Energy-Motion Features Aggregation Network for Players- Fine-Grained Action Analysis in Soccer Videos
IEEE TRANSACTIONS ON CIRCUITS AND SYSTEMS FOR VIDEO TECHNOLOGY(2024)
摘要
Rich and complex events in sports have led to the development of a wide-variety of techniques for interpreting content of sports videos in terms of players' actions, poses, gait, performance, etc. This is due to the requirements from coaches, trainers and players who expect to analyze actions in top sports events, as well as sports fans who practice to imitate professional playing skills, e.g., dribbling, shooting, etc. However, this poses two key challenges for automated sports analysis community. Firstly, there are extremely limited public sports datasets. Secondly, recent advances in interpretations of sports activities, e.g., soccer, are predominantly made through analyzing coarse-grained contents. Players' fine-grained skills analysis still remains under-explored. To alleviate these problems, this paper (a) collects the dataset of highlight videos of soccer players, including two coarse-grained action types of soccer players and six fine-grained actions of players. Detailed annotations are provided for the collected dataset, in terms of action classes, bounding boxes, segmentation maps, and body keypoints of soccer players, and positions of a soccer ball in a game. (b) leverages the understanding of complex highlight videos by proposing an energy-motion features aggregation network-EMANet to fully exploit energy-based representation of soccer players movements in video sequences and explicit motion dynamics of soccer players in videos for soccer players' fine-grained action analysis. Experimental results and ablation studies validate the proposed approach in recognizing soccer players actions using the collected soccer highlight video datasets.
更多查看译文
关键词
Dribbling and shooting,player action analysis,soccer action analysis,sports analytics
AI 理解论文
溯源树
样例
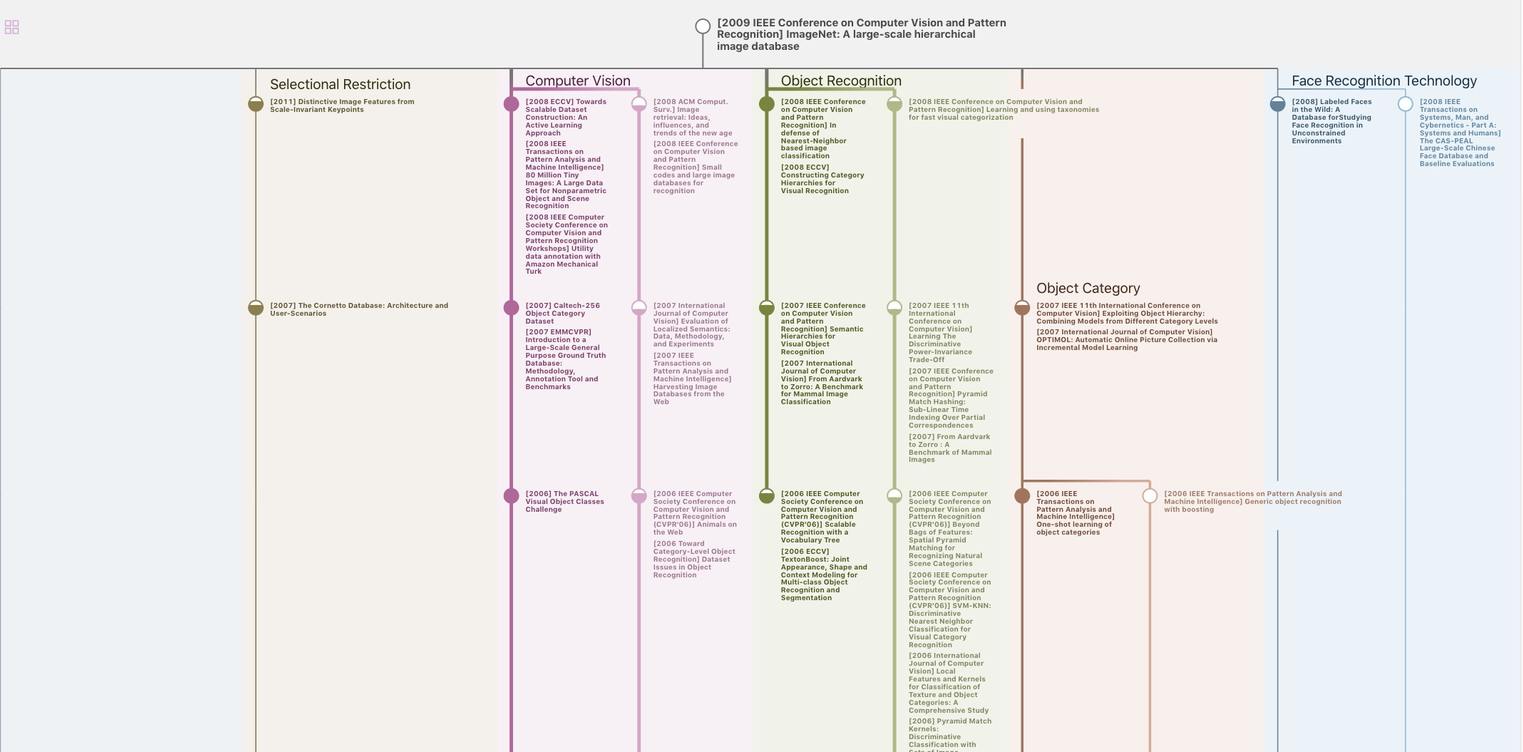
生成溯源树,研究论文发展脉络
Chat Paper
正在生成论文摘要