A Computational Model for Storing and Embedding Data in Dynamically Evolving Network Synaptic Structures
Research Square (Research Square)(2023)
摘要
Abstract Spike-timing dependent plasticity (STDP) is widely accepted as a mechanism through which the brain can learn information. Basing synaptic changes on the timing between spikes enhances contributing edges within a network. While STDP rules control the evolution of networks, most research focuses on spiking rates or specific activation paths when evaluating learned information. While imaging studies demonstrate physical changes to synapses due to STDP, these changes have not been interrogated based on their embedding capacity of a stimulus .Here, we show that networks with biological features can embed stimulus information into their synaptic weights. We use a k-nearest neighbor algorithm on the synaptic weights of independent networks to identify their stimulus with high accuracy based on local neighborhoods.While spike rates and timings remain useful, structural embeddings represent a new way to integrate information within a biological network. Our results demonstrate that there may be value in observing these changes directly.Beyond computational applications, this analysis may also inform investigation into neuroscience.Research is underway on the potential of astrocytes to integrate synapses in the brain and communicate that information elsewhere.In addition, observations of these synaptic embeddings may lead to novel therapies for memory disorders, such as transient epileptic amnesia.
更多查看译文
关键词
computational model,structures,network
AI 理解论文
溯源树
样例
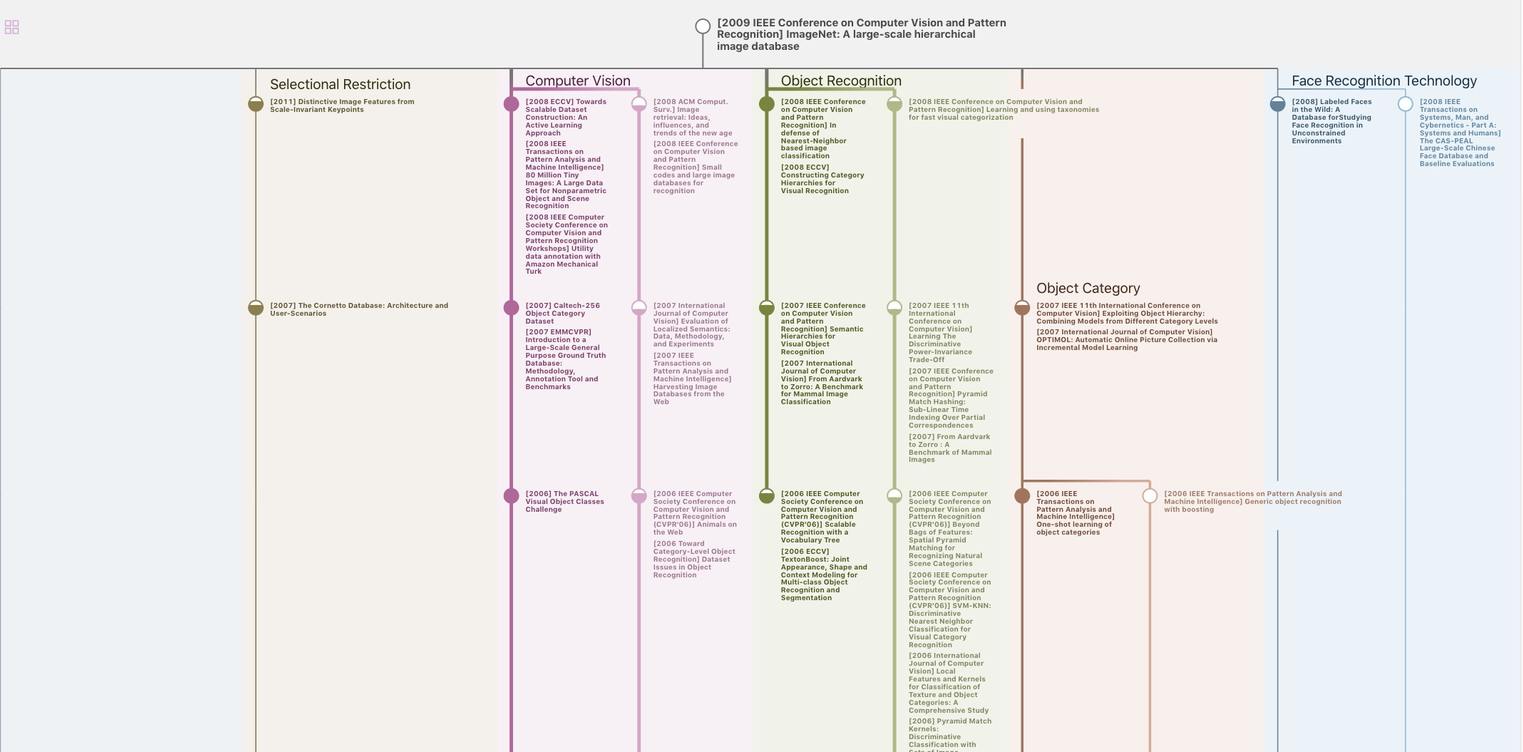
生成溯源树,研究论文发展脉络
Chat Paper
正在生成论文摘要