Decentralized Asynchronous Non-convex Stochastic Optimization on Directed Graphs
IEEE Transactions on Control of Network Systems(2023)
摘要
We consider a decentralized stochastic optimization problem over a network of agents, modeled as a directed graph: Agents aim to asynchronously minimize the average of their individual losses (possibly non-convex), each one having access only to a noisy estimate of the gradient of its own function. We propose an asynchronous distributed algorithm for such a class of problems. The algorithm combines stochastic gradients with tracking in an asynchronous push-sum framework and obtains a sublinear convergence rate, matching the rate of the centralized stochastic gradient descent applied to the nonconvex minimization. Our experiments on a non-convex image classification task using convolutional neural network validate the convergence of our proposed algorithm across different number of nodes and graph connectivity percentages.
更多查看译文
关键词
stochastic,optimization,graphs,non-convex
AI 理解论文
溯源树
样例
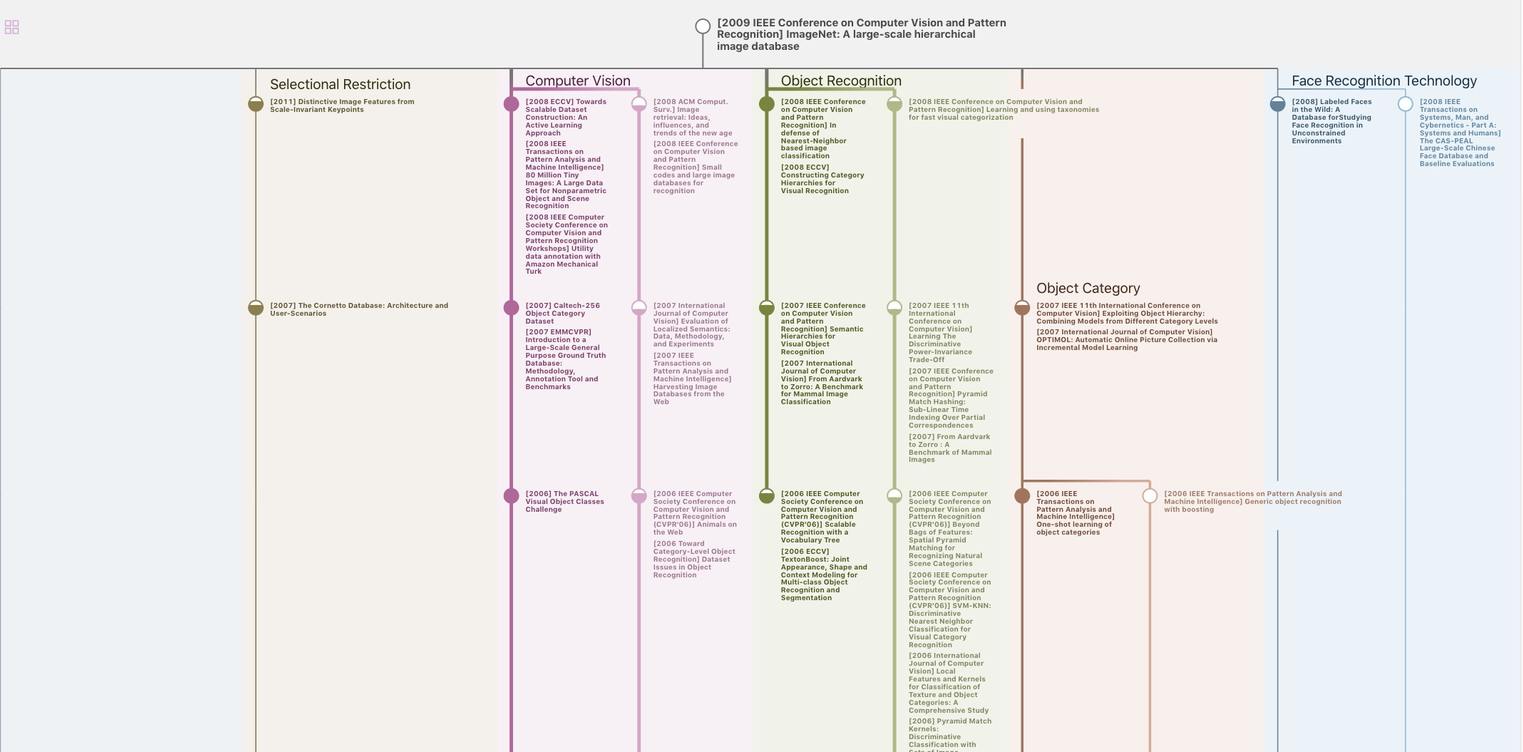
生成溯源树,研究论文发展脉络
Chat Paper
正在生成论文摘要