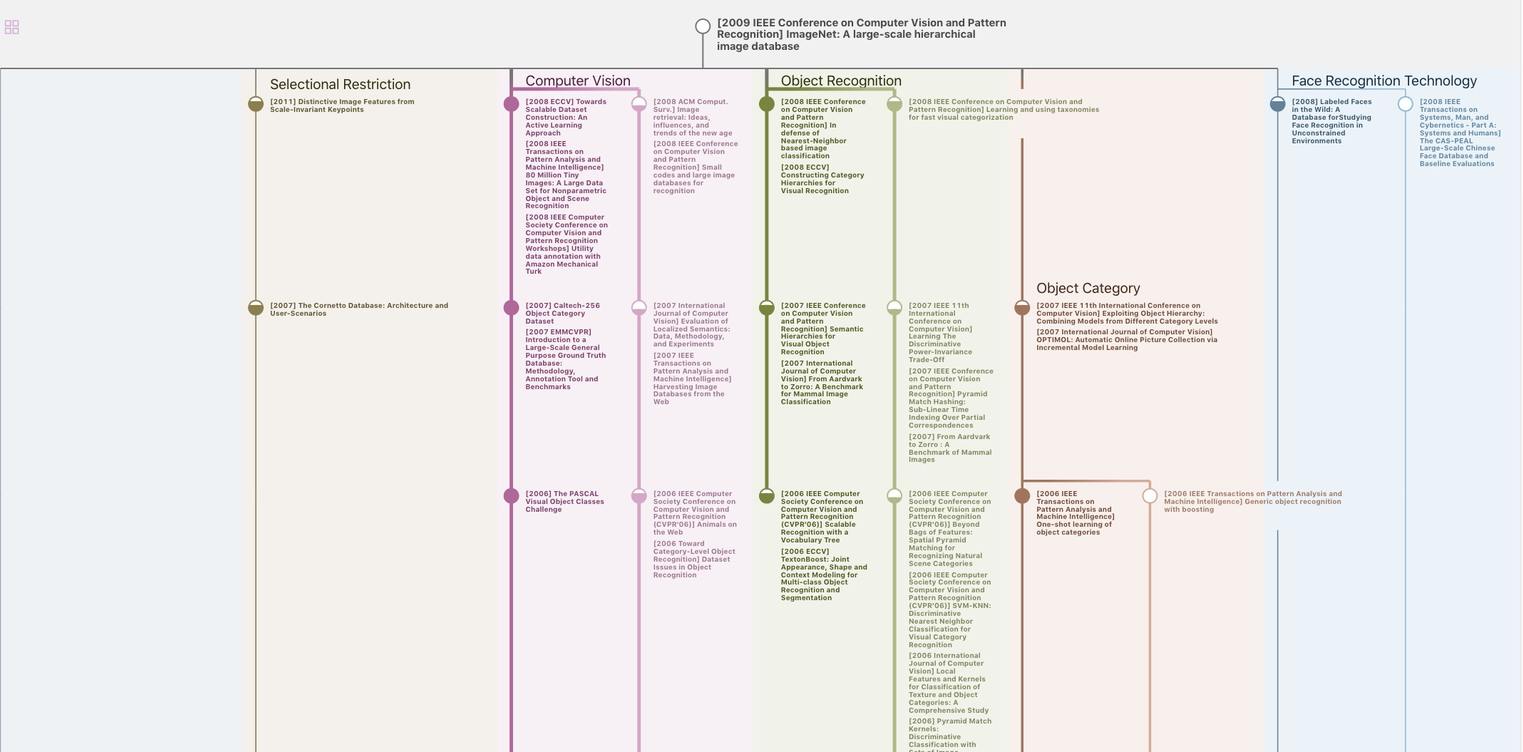
生成溯源树,研究论文发展脉络
Chat Paper
正在生成论文摘要