Iterative-in-Iterative Super-Resolution Biomedical Imaging Using One Real Image
arXiv (Cornell University)(2023)
摘要
Deep learning-based super-resolution models have the potential to revolutionize biomedical imaging and diagnoses by effectively tackling various challenges associated with early detection, personalized medicine, and clinical automation. However, the requirement of an extensive collection of high-resolution images presents limitations for widespread adoption in clinical practice. In our experiment, we proposed an approach to effectively train the deep learning-based super-resolution models using only one real image by leveraging self-generated high-resolution images. We employed a mixed metric of image screening to automatically select images with a distribution similar to ground truth, creating an incrementally curated training data set that encourages the model to generate improved images over time. After five training iterations, the proposed deep learning-based super-resolution model experienced a 7.5\% and 5.49\% improvement in structural similarity and peak-signal-to-noise ratio, respectively. Significantly, the model consistently produces visually enhanced results for training, improving its performance while preserving the characteristics of original biomedical images. These findings indicate a potential way to train a deep neural network in a self-revolution manner independent of real-world human data.
更多查看译文
关键词
imaging,real image,iterative-in-iterative,super-resolution
AI 理解论文
溯源树
样例
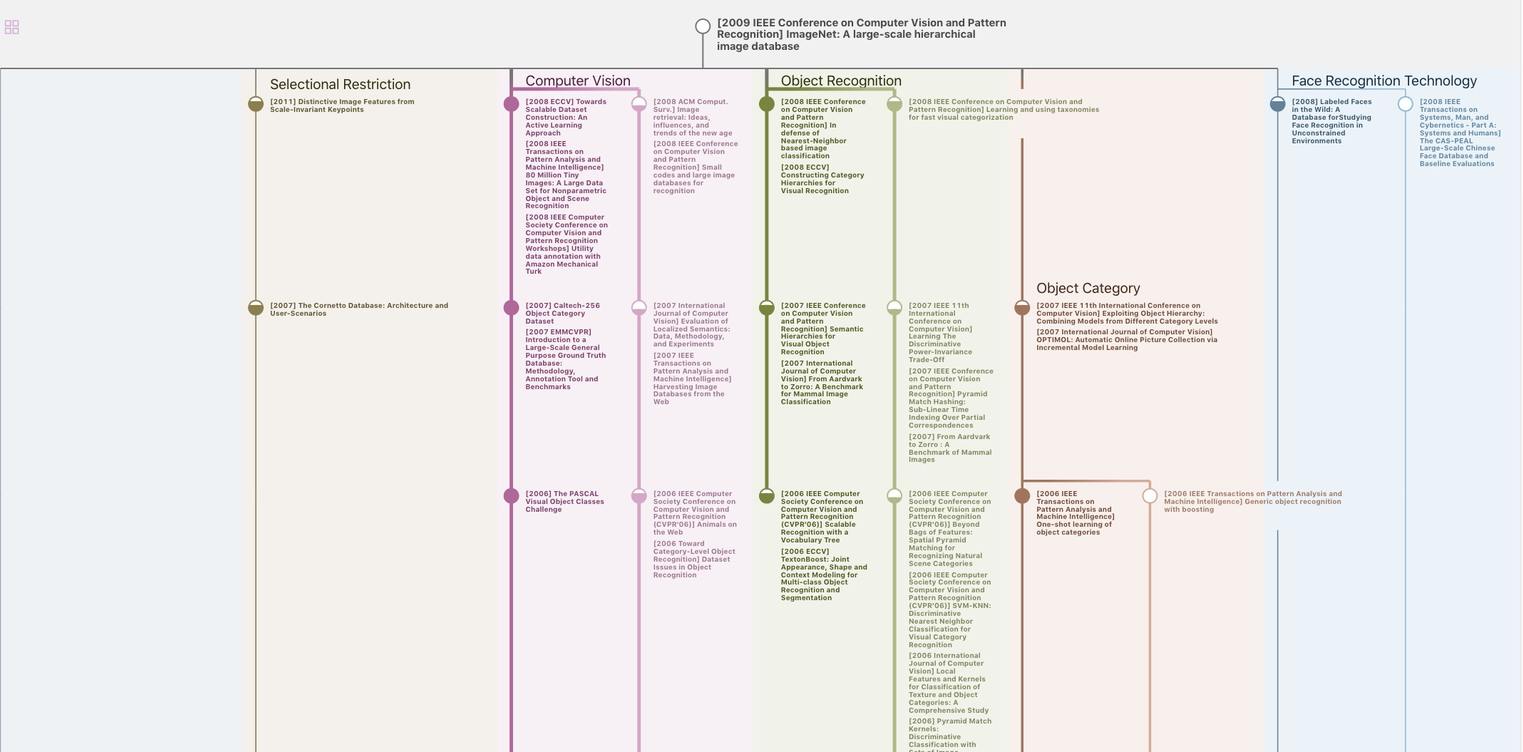
生成溯源树,研究论文发展脉络
Chat Paper
正在生成论文摘要