Federated Learning Enabled Link Scheduling in D2D Wireless Networks
IEEE WIRELESS COMMUNICATIONS LETTERS(2024)
摘要
Centralized machine learning methods for device-to-device (D2D) link scheduling may lead to a computing burden for a central server, transmission latency for decisions, and privacy issues for D2D communications. To mitigate these challenges, a federated learning (FL) based method is proposed to solve the link scheduling problem, where a global model is distributedly trained at local devices, and a server is used for aggregating model parameters instead of training samples. Specially, a more realistic scenario with limited channel state information (CSI) is considered instead of full CSI. Despite a decentralized implementation, simulation results demonstrate that the proposed FL based approach with limited CSI performs close to the conventional optimization algorithm. In addition, the FL based solution achieves almost the same performance as that of the centralized training.
更多查看译文
关键词
Federated learning,device-to-device (D2D),link scheduling
AI 理解论文
溯源树
样例
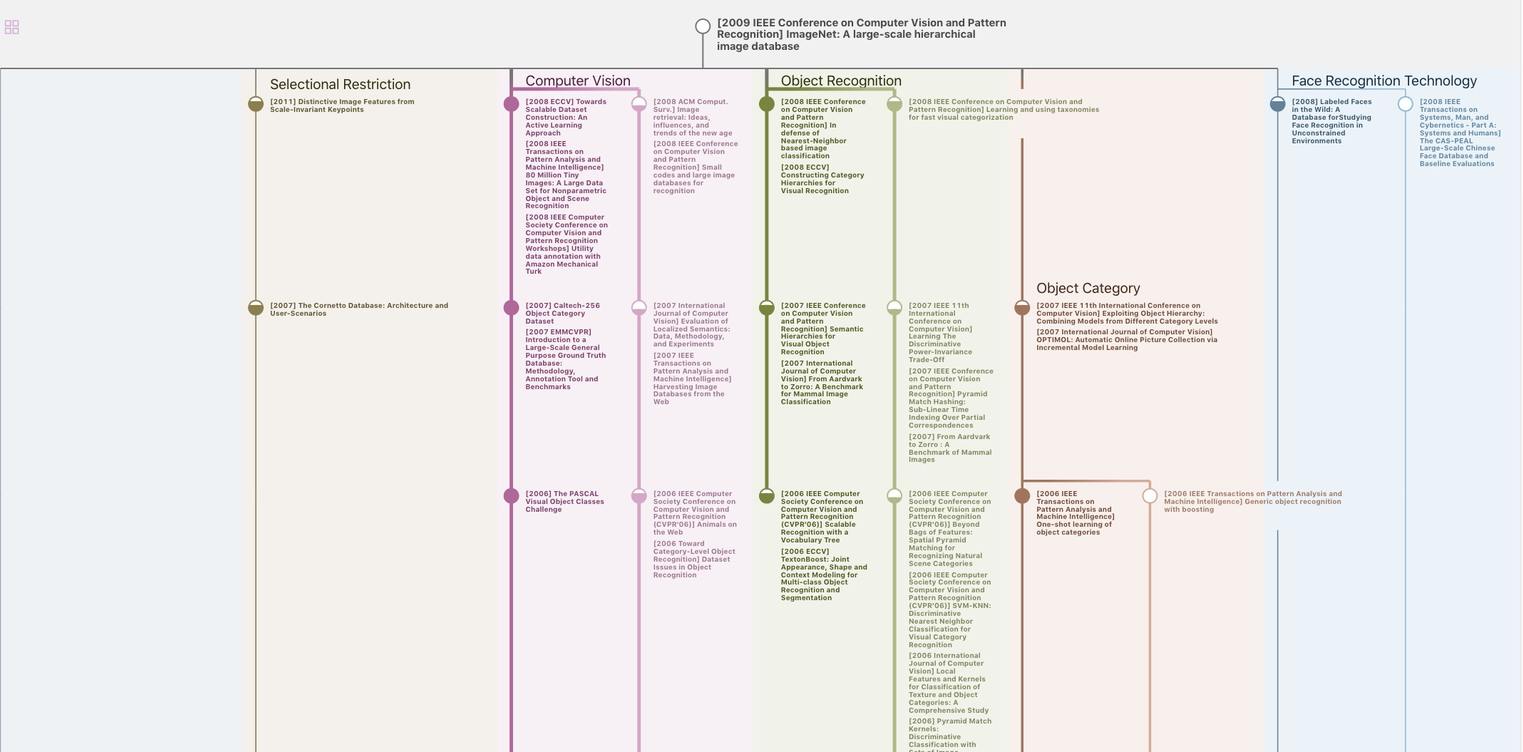
生成溯源树,研究论文发展脉络
Chat Paper
正在生成论文摘要