Automotive Security: Threat Forewarning and ECU Source Mapping Derived From Physical Features of Network Signals
IEEE TRANSACTIONS ON INTELLIGENT TRANSPORTATION SYSTEMS(2023)
摘要
Intelligent Connected Vehicles (ICVs), have developed rapidly towards information networking, and they are destined to become an important carrier of future travel. However, they also face some challenges like cyber-security. Controller Area Network (CAN), as a typical communication medium of ICVs, is very vulnerable to cyber-attacks since they lack identity authentication and message encryption. Therefore, how to pinpoint the source of abnormal messages, also namely Electronic Control Unit (ECU) source mapping scheme, is of significance to the in-vehicle communication. However, most existing studies that utilize learning algorithms consider little on the stability of training features. To this end, this paper develops a hybrid-feature-extraction based ECU mapping scheme derived from physical features of network signals. In detail, more stable bit-block groups and mode value of high-level voltages are extracted as training features. On this basis, single-layer Softmax classifier is formulated to accurately identify ECU sources. Finally, the proposed scheme is experimentally validated with eight ECUs in a real-world vehicle. Results show that the average identification accuracy of all ECUs is close to 98%, which has been improved by more than 6% compared with other typical methods. The present study effectively addresses the deficiency of identity authentication in CAN.
更多查看译文
关键词
Intelligent connected vehicle (ICV),electric control unit (ECU),threat forewarning,bit-block groups,feature extraction
AI 理解论文
溯源树
样例
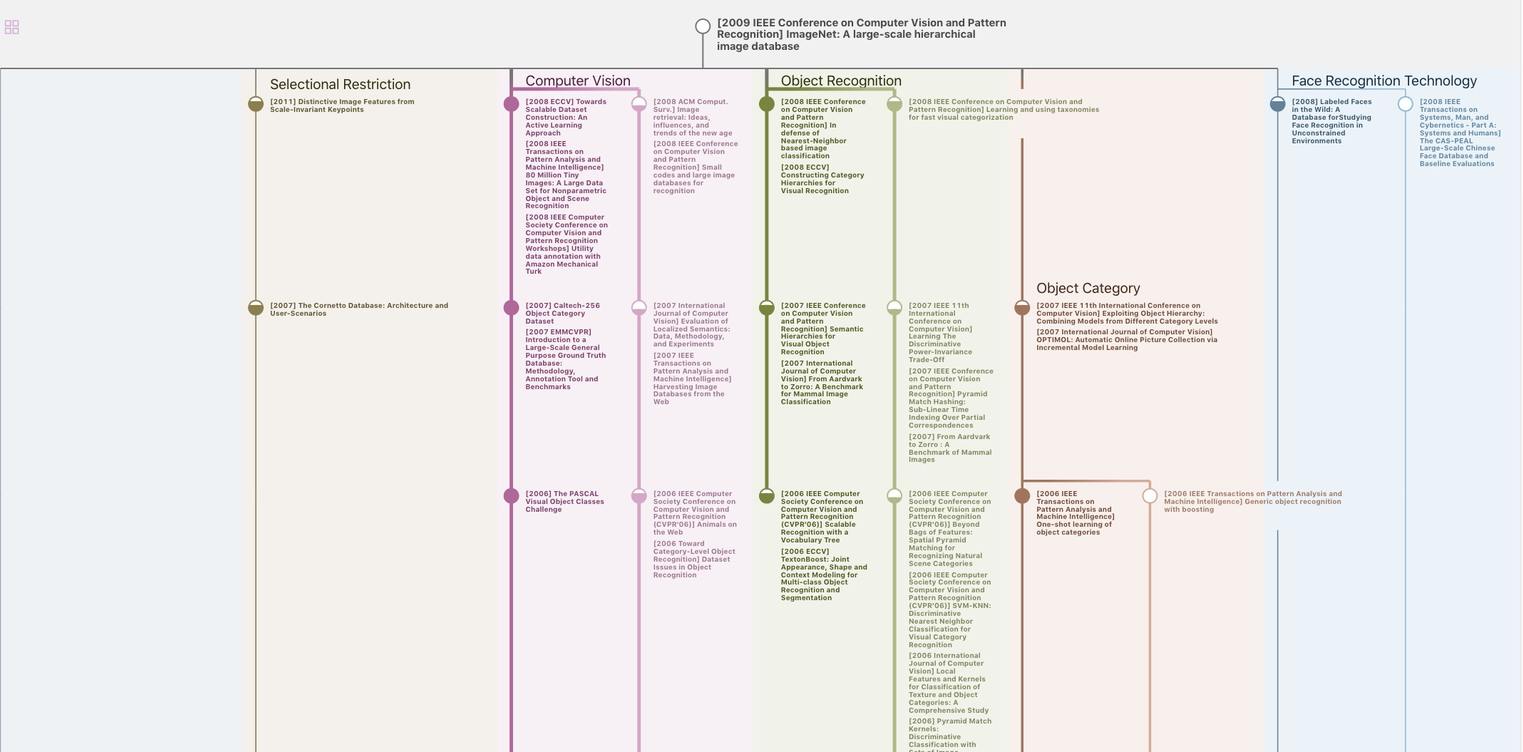
生成溯源树,研究论文发展脉络
Chat Paper
正在生成论文摘要