A kernel Stein test for comparing latent variable models
Journal of the Royal Statistical Society Series B: Statistical Methodology(2023)
摘要
Abstract We propose a kernel-based nonparametric test of relative goodness of fit, where the goal is to compare two models, both of which may have unobserved latent variables, such that the marginal distribution of the observed variables is intractable. The proposed test generalizes the recently proposed kernel Stein discrepancy (KSD) tests (Liu et al., Proceedings of the 33rd international conference on machine learning (pp. 276–284); Chwialkowski et al., (2016), In Proceedings of the 33rd international conference on machine learning (pp. 2606–2615); Yang et al., (2018), In Proceedings of the 35th international conference on machine learning (pp. 5561–5570)) to the case of latent variable models, a much more general class than the fully observed models treated previously. The new test, with a properly calibrated threshold, has a well-controlled type-I error. In the case of certain models with low-dimensional latent structures and high-dimensional observations, our test significantly outperforms the relative maximum mean discrepancy test, which is based on samples from the models and does not exploit the latent structure.
更多查看译文
关键词
kernel stein test,models
AI 理解论文
溯源树
样例
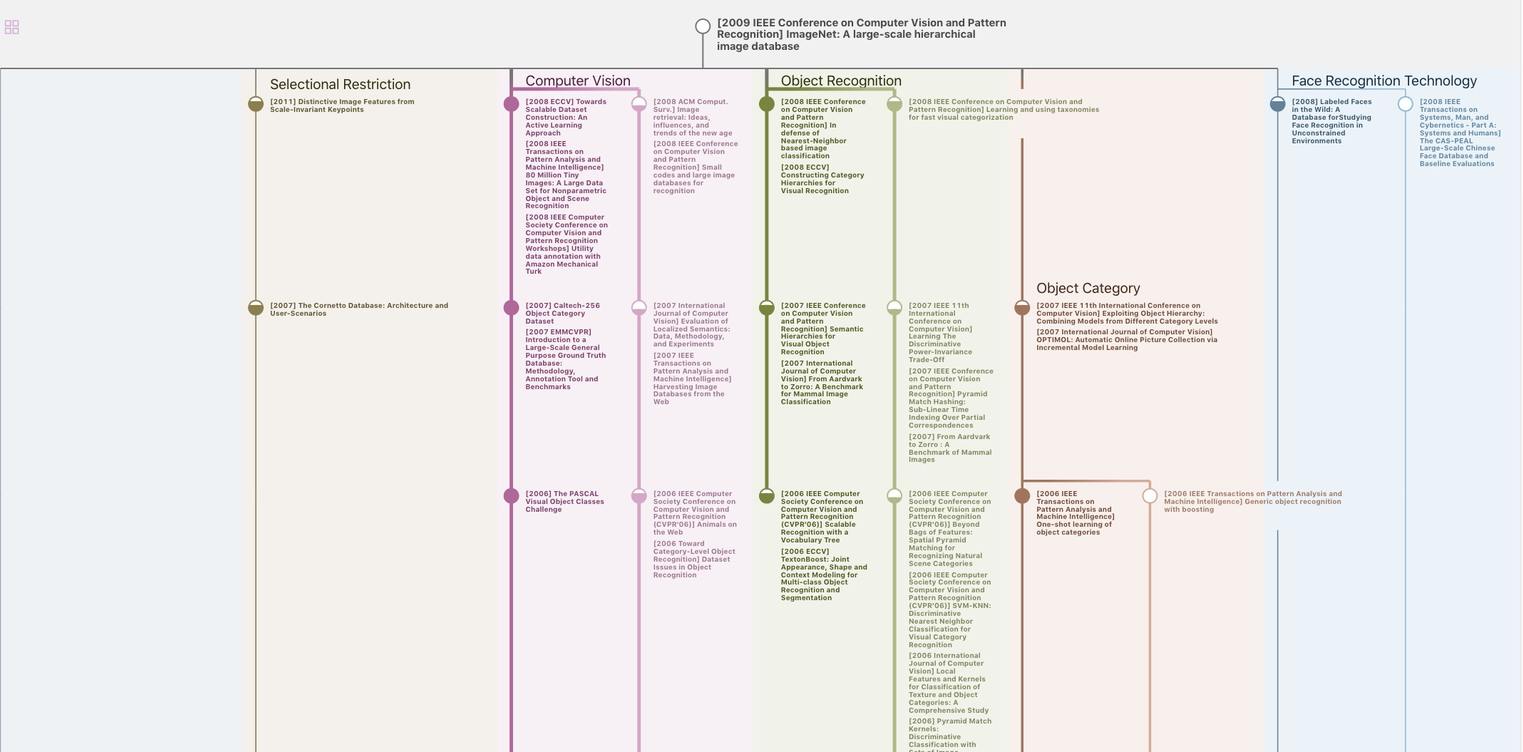
生成溯源树,研究论文发展脉络
Chat Paper
正在生成论文摘要