Decentralized Over-the-Air Federated Learning by Second-Order Optimization Method
IEEE Transactions on Wireless Communications(2023)
摘要
Federated learning (FL) is an emerging technique that enables privacy-preserving distributed learning. Most related works focus on centralized FL, which leverages the coordination of a parameter server to implement local model aggregation. However, this scheme heavily relies on the parameter server, which could cause scalability, communication, and reliability issues. To tackle these problems, decentralized FL, where information is shared through gossip, starts to attract attention. Nevertheless, current research mainly relies on first-order optimization methods that have a relatively slow convergence rate, which leads to excessive communication rounds in wireless networks. To design communication-efficient decentralized FL, we propose a novel over-the-air decentralized second-order federated algorithm. Benefiting from the fast convergence rate of the second-order method, total communication rounds are significantly reduced. Meanwhile, owing to the low-latency model aggregation enabled by over-the-air computation, the communication overheads in each round can also be greatly decreased. The convergence behavior of our approach is then analyzed. The result reveals an error term, which involves a cumulative noise effect, in each iteration. To mitigate the impact of this error term, we conduct system optimization from the perspective of the accumulative term and the individual term, respectively. Numerical experiments demonstrate the superiority of our proposed approach and the effectiveness of system optimization.
更多查看译文
关键词
Decentralized federated learning,over-the-air computation,second-order optimization method
AI 理解论文
溯源树
样例
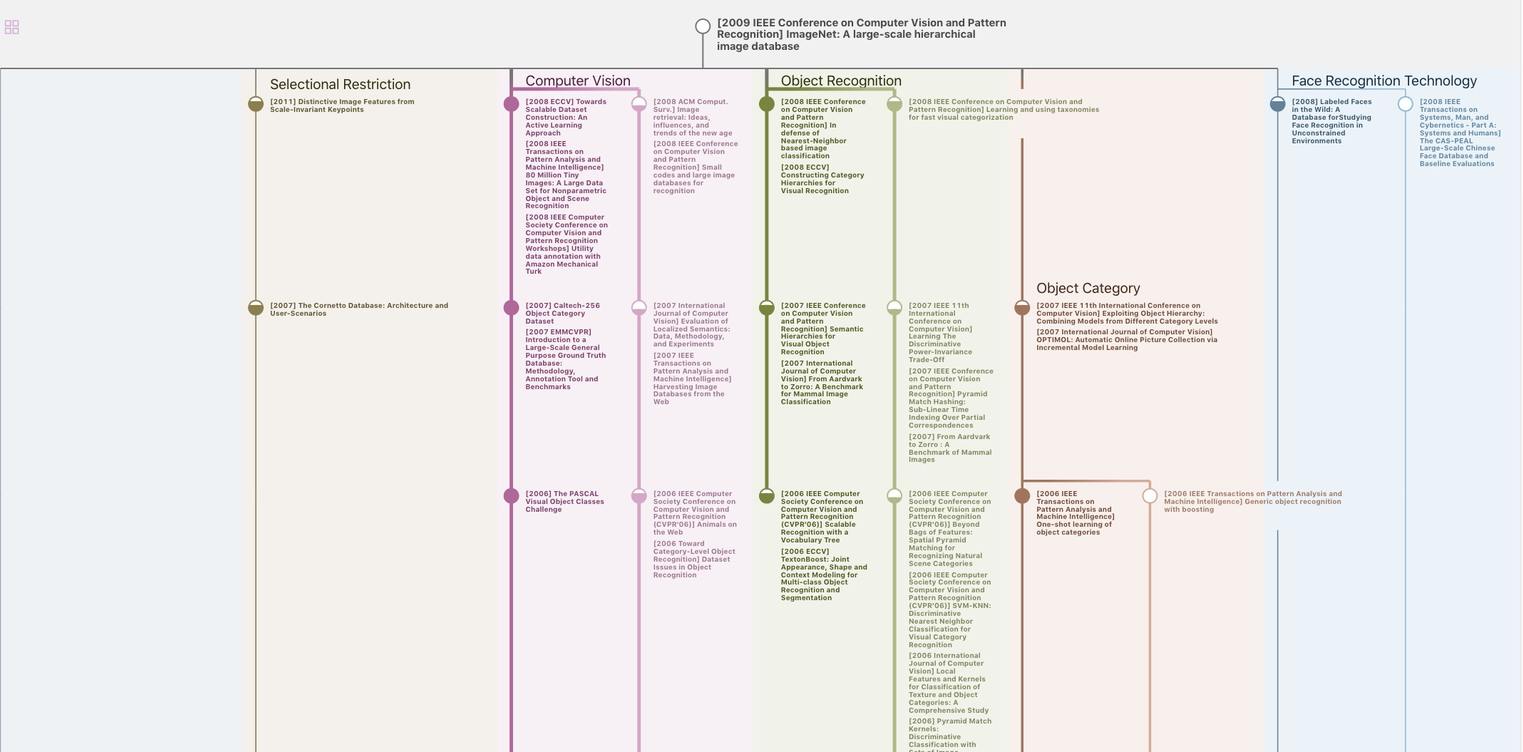
生成溯源树,研究论文发展脉络
Chat Paper
正在生成论文摘要