Resolving Zero-Shot and Fact-Based Visual Question Answering via Enhanced Fact Retrieval
IEEE TRANSACTIONS ON MULTIMEDIA(2024)
摘要
Practical applications with visual question answering (VQA) systems are challenging, and recent research has aimed at investigating this important field. Many issues related to real-world VQA applications must be considered. Although existing methods have focused on adding external knowledge and other descriptive information to assist in reasoning, they are limited by the impact of information retrieval errors on downstream tasks and the misalignment of the aggregated information. Thus, the overall performance of these models must be improved. To address these challenges, we propose a novel VQA model that utilizes a differentiated pretrained model to represent the input information and connects the input data with three external knowledge components through a common feature space. To combine the information in the three feature spaces, we propose an information aggregation strategy that employs a weighted score to aggregate the information in the relation and entity spaces in the answer prediction process. The experimental results show that our method achieves good performance in fact-based and zero-shot VQA tasks and achieves state-of-the-art performance with the ZS-F-VQA dataset.
更多查看译文
关键词
Visualization,Task analysis,Knowledge based systems,Question answering (information retrieval),Predictive models,Knowledge graphs,Feature extraction,Visual question answering,zero-shot,knowledge graph
AI 理解论文
溯源树
样例
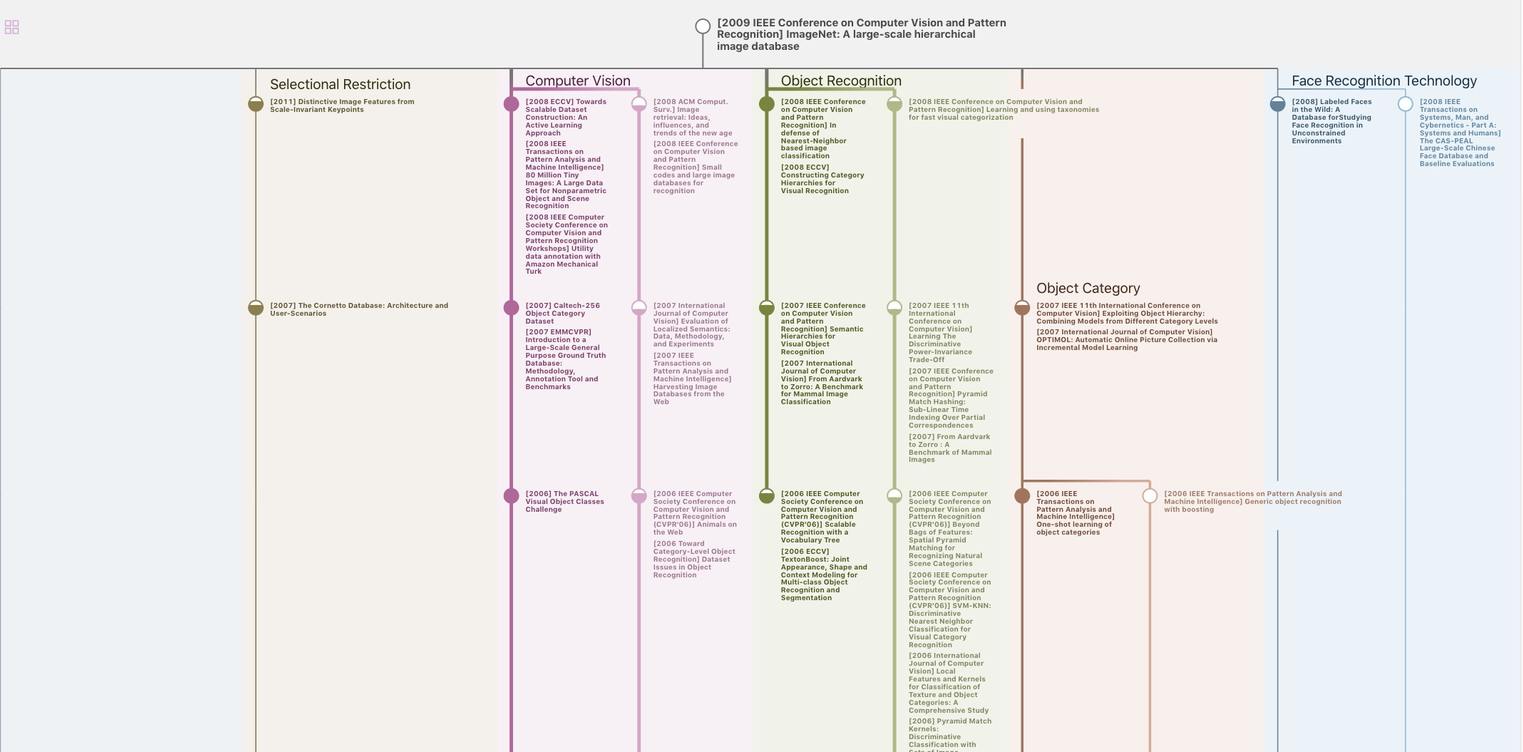
生成溯源树,研究论文发展脉络
Chat Paper
正在生成论文摘要