Privacy-Preserving Queries Using Multisource Private Data Counting on Real Numbers in IoT
IEEE INTERNET OF THINGS JOURNAL(2024)
摘要
In this article, our primary focus is on the current lack of privacy-preserving queries tailored to real-number fields rather than integers. We take multisource private data counting on real numbers (R-PDC) within IoT architecture as a breakthrough point to enable diverse query services without revealing sensitive data. The advantage of this is that the parameters required for queries remain stable and minimal in the scenario of wide numerical domain and dynamic changed data set. First, we present a general R-PDC method based on curve approximation, in which an approximate query function (AQF) is established to approximate the constructed ideal counting curve for element queries on target set. We also demonstrate curve construction process of AQF and provide the feasibility theorem of AQF for counting a target element within an allowable error. By integrating the R-PDC method with fixed-point fully homomorphic encryption, an efficient R-PDC scheme is presented to perform multiparty collaborative queries in IoT. In security aspect, the R-PDC scheme on (m,e) -AQF is proved to be statistically secure against chosen element attack (CEA) for cumulative error is an element of and data set size m . Moreover, the scheme achieves O(nm) computation and O(n(2)gamma) communication complexities for n servers and gamma-length fraction. Finally, as an extension of AQF over single attribute, multidimensional R-PDC method is applied into privacy-preserving Naive Bayes classifier and Apriori algorithm over multiple attributes. Our work provides substantial support and insights for the advancement of privacy computation.
更多查看译文
关键词
Approximate query function (AQF),curve approximation,fixed-point presentation,IoT,multisource private data counting (PDC),privacy-preserving query
AI 理解论文
溯源树
样例
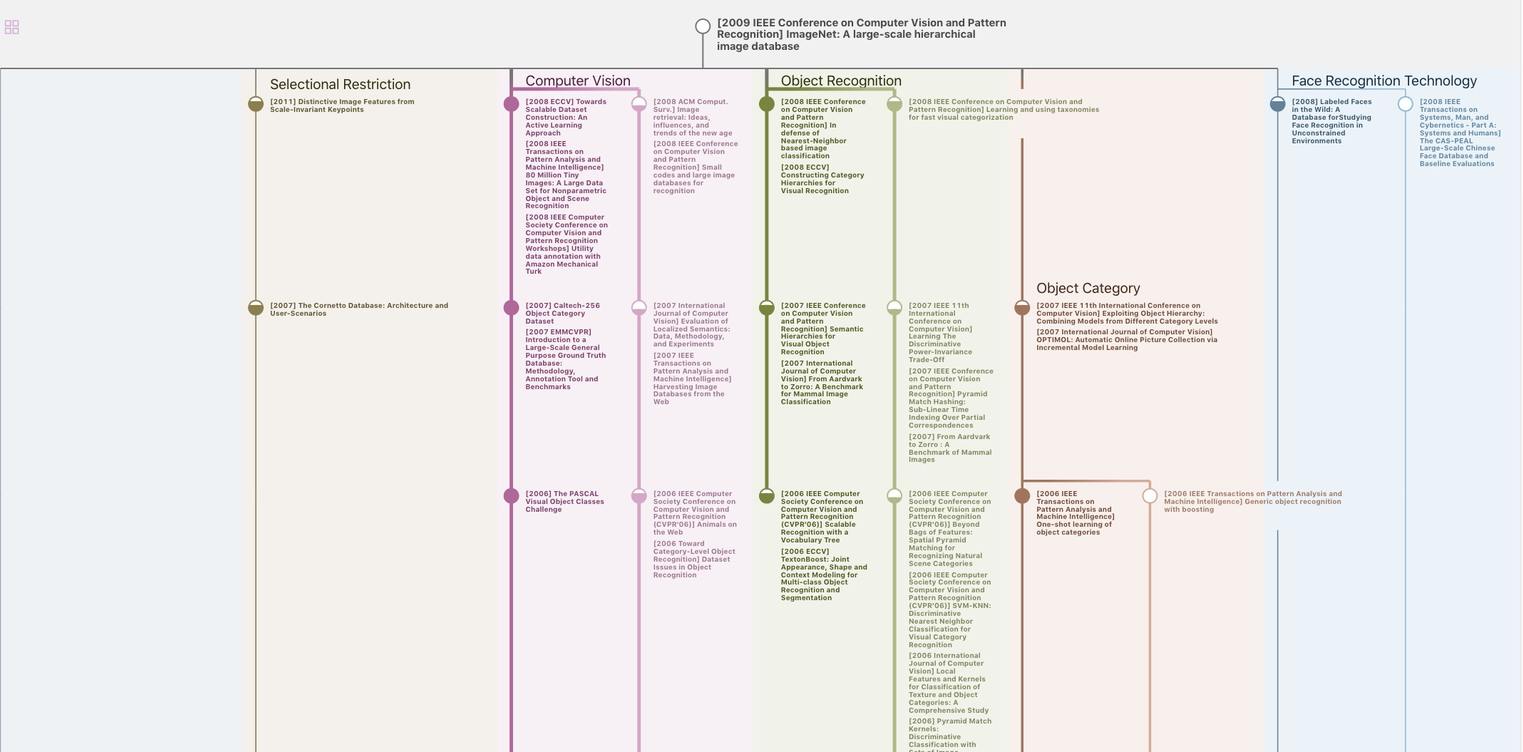
生成溯源树,研究论文发展脉络
Chat Paper
正在生成论文摘要