Vibration-based building health monitoring using spatio-temporal learning model
ENGINEERING APPLICATIONS OF ARTIFICIAL INTELLIGENCE(2023)
摘要
Vibration-based building health monitoring is a promising and feasible approach to assess the operational state of building structures in a remote, automated, and continuous fashion; however, efficiently handling high-dimensional vibration signals from multiple sensors and effectively coping with missing/noisy data represent two main technical challenges. In order to overcome these issues, this study proposes a novel, reliable and robust framework, abbreviated CLG-BHM, based on a hybrid deep learning architecture. First, the framework uses a 1D convolutional neural network layer to learn low-dimensional representation vectors of long sensor signals, which preserve underlying structures' dynamic characteristics. Second, temporal relationships within data are distilled via a Long-Short Term Memory layer. Third, the representation vectors of sensors are aggregated with those of their neighbors in a principled way via a graph attention network layer, resulting in a new latent representation rich in both temporal and spatial information. Finally, the latter is gone through a fully-connected layer to provide damage detection results. The performance and viability of the present method are evidenced via various examples involving a simple lumped mass structure, a semi-rigid steel frame, and an experimental 4-story structure from the literature. Moreover, a robustness study is performed, showing that the method can provide reasonable results with the presence of noisy and missing data.
更多查看译文
关键词
Building health monitoring,Deep learning,Vibration,Structural analysis,Numerical simulation
AI 理解论文
溯源树
样例
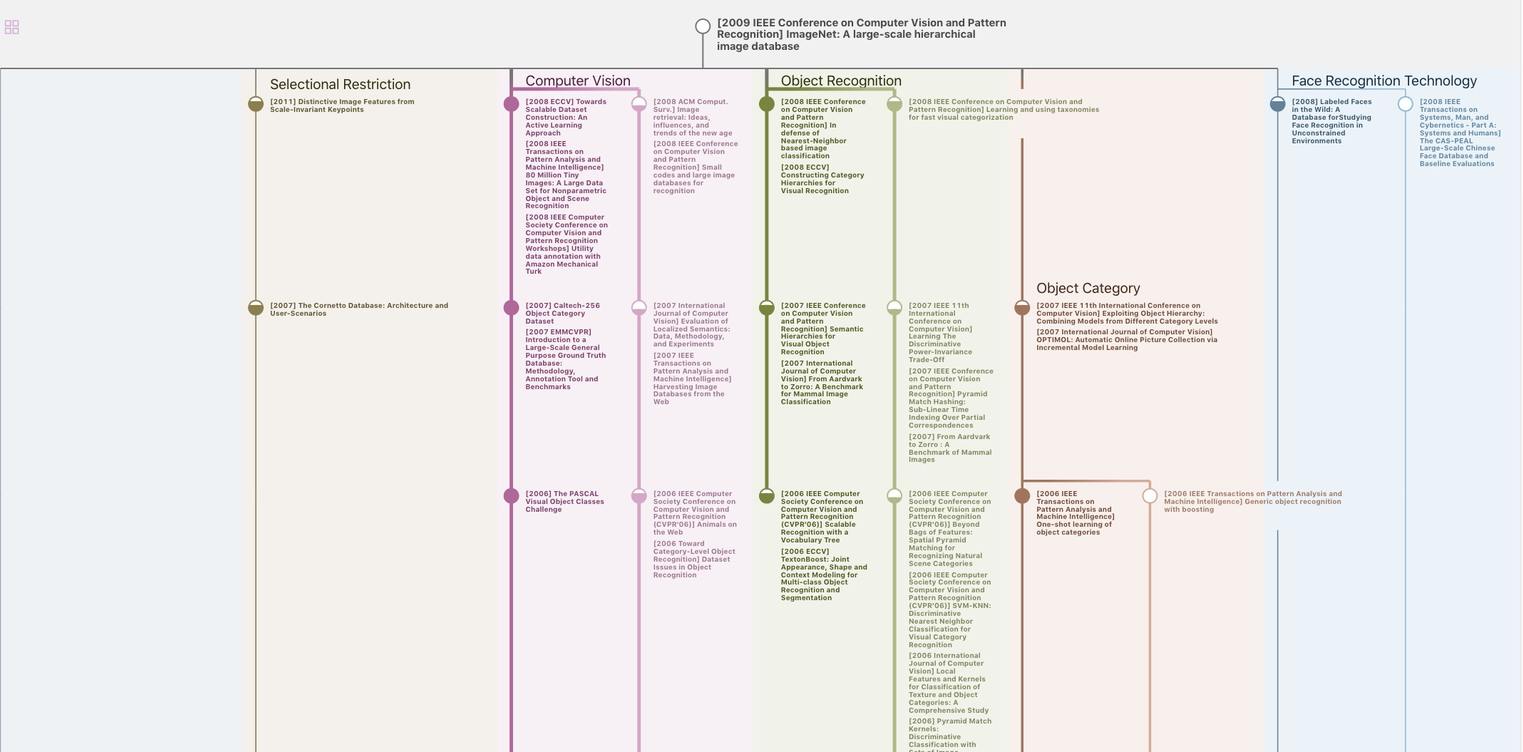
生成溯源树,研究论文发展脉络
Chat Paper
正在生成论文摘要