A Multi-stage Deep Learning Network for Trace Explosive Residues Detection in SERS Chips
IEEE Sensors Journal(2023)
摘要
To address the challenges of relying on specialized personnel and incurring significant time costs in qualitative and quantitative analysis using SERS technology for explosive residue detection, this paper proposes a detection method for explosive residues based on a multi-stage deep learning network and SERS chip. To improve the qualitative analysis performance of the SERS spectrum, a novel FAB-ResNet is constructed through the integration of a modified attention mechanism into the ResNet network. Additionally, for proper processing of long sequential data, the NLSTM network is selected for quantitative analysis with its powerful global information aggregating capability. Consequently, the NLSTM is incorporated into FAB-ResNet to construct a multi-stage hybrid network. Extensive experiments are carried out to prove the effectiveness of the proposed hybrid network. The qualitative results demonstrated the superiority of the proposed FAB-ResNet with its outstanding classification accuracy (100%). Meanwhile, by comparing quantitative results, the NLSTM network provides promising performance ( R 2 = 0.9835, RMSE = 0.1653, MAE = 0.0916, and MARE = 2.7488%). Furthermore, the comparative results among other state-of-the-art networks confirmed the effectiveness of the proposed method as a means of explosive residue detection and analysis, which shows the potential for further application of SERS technology in explosive site detection.
更多查看译文
关键词
trace explosive residues detection,sers chips,deep learning network,deep learning,multi-stage
AI 理解论文
溯源树
样例
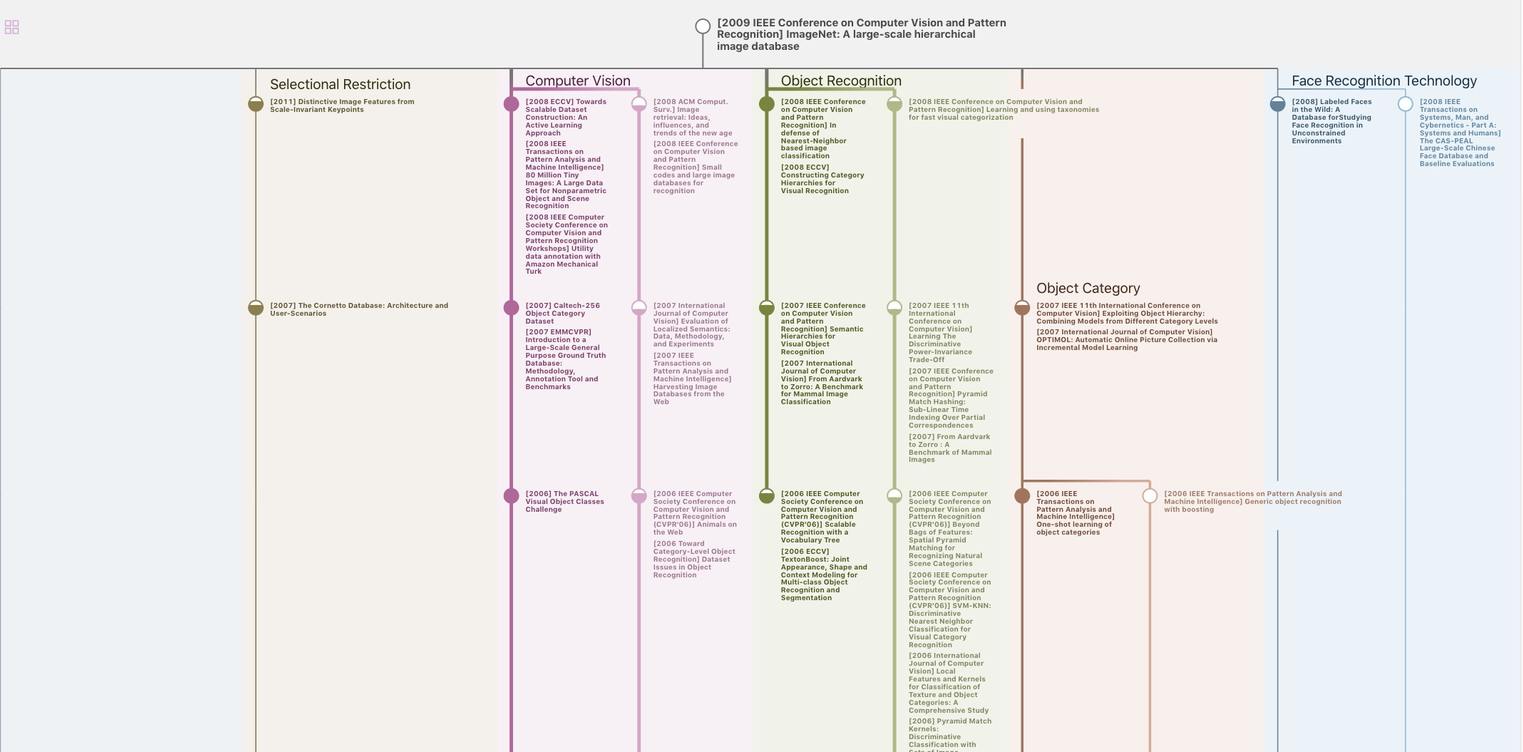
生成溯源树,研究论文发展脉络
Chat Paper
正在生成论文摘要