SGSR-Net: Structure Semantics Guided LiDAR Super-Resolution Network for Indoor LiDAR SLAM
IEEE TRANSACTIONS ON MULTIMEDIA(2024)
摘要
Multi-Beam LiDAR (MBL) sensors sample the real-world with discrete 3D point clouds (PC) and have become a major and essential 3D sensing capability for autonomous robots. To ensure an accurate point sampling on surfaces, high-resolution MBL sensors (e.g., Ouster OS0-128) are commonly used to collect dense point clouds for robot tasks, including object detection and tracking, simultaneous localization and mapping (SLAM), in applications such as autonomous driving vehicles (ADVs). However, the high cost and large volume/weight/energy consumption of such sensors limit their usage in broader applications such as UAV/UGV swarms with small-scale agents with limited payload. Existing studies on Super-Resolution (SR) upsampling of the PC from low-resolution MBL have not considered the geometry semantics of the scenes, thus resulting in less optimal SR points for downstream subtasks (e.g., SLAM). Thus, this article proposes SGSR-Net, a structure semantics-guided MBL Super-Resolution network. SGSR-Net takes the low-resolution range images of the MBL sensors as input and produces dense and structure-aware Super-Resolution point cloud from those sparse measurements through a vertical spatial and channel attention-enhanced CNN model coupling with guided Monte Carlo filtering, for indoor LiDAR-SLAM applications. The SGSR-Net is validated using datasets collected by a UGV equipped with multiple MBL sensors. The results demonstrate that the proposed CG-LSR (CASE Attention Guided Encoder-Decoder LiDAR Super-Resolution Network) reduces the MAE of the SR points by 12.4% down to 0.177 m when compared with the state-of-the-art (SOTA) method Shan et al. (2020), Ren et al. (2021), Kwon et al. (2022), Long and Wang (2022). The indoor SLAM results with SR-points produced by SGSR-Net show that the mean and RMSE of the absolute pose error (APE) are decreased by 27% and 30%, down to 0.849 m and 0.902 m, respectively, which significantly improve the indoor-SLAM performance and stability of SOTA LiDAR-SLAM systems (i.e. LeGO-LOAM Shan and Englot (2018), Dellenbach et al. (2022), Vizzo et al. (2023), Zhang and Singh (2014)).
更多查看译文
关键词
Point cloud compression,Laser radar,Superresolution,Sensors,Simultaneous localization and mapping,Three-dimensional displays,Task analysis,Point cloud,Super-Resolution,SLAM,LiDAR
AI 理解论文
溯源树
样例
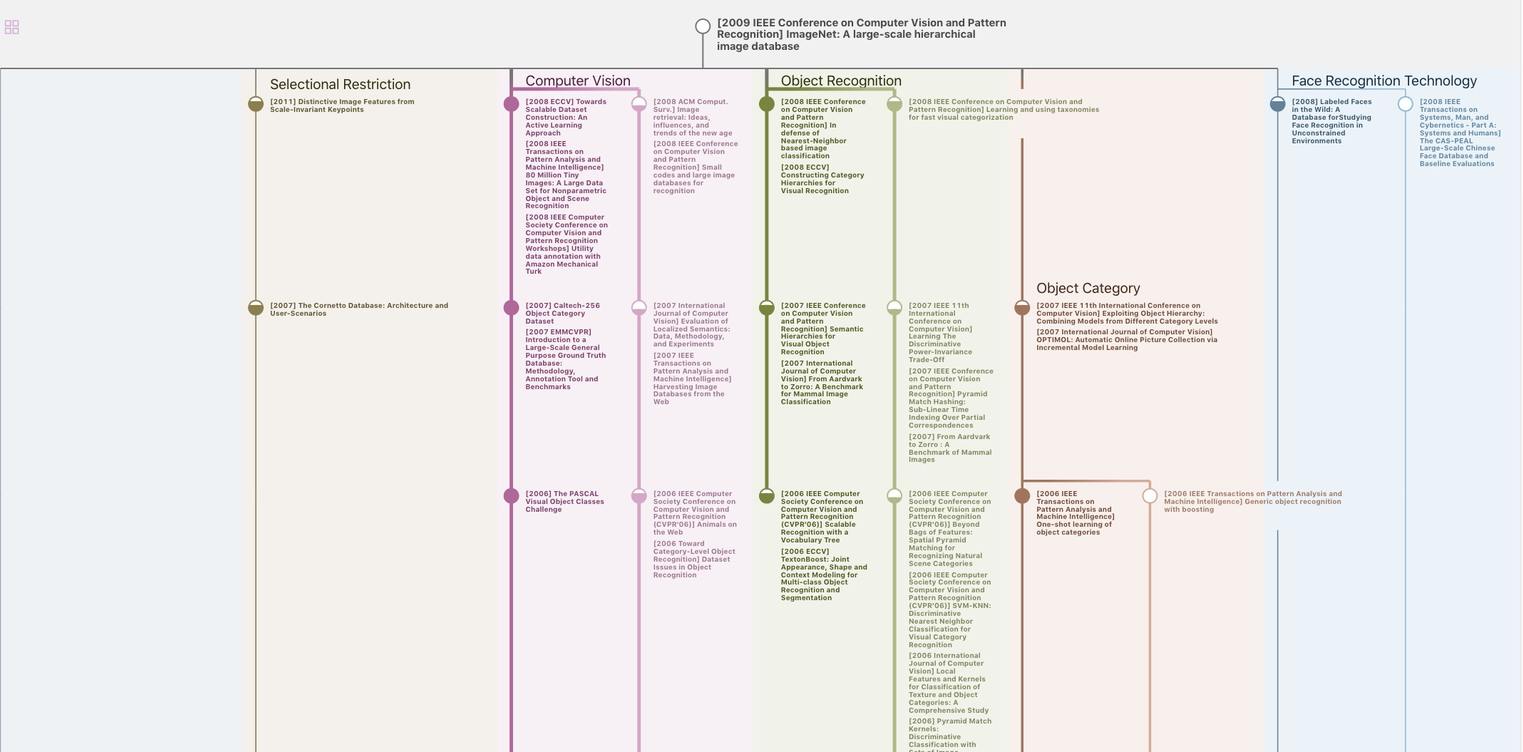
生成溯源树,研究论文发展脉络
Chat Paper
正在生成论文摘要