Assessment of prognostic model performance in the presence of competing risks using a cause-specific hazards approach
Research Square (Research Square)(2023)
摘要
Abstract Background: Calibration is assessed on absolute risks to determine the agreement between predicted risks from the model and the observed risks. For competing risks data, correct specification of more than one model may be required to ensure well-calibrated predicted risks for the event of interest. Furthermore, interest may be in the predicted risks of the event of interest, competing events and all-causes. Therefore, calibration must be assessed simultaneously using various measures. Methods: In this paper, we focus on the development of prediction models using the cause-specific hazards approach. We propose that miscalibration for cause-specific hazards models is assessed using net probabilities of each event alongside the assessment of calibration of the cause-specific cumulative incidence functions. We simulated a range of scenarios to illustrate how to identify which model(s) is mis-specified, both in an internal and external validation setting. Calibration plots are presented alongside performance measures such as the Brier Score and Index of Prediction Accuracy. We propose using pseudo-values to calculate observed risks and we generate a smooth calibration curve with restricted cubic splines. We fitted flexible parametric survival models to the simulated data in order to flexibly estimate baseline cause-specific hazards for prediction of individual risk. Results: Our simulations illustrate that miscalibration due to a mis-specified covariate functional form in the prediction model, or changes in the baseline cause-specific hazards in external validation data are better identified using net probabilities of death. A mis-calibrated model on one cause, could lead to poor calibration on predicted risks for each cause of interest, including the all-cause absolute risk. This is because prediction of a single cause-specific absolute risk is impacted by effects of variables on the cause of interest and competing events. Conclusions: If accurate predictions for both all-cause and each cause-specific absolute risk are of interest, this is best achieved by developing and validating models via the cause-specific hazards approach. For each cause-specific model, researchers should evaluate calibration plots on net probabilities for each event to reveal the cause of any miscalibration. Pseudo-values are also proposed as a means to obtain observed individual risk and smoothed calibration curves.
更多查看译文
关键词
prognostic model performance,risks,cause-specific
AI 理解论文
溯源树
样例
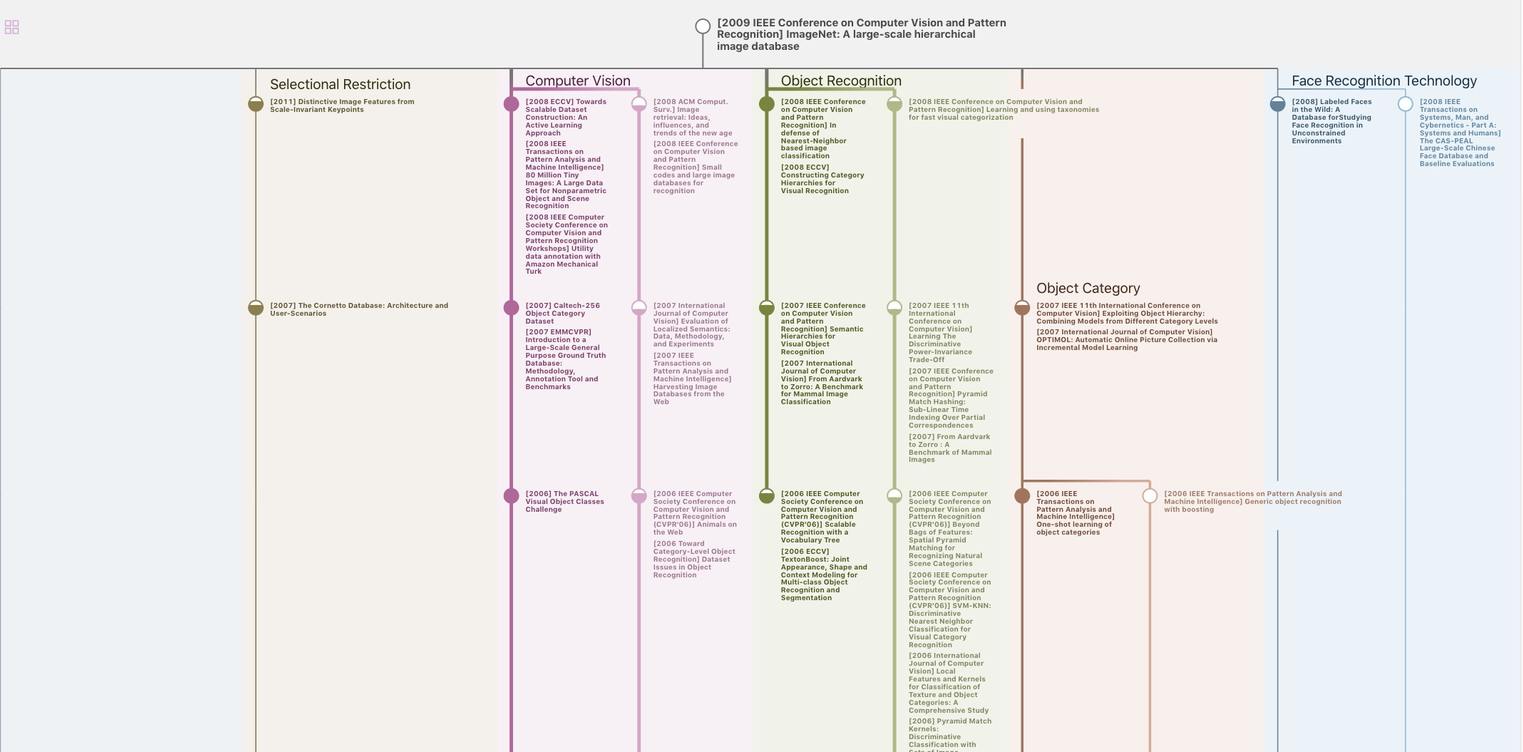
生成溯源树,研究论文发展脉络
Chat Paper
正在生成论文摘要