Day-Ahead PV Power Forecasting Model Based on Fine-Grained Temporal Attention and Cloud-Coverage Spatial Attention
IEEE TRANSACTIONS ON SUSTAINABLE ENERGY(2024)
摘要
With the increase of grid-connected photovoltaic (PV) power plants, measuring the uncertainty of PV output forecast due to multiple meteorological factors has become an important method to ensure the stable operation of the power system. However, the manual similar days' extraction methods of historical power features and cloud motion features, which are commonly used for PV spatial-temporal prediction, are inefficient and coarse-grained. In this paper, focusing on automatic attention of the local similar sequences in daily features, we improve the attention scoring method of the original transformer to increase the calculation accuracy of the similarity of PV power sequences. We construct an end-to-end PV power prediction framework using an encoder-decoder architecture. Based on the spatial-temporal similarity characteristics of PV output, the fine-grained temporal and cloud-covered spatial attentions embedded in the prediction framework are proposed using the improved similar sequence attention module. Among them, fine-grained temporal attention can dynamically position and utilize similar historical PV power sequences for the predicted period; cloud-coverage spatial attention automatically captures the neighbor site's matching PV power sequences. Experiments were conducted on a real PV dataset, and the root mean square error was reduced by 21.34% on average compared to the baseline model. The results proved the effectiveness of the proposed model.
更多查看译文
关键词
Cloud-coverage spatial attention,day-ahead PV power forecasting,fine-grained temporal attention,similar sequence attention score
AI 理解论文
溯源树
样例
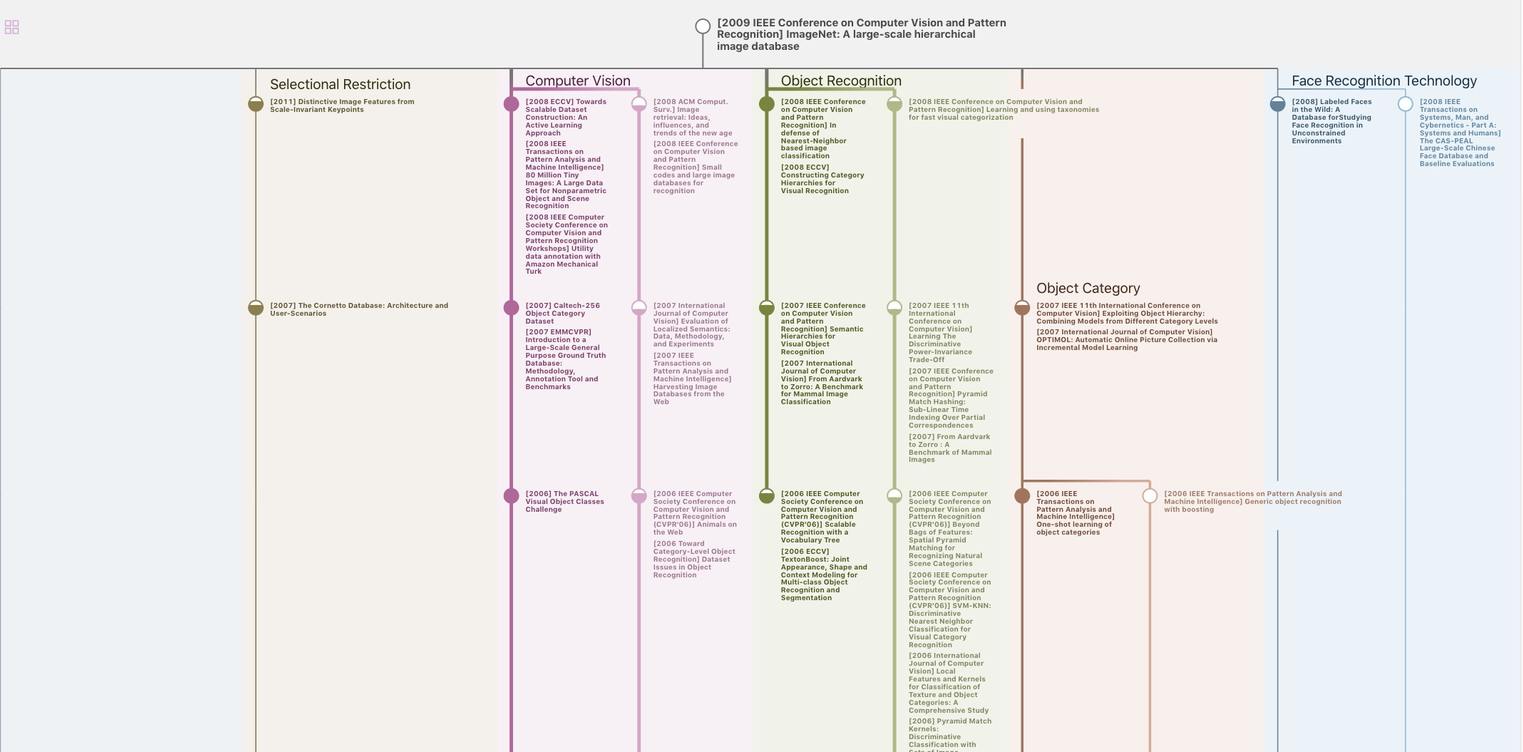
生成溯源树,研究论文发展脉络
Chat Paper
正在生成论文摘要