NeuralODE-Based Latent Trajectories into AutoEncoder Architecture for Surrogate Modelling of Parametrized High-Dimensional Dynamical Systems
ARTIFICIAL NEURAL NETWORKS AND MACHINE LEARNING, ICANN 2023, PT VI(2023)
摘要
Data-driven modelling has recently gained interest in the scientific computing community with the purpose of emulating complex large scale systems. Surrogate modelling based on autoencoders (AEs) is widely employed across several engineering fields to model the time-history response of nonlinear high-dimensional dynamical systems from a set of design parameters. In this direction, this paper introduces an efficient deep learning scheme consisting of a two-steps autoencoding framework in conjunction with Neural Ordinary Differential Equations (NODEs), a novel approach for modelling time-continuous dynamics. The proposition aims at alleviating the drawbacks of similar methodologies employed for the same task, namely Parametrized NODE (PNODE) and the two-steps AE-based surrogate models, to provide a more powerful predictive tool. The effectiveness of the conceived methodology has been assessed by considering the task of emulating the spatiotemporal dynamics described by the 1D viscous Burgers' equation. The outcomes of our empirical analysis demonstrate that our approach outperforms the alternative state-of-the-art models in terms of predictive capability.
更多查看译文
关键词
Surrogate modelling,Deep Learning,NODE,Dynamical Systems
AI 理解论文
溯源树
样例
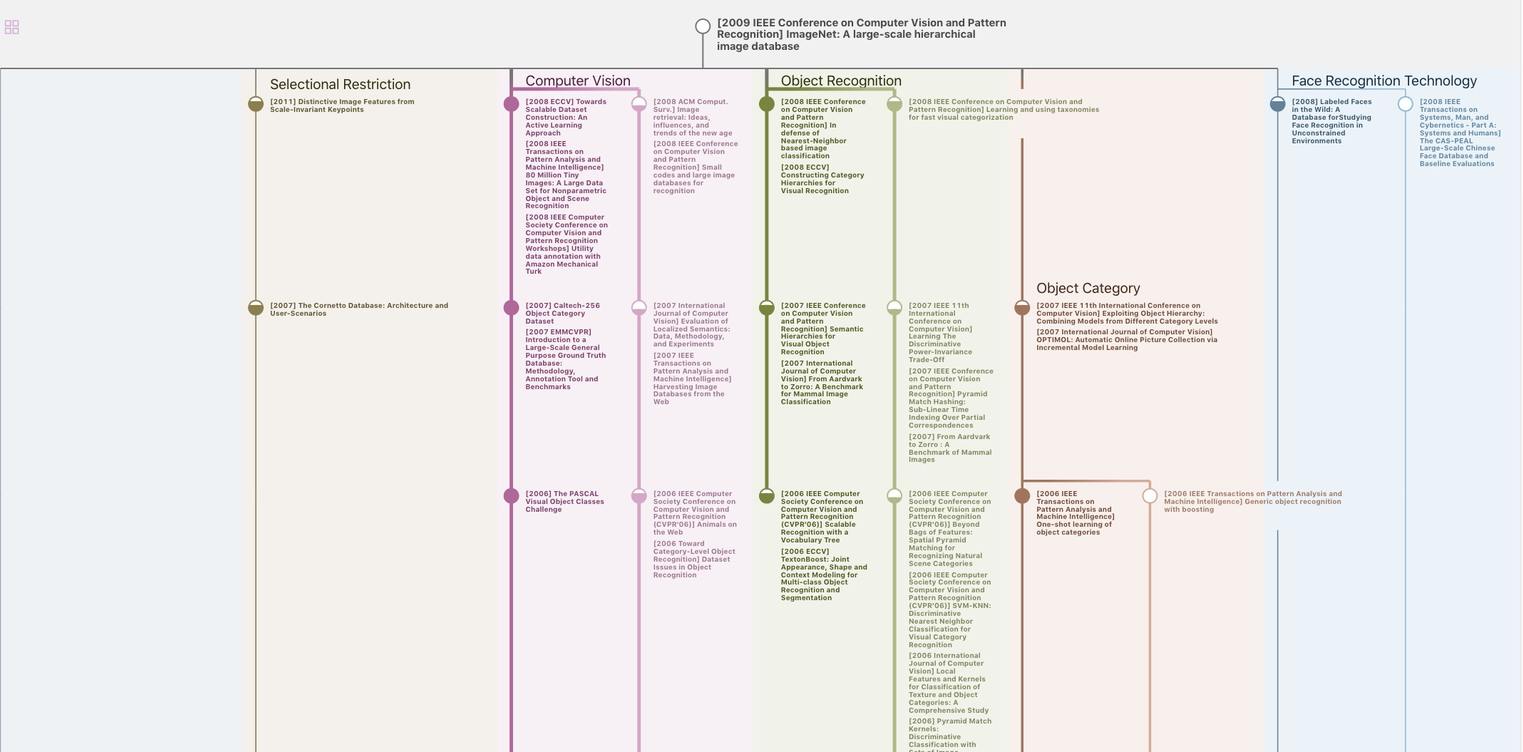
生成溯源树,研究论文发展脉络
Chat Paper
正在生成论文摘要