Comparison between Machine Learning and Bivariate Statistical Models for Groundwater Recharge Zones
Research Square (Research Square)(2023)
摘要
Abstract Due to population growth and climate change, dependence on groundwater is expected to increase. This growth has put forth a major challenge of management for sustainable groundwater storage. This study illustrates a newly introduced bivariate statistical model with an ensembled data mining approach. Certainty factor (CF), evidential belief function (EBF), frequency ratio (FR) and convolutional neural network (CNN) are four bivariant statistical models. These four models are integrated with the logistic model tree (LMT) and random forest (RF). These models are used for preparing the groundwater potential map (GPM). The receiver operating characteristic (ROC) curve and area under the curve (AUC) were utilized for calculating the accuracy of the groundwater potential maps. The sequence and values of AUC obtained from the results are as CNN-RF (0.923), CF-RF (0.914), EBF-RF (0.911), FR-RF (0.904), CF-LMT (0.893), EBF-LMT (0.872) and FR-LMT (0.817). It can be concluded that the combination of bivariate statistic models and data mining techniques advance the method’s efficiency in creating a potential mapping of groundwater.
更多查看译文
关键词
groundwater recharge zones,bivariate statistical models,machine learning
AI 理解论文
溯源树
样例
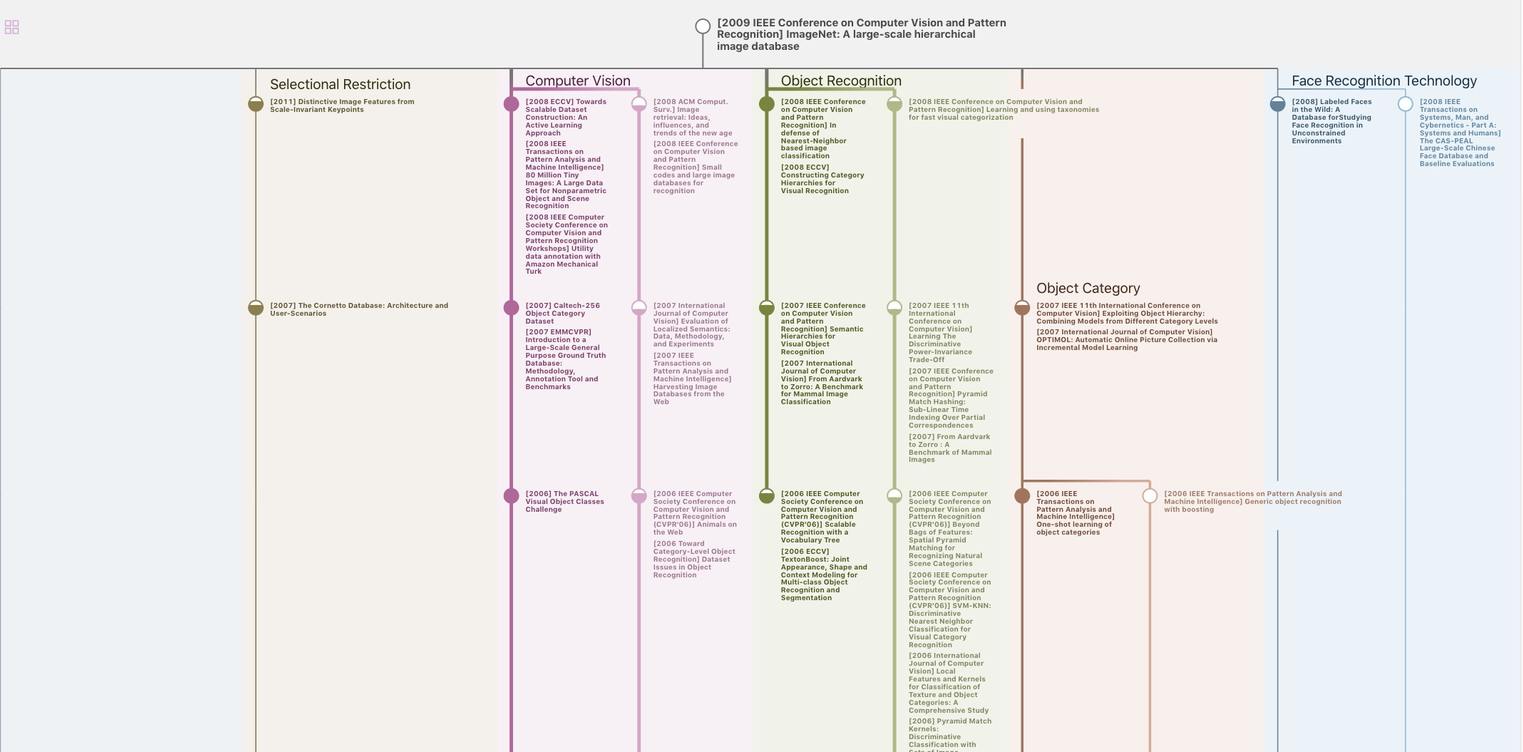
生成溯源树,研究论文发展脉络
Chat Paper
正在生成论文摘要