EPM-Net: Efficient Feature Extraction, Point-Pair Feature Matching for Robust 6-D Pose Estimation
IEEE TRANSACTIONS ON MULTIMEDIA(2024)
摘要
Estimating the 6-D poses of objects from RGB-D images holds great potential for several applications. However, given that the 6-D pose estimation accuracy is significantly affected by occlusion and noise between the objects in an image, this paper proposes a novel 6-D pose estimation method based on Efficient feature extraction and Point-pair feature matching. Specifically, we develop the Efficient channel attention Convolutional Neural Network (ECNN) and SO(3)-Encoder modules to extract 2-D features from the RGB image and SO(3)-equivariant features from the depth image, respectively. These features are fused in the DenseFusion module to obtain 3-D features in the camera space. Meanwhile, we exploit CAD model priors to obtain 3-D features in the model space through the model feature encoder, and then we globally regress the 3-D features in the camera and model space. According to these features, we generate oriented point clouds in each space, and then conduct point-pair feature matching to obtain pose information. Finally, we perform direct pose regression on the 3-D features in the camera and model space, and then resulting point-pair feature matching pose information is combined with the direct point-wise pose regression information to enhance pose prediction accuracy. Experimental results on three widely used benchmarking datasets demonstrate that our method achieves state-of-the-art performance, particularly for severe occluded scenes.
更多查看译文
关键词
6-D pose estimation,feature extraction,point-pair features (PPF),RGB-D images
AI 理解论文
溯源树
样例
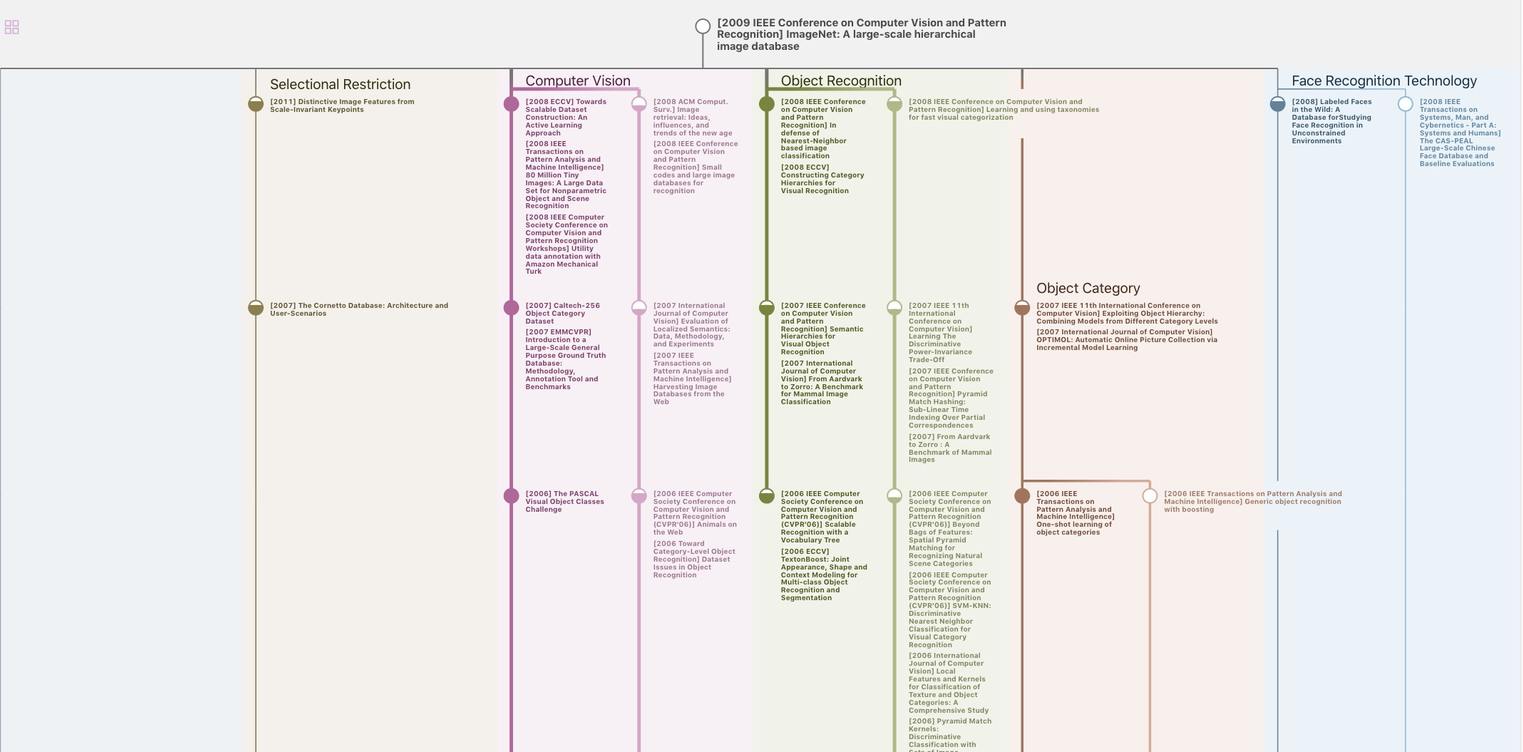
生成溯源树,研究论文发展脉络
Chat Paper
正在生成论文摘要