Sustainable Intrusion Detection in Vehicular Controller Area Networks using Machine Intelligence Paradigm
Sustainable Machine Intelligence Journal(2023)
摘要
The advent of smart mobility and the proliferation of connected vehicles have introduced new challenges in securing Vehicular Controller Area Networks (CANs) against cyber threats. This paper proposes an innovative machine intelligence paradigm for sustainable intrusion detection within vehicular networks. We present a Deep Neural Network (DNN) model that effectively classifies CAN traffic into categories, including Normal, Denial of Service (DoS), Gear Attack (Spoofing), RPM Attack (Spoofing), and Fuzzy Attack. The DNN's architecture is designed to learn and adapt to the dynamic nature of vehicular communications, enhancing its ability to detect network intrusions. The study encompasses an inclusive exploration of the CAN bus architecture, message data format, and related security vulnerabilities to provide a solid foundation for intrusion detection. Our methodology employs mathematical representations of the DNN model, offering insight into its training process. Visualizations of results, such as confusion matrices, ROC-AUC curves, T-SNE plots, and SHAP explanations, provide a holistic view of the model's performance and offer valuable insights for system refinement. By bridging the gap between machine intelligence and vehicular security, this research contributes to the ongoing efforts to fortify critical infrastructure, ensuring the reliability and sustainability of vehicular networks in the era of connected and autonomous vehicles.
更多查看译文
关键词
vehicular controller area networks,machine intelligence paradigm
AI 理解论文
溯源树
样例
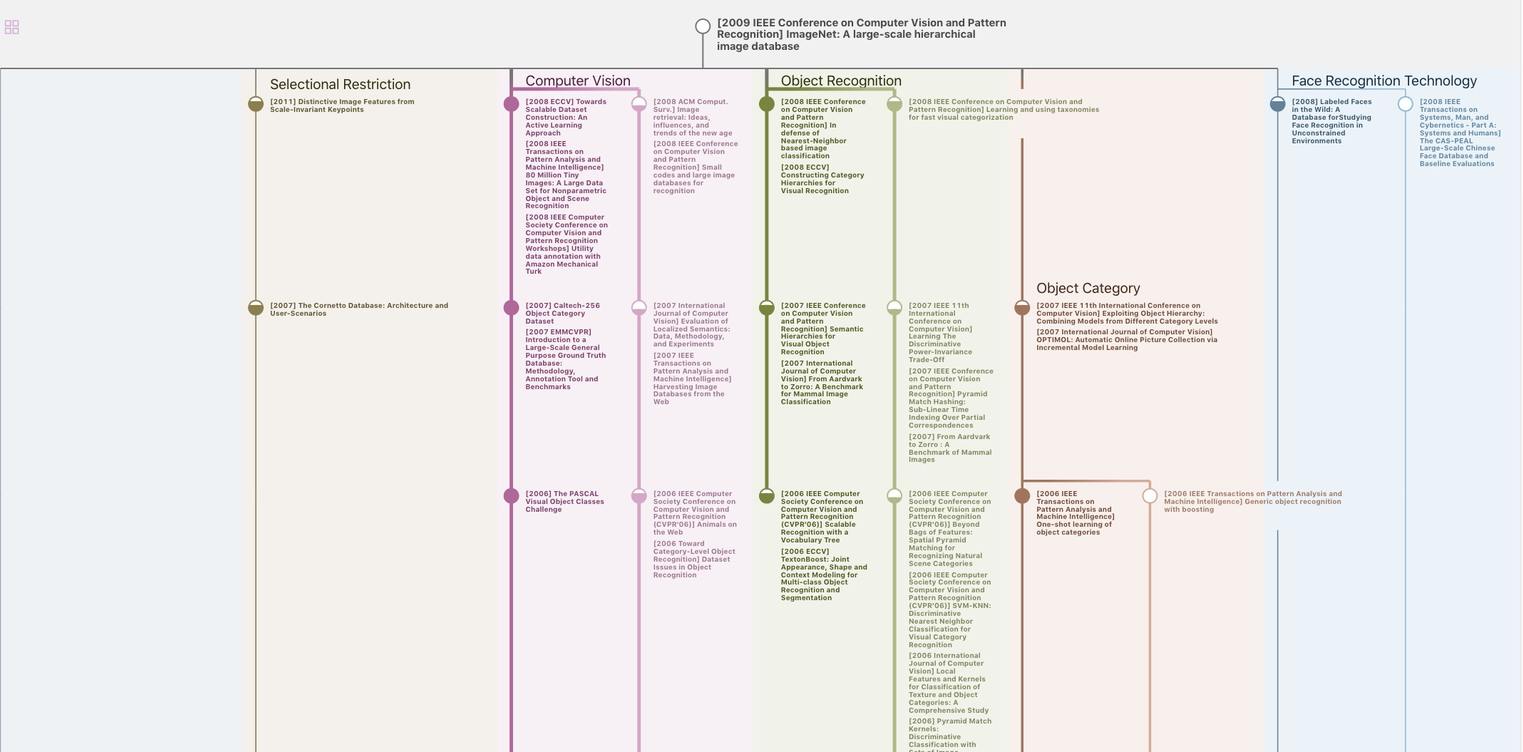
生成溯源树,研究论文发展脉络
Chat Paper
正在生成论文摘要