Partial Alignment of Time Series for Action and Activity Prediction
Communications in computer and information science(2023)
摘要
The temporal alignment of two complete action/activity sequences has been the focus of interest in many research works. However, the problem of partially aligning an incomplete sequence to a complete one has not been sufficiently explored. Very effective alignment algorithms such as Dynamic Time Warping (DTW) and Soft Dynamic Time Warping (S-DTW) are not capable of handling incomplete sequences. To overcome this limitation the Open-End DTW (OE-DTW) and the Open-Begin-End DTW (OBE-DTW) algorithms were introduced. The OE-DTW has the capability to align sequences with common begin points but unknown ending points, while the OBE-DTW has the ability to align unsegmented sequences. We focus on two new alignment algorithms, namely the Open-End Soft DTW (OE-S-DTW) and the Open-Begin-End Soft DTW (OBE-S-DTW) which combine the partial alignment capabilities of OE-DTW and OBE-DTW with those of Soft DTW (S-DTW). Specifically, these algorithms have the segregational capabilities of DTW combined with the soft-minimum operator of the S-DTW algorithm that results in improved, differentiable alignment in the case of continuous, unsegmented actions/activities. The developed algorithms are well-suited tools for addressing the problem of action prediction. By properly matching and aligning an on-going, incomplete action/activity sequence to prototype, complete ones, we may gain insight in what comes next in the on-going action/activity. The proposed algorithms are evaluated on the MHAD, MHAD101-v/-s, MSR Daily Activities and CAD-120 datasets and are shown to outperform relevant state of the art approaches.
更多查看译文
关键词
activity prediction,partial alignment,time series
AI 理解论文
溯源树
样例
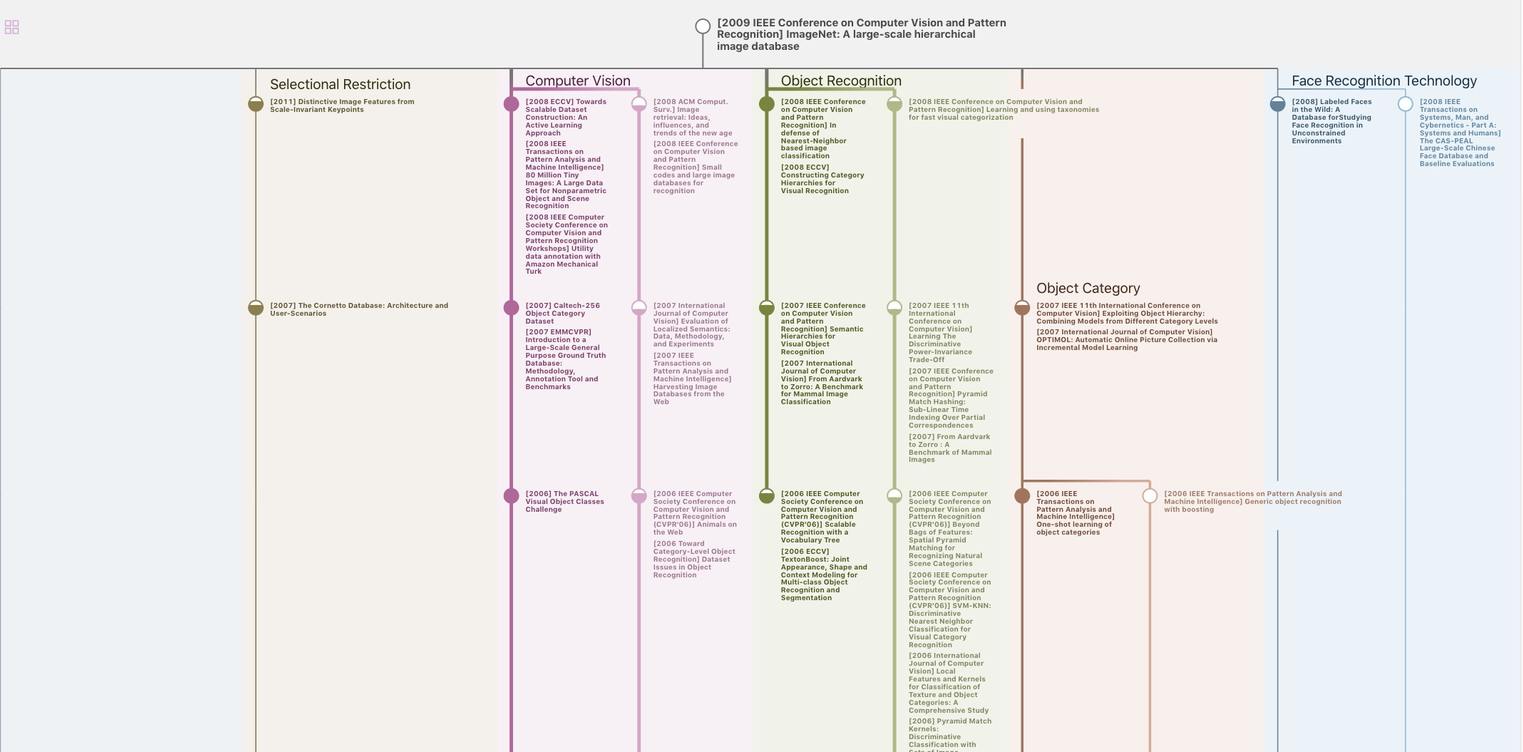
生成溯源树,研究论文发展脉络
Chat Paper
正在生成论文摘要